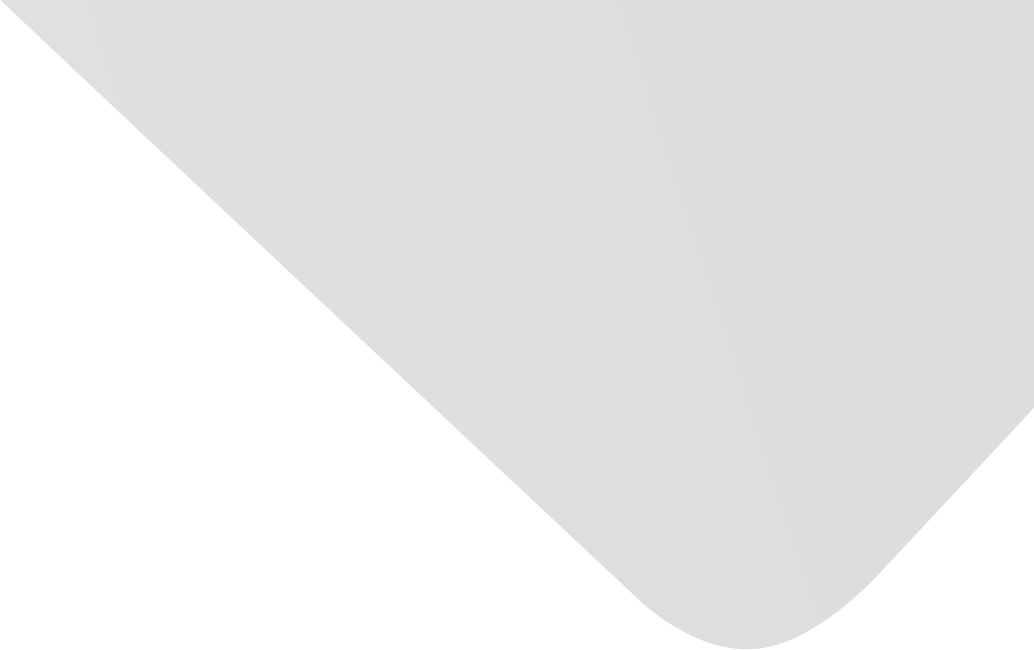
Simultaneous Channel and Feature Selection of Fused EEG Features Based on Sparse Group Lasso
Joint Authors
Source
Issue
Vol. 2015, Issue 2015 (31 Dec. 2015), pp.1-13, 13 p.
Publisher
Hindawi Publishing Corporation
Publication Date
2015-02-24
Country of Publication
Egypt
No. of Pages
13
Main Subjects
Abstract EN
Feature extraction and classification of EEG signals are core parts of brain computer interfaces (BCIs).
Due to the high dimension of the EEG feature vector, an effective feature selection algorithm has become an integral part of research studies.
In this paper, we present a new method based on a wrapped Sparse Group Lasso for channel and feature selection of fused EEG signals.
The high-dimensional fused features are firstly obtained, which include the power spectrum, time-domain statistics, AR model, and the wavelet coefficient features extracted from the preprocessed EEG signals.
The wrapped channel and feature selection method is then applied, which uses the logistical regression model with Sparse Group Lasso penalized function.
The model is fitted on the training data, and parameter estimation is obtained by modified blockwise coordinate descent and coordinate gradient descent method.
The best parameters and feature subset are selected by using a 10-fold cross-validation.
Finally, the test data is classified using the trained model.
Compared with existing channel and feature selection methods, results show that the proposed method is more suitable, more stable, and faster for high-dimensional feature fusion.
It can simultaneously achieve channel and feature selection with a lower error rate.
The test accuracy on the data used from international BCI Competition IV reached 84.72%.
American Psychological Association (APA)
Wang, Jin-Jia& Xue, Fang& Li, Hui. 2015. Simultaneous Channel and Feature Selection of Fused EEG Features Based on Sparse Group Lasso. BioMed Research International،Vol. 2015, no. 2015, pp.1-13.
https://search.emarefa.net/detail/BIM-1056433
Modern Language Association (MLA)
Wang, Jin-Jia…[et al.]. Simultaneous Channel and Feature Selection of Fused EEG Features Based on Sparse Group Lasso. BioMed Research International No. 2015 (2015), pp.1-13.
https://search.emarefa.net/detail/BIM-1056433
American Medical Association (AMA)
Wang, Jin-Jia& Xue, Fang& Li, Hui. Simultaneous Channel and Feature Selection of Fused EEG Features Based on Sparse Group Lasso. BioMed Research International. 2015. Vol. 2015, no. 2015, pp.1-13.
https://search.emarefa.net/detail/BIM-1056433
Data Type
Journal Articles
Language
English
Notes
Includes bibliographical references
Record ID
BIM-1056433