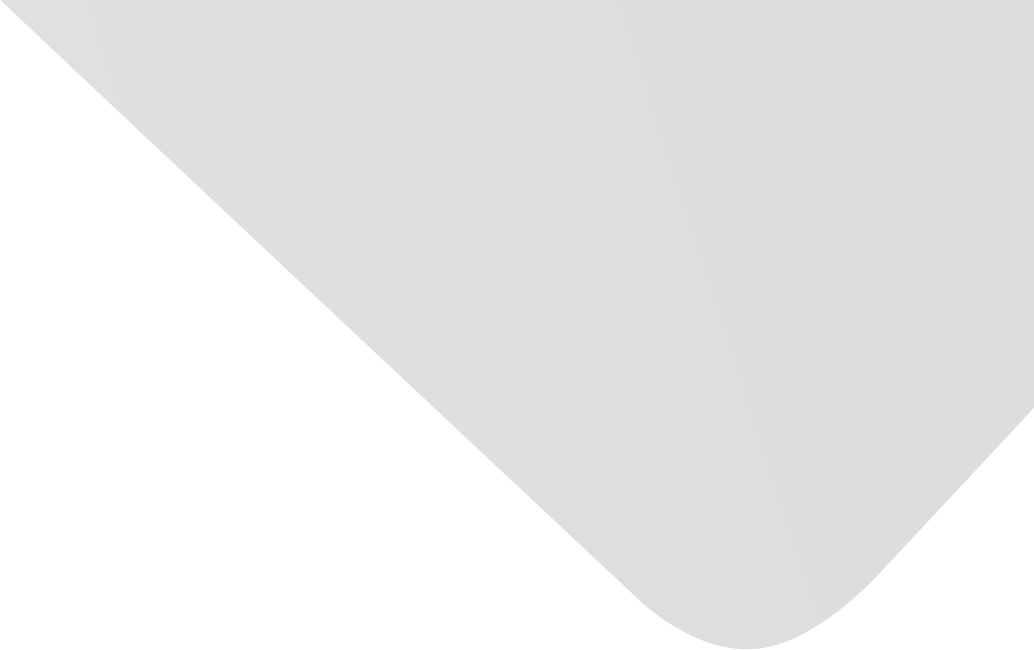
Brain machine interface : analysis of segmented eeg signal classification using short-time pca and recurrent neural networks
Other Title(s)
دائرة موائمه بين الدماغ و لآله : تحليل تصنيف إشارة الدماغ المقطعه باستخدام طريقة تحليل المكون الرئيس بالزمن القصير و دوائر الشبكات العصبية المرتدة
Joint Authors
Paulraj, M. P.
Hema, C. R.
Nagarajan, R.
Yaqub, Sazali
Adam, Abd al-Hamid
Source
The Iraqi Journal of Electrical and Electronic Engineering
Issue
Vol. 4, Issue 1 (31 Jan. 2008), pp.77-85, 9 p.
Publisher
University of Basrah College of Engineering
Publication Date
2008-01-31
Country of Publication
Iraq
No. of Pages
9
Main Subjects
Information Technology and Computer Science
Topics
Abstract AR
تقوم دوائر الموائمة بين الإنسان و الآلة بعملية الاتصال بين الدماغ البشري و الآلة و الأجهزة الخارجية.
تكمن أهمية دراسة هذه الدوائر في علاج المرضى الذین یعانون من أمراض الانحلال العصبي مثل فقدان طرق الاتصال العصبي عدى عملية الإحساس.
أحدى طرق العلاج لهؤلاء المرضى هو أیجاد طرق اتصال بدیله عن طریق دوائر الموائمة بين الدماغ و لآله.
تتعرف هذه الدوائر على أشارات الدماغ عن طریق الكترودات EEG و يتم الكشف عن مهام الدماغ عن طریق تصنيف الإشارة الدماغية.
یتناول هذا البحث ببناء دوائر موائمه للكشف عن خمس عمليات من موضعين.
و یستخدم شبكات عصبيه مرتدة نوع آن ألمن لغرض التصنيف.
تمت دراسة طریقتين لتقسيم الإشارة أحداها متراكبة و الأخرى غير متراكبة.
و وجد إن تقسيم الإشارة المتراكب یولد معدل تصنيف أعلى و ضمن مدیات 78.5 % إلى 100 % أما التقسيم غير المتراكب فأنه یولد تصنيف أفضل من حيث أعلى تصنيف.
Abstract EN
Brain machine interface provides a communication channel between the human brain and an external device.
Brain interfaces are studied to provide rehabilitation to patients with neurodegenerative diseases ; such patients loose all communication pathways except for their sensory and cognitive functions.
One of the possible rehabilitation methods for these patients is to provide a brain machine interface (BMI) for communication ; the BMI uses the electrical activity of the brain detected by scalp EEG electrodes.
Classification of EEG signals extracted during mental tasks is a technique for designing a BMI.
In this paper a BMI design using five mental tasks from two subjects were studied, a combination of two tasks is studied per subject.
An Elman recurrent neural network is proposed for classification of EEG signals.
Two feature extraction algorithms using overlapped and non overlapped signal segments are analyzed.
Principal component analysis is used for extracting features from the EEG signal segments.
Classification performance of overlapping EEG signal segments is observed to be better in terms of average classification with a range of 78.5 % to 100 %, while the non overlapping EEG signal segments show better classification in terms of maximum classifications.
American Psychological Association (APA)
Hema, C. R.& Paulraj, M. P.& Nagarajan, R.& Yaqub, Sazali& Adam, Abd al-Hamid. 2008. Brain machine interface : analysis of segmented eeg signal classification using short-time pca and recurrent neural networks. The Iraqi Journal of Electrical and Electronic Engineering،Vol. 4, no. 1, pp.77-85.
https://search.emarefa.net/detail/BIM-105703
Modern Language Association (MLA)
Adam, Abd al-Hamid…[et al.]. Brain machine interface : analysis of segmented eeg signal classification using short-time pca and recurrent neural networks. The Iraqi Journal of Electrical and Electronic Engineering Vol. 4, no. 1 (2008), pp.77-85.
https://search.emarefa.net/detail/BIM-105703
American Medical Association (AMA)
Hema, C. R.& Paulraj, M. P.& Nagarajan, R.& Yaqub, Sazali& Adam, Abd al-Hamid. Brain machine interface : analysis of segmented eeg signal classification using short-time pca and recurrent neural networks. The Iraqi Journal of Electrical and Electronic Engineering. 2008. Vol. 4, no. 1, pp.77-85.
https://search.emarefa.net/detail/BIM-105703
Data Type
Journal Articles
Language
English
Notes
Include bibliographical references : pp. 82-83
Record ID
BIM-105703