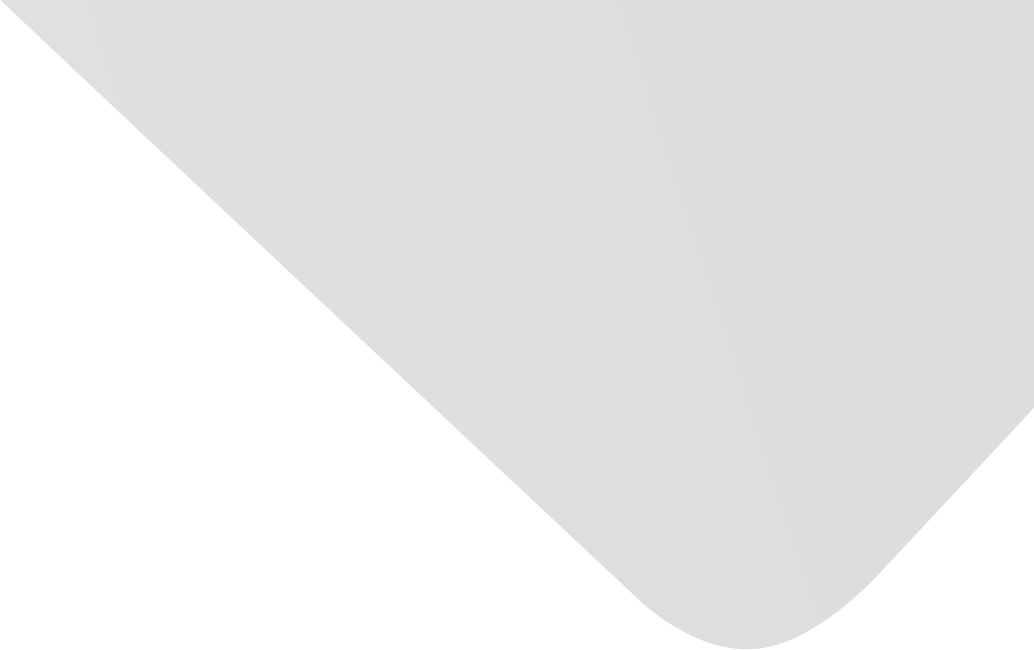
Symmetry Based Automatic Evolution of Clusters: A New Approach to Data Clustering
Joint Authors
Source
Computational Intelligence and Neuroscience
Issue
Vol. 2015, Issue 2015 (31 Dec. 2015), pp.1-21, 21 p.
Publisher
Hindawi Publishing Corporation
Publication Date
2015-08-03
Country of Publication
Egypt
No. of Pages
21
Main Subjects
Abstract EN
We present a multiobjective genetic clustering approach, in which data points are assigned to clusters based on new line symmetry distance.
The proposed algorithm is called multiobjective line symmetry based genetic clustering (MOLGC).
Two objective functions, first the Davies-Bouldin (DB) index and second the line symmetry distance based objective functions, are used.
The proposed algorithm evolves near-optimal clustering solutions using multiple clustering criteria, without a priori knowledge of the actual number of clusters.
The multiple randomized K dimensional (Kd) trees based nearest neighbor search is used to reduce the complexity of finding the closest symmetric points.
Experimental results based on several artificial and real data sets show that proposed clustering algorithm can obtain optimal clustering solutions in terms of different cluster quality measures in comparison to existing SBKM and MOCK clustering algorithms.
American Psychological Association (APA)
Vijendra, Singh& Laxman, Sahoo. 2015. Symmetry Based Automatic Evolution of Clusters: A New Approach to Data Clustering. Computational Intelligence and Neuroscience،Vol. 2015, no. 2015, pp.1-21.
https://search.emarefa.net/detail/BIM-1057756
Modern Language Association (MLA)
Vijendra, Singh& Laxman, Sahoo. Symmetry Based Automatic Evolution of Clusters: A New Approach to Data Clustering. Computational Intelligence and Neuroscience No. 2015 (2015), pp.1-21.
https://search.emarefa.net/detail/BIM-1057756
American Medical Association (AMA)
Vijendra, Singh& Laxman, Sahoo. Symmetry Based Automatic Evolution of Clusters: A New Approach to Data Clustering. Computational Intelligence and Neuroscience. 2015. Vol. 2015, no. 2015, pp.1-21.
https://search.emarefa.net/detail/BIM-1057756
Data Type
Journal Articles
Language
English
Notes
Includes bibliographical references
Record ID
BIM-1057756