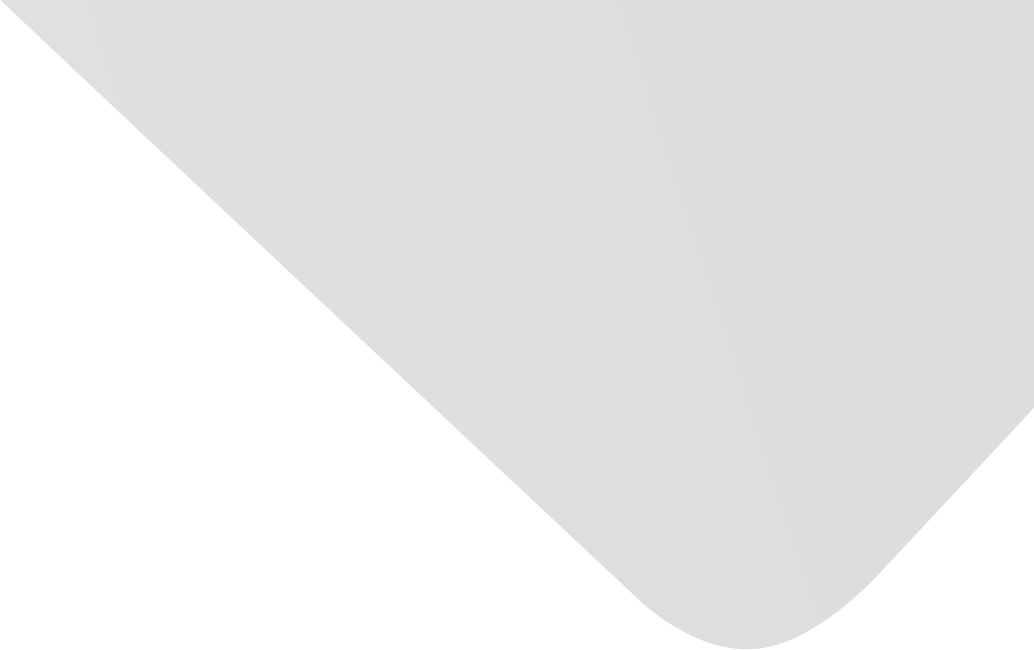
An Enhanced Differential Evolution Algorithm Based on Multiple Mutation Strategies
Joint Authors
Meng, Xuelei
Gao, Mingxia
An, Mei-qing
Li, Yin-zhen
Xiang, Wan-li
Source
Computational Intelligence and Neuroscience
Issue
Vol. 2015, Issue 2015 (31 Dec. 2015), pp.1-15, 15 p.
Publisher
Hindawi Publishing Corporation
Publication Date
2015-11-01
Country of Publication
Egypt
No. of Pages
15
Main Subjects
Abstract EN
Differential evolution algorithm is a simple yet efficient metaheuristic for global optimization over continuous spaces.
However, there is a shortcoming of premature convergence in standard DE, especially in DE/best/1/bin.
In order to take advantage of direction guidance information of the best individual of DE/best/1/bin and avoid getting into local trap, based on multiple mutation strategies, an enhanced differential evolution algorithm, named EDE, is proposed in this paper.
In the EDE algorithm, an initialization technique, opposition-based learning initialization for improving the initial solution quality, and a new combined mutation strategy composed of DE/current/1/bin together with DE/pbest/bin/1 for the sake of accelerating standard DE and preventing DE from clustering around the global best individual, as well as a perturbation scheme for further avoiding premature convergence, are integrated.
In addition, we also introduce two linear time-varying functions, which are used to decide which solution search equation is chosen at the phases of mutation and perturbation, respectively.
Experimental results tested on twenty-five benchmark functions show that EDE is far better than the standard DE.
In further comparisons, EDE is compared with other five state-of-the-art approaches and related results show that EDE is still superior to or at least equal to these methods on most of benchmark functions.
American Psychological Association (APA)
Xiang, Wan-li& Meng, Xuelei& An, Mei-qing& Li, Yin-zhen& Gao, Mingxia. 2015. An Enhanced Differential Evolution Algorithm Based on Multiple Mutation Strategies. Computational Intelligence and Neuroscience،Vol. 2015, no. 2015, pp.1-15.
https://search.emarefa.net/detail/BIM-1057678
Modern Language Association (MLA)
Xiang, Wan-li…[et al.]. An Enhanced Differential Evolution Algorithm Based on Multiple Mutation Strategies. Computational Intelligence and Neuroscience No. 2015 (2015), pp.1-15.
https://search.emarefa.net/detail/BIM-1057678
American Medical Association (AMA)
Xiang, Wan-li& Meng, Xuelei& An, Mei-qing& Li, Yin-zhen& Gao, Mingxia. An Enhanced Differential Evolution Algorithm Based on Multiple Mutation Strategies. Computational Intelligence and Neuroscience. 2015. Vol. 2015, no. 2015, pp.1-15.
https://search.emarefa.net/detail/BIM-1057678
Data Type
Journal Articles
Language
English
Notes
Includes bibliographical references
Record ID
BIM-1057678