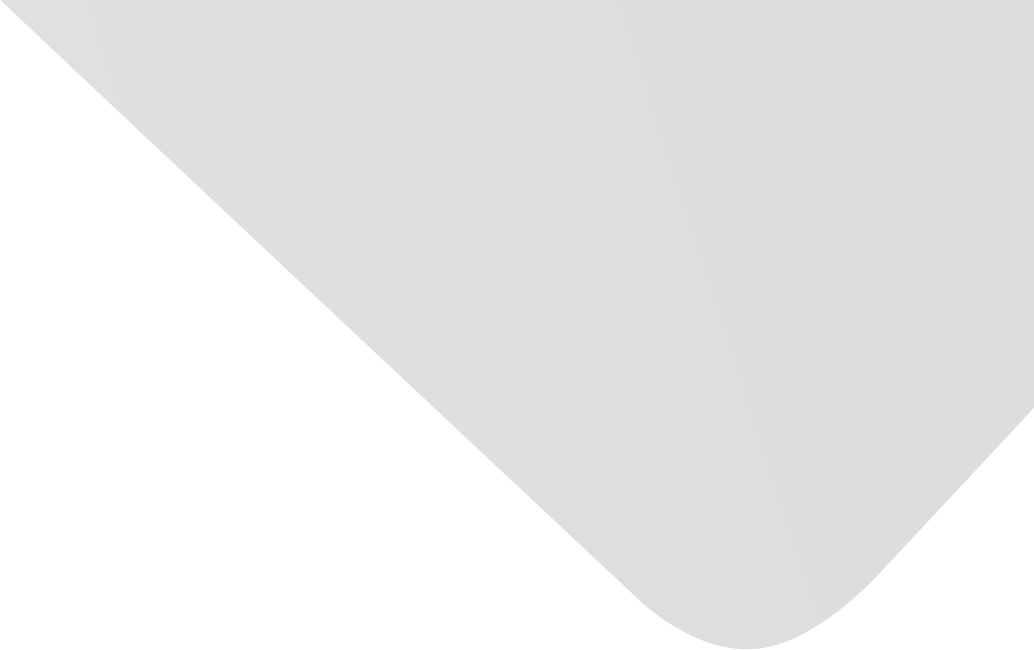
Similarity Measure Learning in Closed-Form Solution for Image Classification
Joint Authors
Chen, Jing
Chen, C. L. Philip
Lin, Yuewei
Zhaowei, Shang
Fang, Bin
Tang, Yuan Yan
Source
Issue
Vol. 2014, Issue 2014 (31 Dec. 2014), pp.1-15, 15 p.
Publisher
Hindawi Publishing Corporation
Publication Date
2014-06-26
Country of Publication
Egypt
No. of Pages
15
Main Subjects
Medicine
Information Technology and Computer Science
Abstract EN
Adopting a measure is essential in many multimedia applications.
Recently, distance learning is becoming an active research problem.
In fact, the distance is the natural measure for dissimilarity.
Generally, a pairwise relationship between two objects in learning tasks includes two aspects: similarity and dissimilarity.
The similarity measure provides different information for pairwise relationships.
However, similarity learning has been paid less attention in learning problems.
In this work, firstly, we propose a general framework for similarity measure learning (SML).
Additionally, we define a generalized type of correlation as a similarity measure.
By a set of parameters, generalized correlation provides flexibility for learning tasks.
Based on this similarity measure, we present a specific algorithm under the SML framework, called correlation similarity measure learning (CSML), to learn a parameterized similarity measure over input space.
A nonlinear extension version of CSML, kernel CSML, is also proposed.
Particularly, we give a closed-form solution avoiding iterative search for a local optimal solution in the high-dimensional space as the previous work did.
Finally, classification experiments have been performed on face databases and a handwritten digits database to demonstrate the efficiency and reliability of CSML and KCSML.
American Psychological Association (APA)
Chen, Jing& Tang, Yuan Yan& Chen, C. L. Philip& Fang, Bin& Zhaowei, Shang& Lin, Yuewei. 2014. Similarity Measure Learning in Closed-Form Solution for Image Classification. The Scientific World Journal،Vol. 2014, no. 2014, pp.1-15.
https://search.emarefa.net/detail/BIM-1050884
Modern Language Association (MLA)
Chen, Jing…[et al.]. Similarity Measure Learning in Closed-Form Solution for Image Classification. The Scientific World Journal No. 2014 (2014), pp.1-15.
https://search.emarefa.net/detail/BIM-1050884
American Medical Association (AMA)
Chen, Jing& Tang, Yuan Yan& Chen, C. L. Philip& Fang, Bin& Zhaowei, Shang& Lin, Yuewei. Similarity Measure Learning in Closed-Form Solution for Image Classification. The Scientific World Journal. 2014. Vol. 2014, no. 2014, pp.1-15.
https://search.emarefa.net/detail/BIM-1050884
Data Type
Journal Articles
Language
English
Notes
Includes bibliographical references
Record ID
BIM-1050884