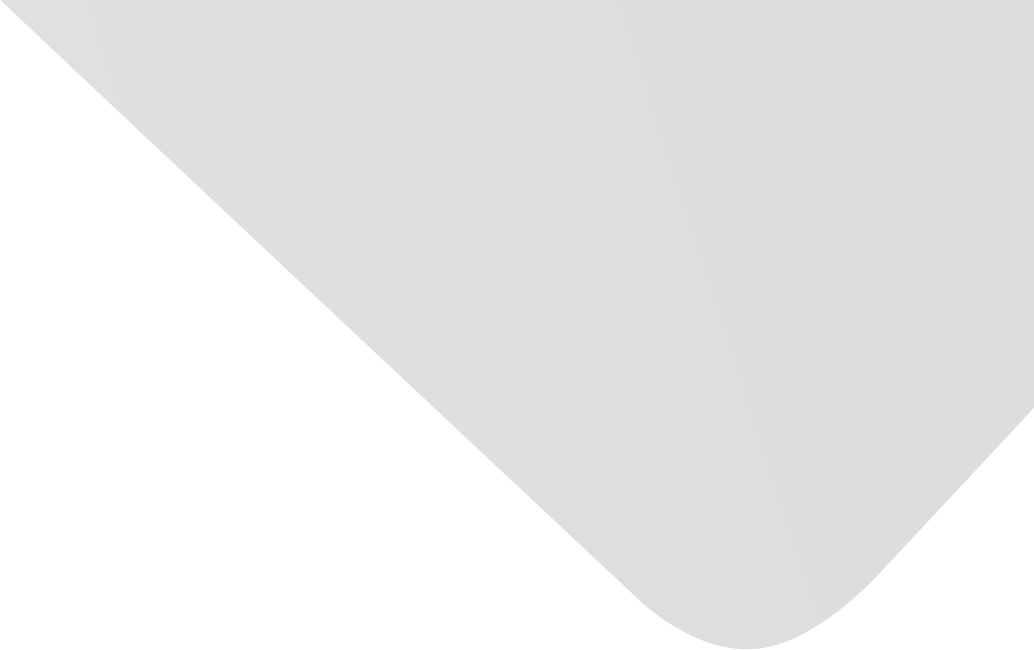
A Linear-RBF Multikernel SVM to Classify Big Text Corpora
Joint Authors
Iglesias, E. L.
Borrajo, L.
Romero, R.
Source
Issue
Vol. 2015, Issue 2015 (31 Dec. 2015), pp.1-14, 14 p.
Publisher
Hindawi Publishing Corporation
Publication Date
2015-03-23
Country of Publication
Egypt
No. of Pages
14
Main Subjects
Abstract EN
Support vector machine (SVM) is a powerful technique for classification.
However, SVM is not suitable for classification of large datasets or text corpora, because the training complexity of SVMs is highly dependent on the input size.
Recent developments in the literature on the SVM and other kernel methods emphasize the need to consider multiple kernels or parameterizations of kernels because they provide greater flexibility.
This paper shows a multikernel SVM to manage highly dimensional data, providing an automatic parameterization with low computational cost and improving results against SVMs parameterized under a brute-force search.
The model consists in spreading the dataset into cohesive term slices (clusters) to construct a defined structure (multikernel).
The new approach is tested on different text corpora.
Experimental results show that the new classifier has good accuracy compared with the classic SVM, while the training is significantly faster than several other SVM classifiers.
American Psychological Association (APA)
Romero, R.& Iglesias, E. L.& Borrajo, L.. 2015. A Linear-RBF Multikernel SVM to Classify Big Text Corpora. BioMed Research International،Vol. 2015, no. 2015, pp.1-14.
https://search.emarefa.net/detail/BIM-1057116
Modern Language Association (MLA)
Romero, R.…[et al.]. A Linear-RBF Multikernel SVM to Classify Big Text Corpora. BioMed Research International No. 2015 (2015), pp.1-14.
https://search.emarefa.net/detail/BIM-1057116
American Medical Association (AMA)
Romero, R.& Iglesias, E. L.& Borrajo, L.. A Linear-RBF Multikernel SVM to Classify Big Text Corpora. BioMed Research International. 2015. Vol. 2015, no. 2015, pp.1-14.
https://search.emarefa.net/detail/BIM-1057116
Data Type
Journal Articles
Language
English
Notes
Includes bibliographical references
Record ID
BIM-1057116