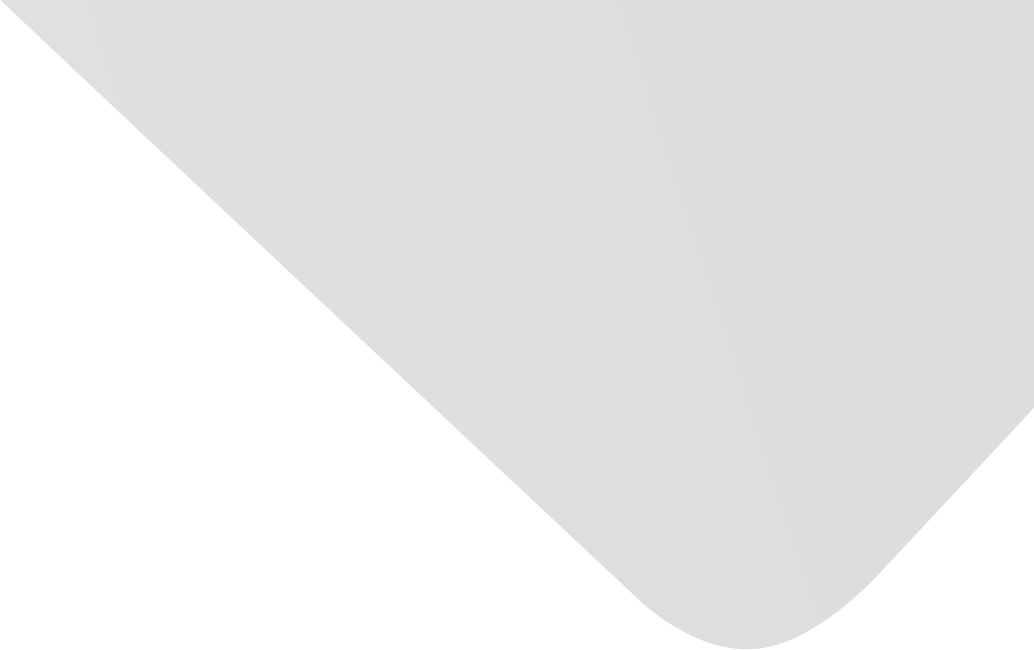
Network Anomaly Detection System with Optimized DS Evidence Theory
Joint Authors
Liu, Yuan
Wang, Xiaofeng
Liu, Kaiyu
Source
Issue
Vol. 2014, Issue 2014 (31 Dec. 2014), pp.1-13, 13 p.
Publisher
Hindawi Publishing Corporation
Publication Date
2014-08-31
Country of Publication
Egypt
No. of Pages
13
Main Subjects
Medicine
Information Technology and Computer Science
Abstract EN
Network anomaly detection has been focused on by more people with the fast development of computer network.
Some researchers utilized fusion method and DS evidence theory to do network anomaly detection but with low performance, and they did not consider features of network—complicated and varied.
To achieve high detection rate, we present a novel network anomaly detection system with optimized Dempster-Shafer evidence theory (ODS) and regression basic probability assignment (RBPA) function.
In this model, we add weights for each senor to optimize DS evidence theory according to its previous predict accuracy.
And RBPA employs sensor’s regression ability to address complex network.
By four kinds of experiments, we find that our novel network anomaly detection model has a better detection rate, and RBPA as well as ODS optimization methods can improve system performance significantly.
American Psychological Association (APA)
Liu, Yuan& Wang, Xiaofeng& Liu, Kaiyu. 2014. Network Anomaly Detection System with Optimized DS Evidence Theory. The Scientific World Journal،Vol. 2014, no. 2014, pp.1-13.
https://search.emarefa.net/detail/BIM-1050920
Modern Language Association (MLA)
Liu, Yuan…[et al.]. Network Anomaly Detection System with Optimized DS Evidence Theory. The Scientific World Journal No. 2014 (2014), pp.1-13.
https://search.emarefa.net/detail/BIM-1050920
American Medical Association (AMA)
Liu, Yuan& Wang, Xiaofeng& Liu, Kaiyu. Network Anomaly Detection System with Optimized DS Evidence Theory. The Scientific World Journal. 2014. Vol. 2014, no. 2014, pp.1-13.
https://search.emarefa.net/detail/BIM-1050920
Data Type
Journal Articles
Language
English
Notes
Includes bibliographical references
Record ID
BIM-1050920