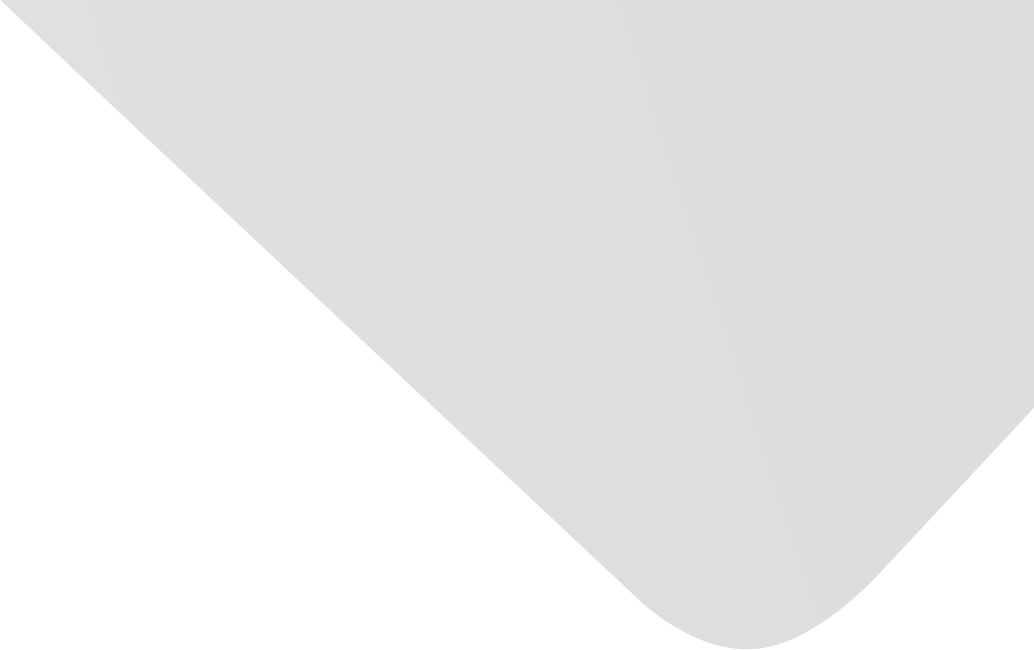
Tumour Relapse Prediction Using Multiparametric MR Data Recorded during Follow-Up of GBM Patients
Joint Authors
Van Huffel, Sabine
Van Gool, Stefaan
Ion-Margineanu, Adrian
Van Cauter, Sofie
Sima, Diana M.
Maes, Frederik
Sunaert, Stefan
Himmelreich, Uwe
Source
Issue
Vol. 2015, Issue 2015 (31 Dec. 2015), pp.1-13, 13 p.
Publisher
Hindawi Publishing Corporation
Publication Date
2015-08-27
Country of Publication
Egypt
No. of Pages
13
Main Subjects
Abstract EN
Purpose.
We have focused on finding a classifier that best discriminates between tumour progression and regression based on multiparametric MR data retrieved from follow-up GBM patients.
Materials and Methods.
Multiparametric MR data consisting of conventional and advanced MRI (perfusion, diffusion, and spectroscopy) were acquired from 29 GBM patients treated with adjuvant therapy after surgery over a period of several months.
A 27-feature vector was built for each time point, although not all features could be obtained at all time points due to missing data or quality issues.
We tested classifiers using LOPO method on complete and imputed data.
We measure the performance by computing BER for each time point and wBER for all time points.
Results.
If we train random forests, LogitBoost, or RobustBoost on data with complete features, we can differentiate between tumour progression and regression with 100% accuracy, one time point (i.e., about 1 month) earlier than the date when doctors had put a label (progressive or responsive) according to established radiological criteria.
We obtain the same result when training the same classifiers solely on complete perfusion data.
Conclusions.
Our findings suggest that ensemble classifiers (i.e., random forests and boost classifiers) show promising results in predicting tumour progression earlier than established radiological criteria and should be further investigated.
American Psychological Association (APA)
Ion-Margineanu, Adrian& Van Cauter, Sofie& Sima, Diana M.& Maes, Frederik& Van Gool, Stefaan& Sunaert, Stefan…[et al.]. 2015. Tumour Relapse Prediction Using Multiparametric MR Data Recorded during Follow-Up of GBM Patients. BioMed Research International،Vol. 2015, no. 2015, pp.1-13.
https://search.emarefa.net/detail/BIM-1056962
Modern Language Association (MLA)
Ion-Margineanu, Adrian…[et al.]. Tumour Relapse Prediction Using Multiparametric MR Data Recorded during Follow-Up of GBM Patients. BioMed Research International No. 2015 (2015), pp.1-13.
https://search.emarefa.net/detail/BIM-1056962
American Medical Association (AMA)
Ion-Margineanu, Adrian& Van Cauter, Sofie& Sima, Diana M.& Maes, Frederik& Van Gool, Stefaan& Sunaert, Stefan…[et al.]. Tumour Relapse Prediction Using Multiparametric MR Data Recorded during Follow-Up of GBM Patients. BioMed Research International. 2015. Vol. 2015, no. 2015, pp.1-13.
https://search.emarefa.net/detail/BIM-1056962
Data Type
Journal Articles
Language
English
Notes
Includes bibliographical references
Record ID
BIM-1056962