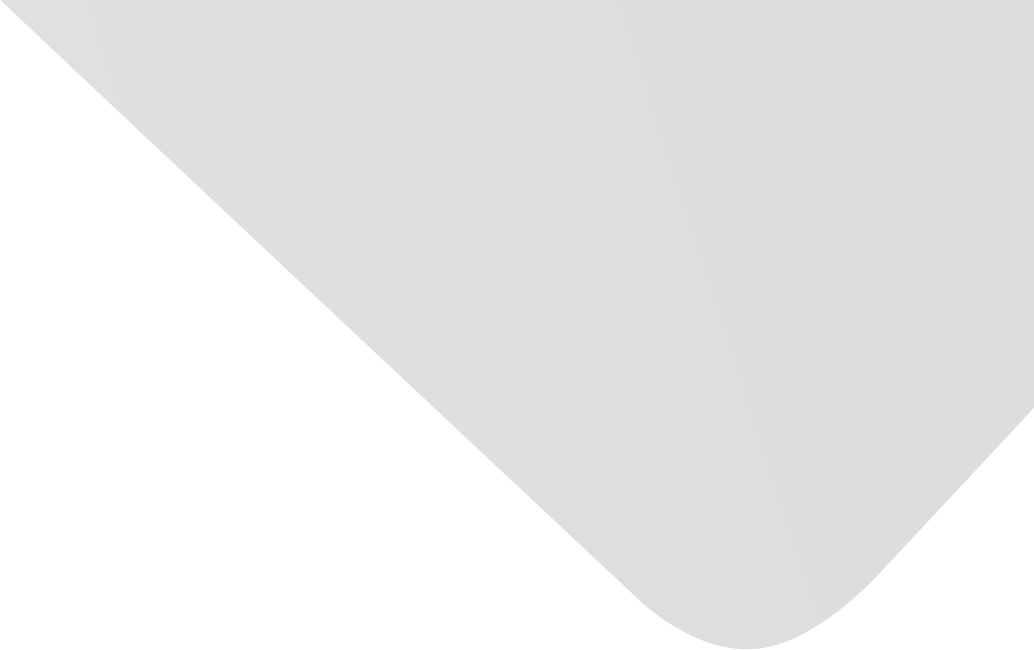
K -Profiles: A Nonlinear Clustering Method for Pattern Detection in High Dimensional Data
Joint Authors
Wang, Kai
Zhao, Qing
Lu, Jianwei
Yu, Tianwei
Source
Issue
Vol. 2015, Issue 2015 (31 Dec. 2015), pp.1-10, 10 p.
Publisher
Hindawi Publishing Corporation
Publication Date
2015-08-03
Country of Publication
Egypt
No. of Pages
10
Main Subjects
Abstract EN
With modern technologies such as microarray, deep sequencing, and liquid chromatography-mass spectrometry (LC-MS), it is possible to measure the expression levels of thousands of genes/proteins simultaneously to unravel important biological processes.
A very first step towards elucidating hidden patterns and understanding the massive data is the application of clustering techniques.
Nonlinear relations, which were mostly unutilized in contrast to linear correlations, are prevalent in high-throughput data.
In many cases, nonlinear relations can model the biological relationship more precisely and reflect critical patterns in the biological systems.
Using the general dependency measure, Distance Based on Conditional Ordered List (DCOL) that we introduced before, we designed the nonlinear K-profiles clustering method, which can be seen as the nonlinear counterpart of the K-means clustering algorithm.
The method has a built-in statistical testing procedure that ensures genes not belonging to any cluster do not impact the estimation of cluster profiles.
Results from extensive simulation studies showed that K-profiles clustering not only outperformed traditional linear K-means algorithm, but also presented significantly better performance over our previous General Dependency Hierarchical Clustering (GDHC) algorithm.
We further analyzed a gene expression dataset, on which K-profile clustering generated biologically meaningful results.
American Psychological Association (APA)
Wang, Kai& Zhao, Qing& Lu, Jianwei& Yu, Tianwei. 2015. K -Profiles: A Nonlinear Clustering Method for Pattern Detection in High Dimensional Data. BioMed Research International،Vol. 2015, no. 2015, pp.1-10.
https://search.emarefa.net/detail/BIM-1057242
Modern Language Association (MLA)
Wang, Kai…[et al.]. K -Profiles: A Nonlinear Clustering Method for Pattern Detection in High Dimensional Data. BioMed Research International No. 2015 (2015), pp.1-10.
https://search.emarefa.net/detail/BIM-1057242
American Medical Association (AMA)
Wang, Kai& Zhao, Qing& Lu, Jianwei& Yu, Tianwei. K -Profiles: A Nonlinear Clustering Method for Pattern Detection in High Dimensional Data. BioMed Research International. 2015. Vol. 2015, no. 2015, pp.1-10.
https://search.emarefa.net/detail/BIM-1057242
Data Type
Journal Articles
Language
English
Notes
Includes bibliographical references
Record ID
BIM-1057242