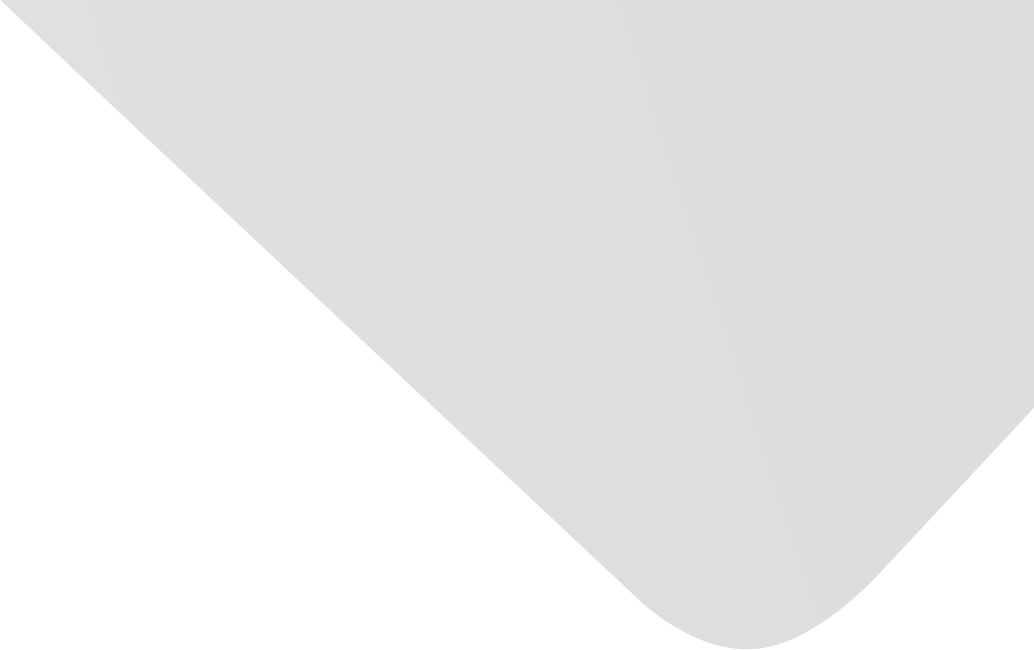
Application of Random Forest Survival Models to Increase Generalizability of Decision Trees: A Case Study in Acute Myocardial Infarction
Joint Authors
Baneshi, Mohammad Reza
Yosefian, Iman
Mosa Farkhani, Ehsan
Source
Computational and Mathematical Methods in Medicine
Issue
Vol. 2015, Issue 2015 (31 Dec. 2015), pp.1-6, 6 p.
Publisher
Hindawi Publishing Corporation
Publication Date
2015-12-21
Country of Publication
Egypt
No. of Pages
6
Main Subjects
Abstract EN
Background.
Tree models provide easily interpretable prognostic tool, but instable results.
Two approaches to enhance the generalizability of the results are pruning and random survival forest (RSF).
The aim of this study is to assess the generalizability of saturated tree (ST), pruned tree (PT), and RSF.
Methods.
Data of 607 patients was randomly divided into training and test set applying 10-fold cross-validation.
Using training sets, all three models were applied.
Using Log-Rank test, ST was constructed by searching for optimal cutoffs.
PT was selected plotting error rate versus minimum sample size in terminal nodes.
In construction of RSF, 1000 bootstrap samples were drawn from the training set.
C-index and integrated Brier score (IBS) statistic were used to compare models.
Results.
ST provides the most overoptimized statistics.
Mean difference between C-index in training and test set was 0.237.
Corresponding figure in PT and RSF was 0.054 and 0.007.
In terms of IBS, the difference was 0.136 in ST, 0.021 in PT, and 0.0003 in RSF.
Conclusion.
Pruning of tree and assessment of its performance of a test set partially improve the generalizability of decision trees.
RSF provides results that are highly generalizable.
American Psychological Association (APA)
Yosefian, Iman& Mosa Farkhani, Ehsan& Baneshi, Mohammad Reza. 2015. Application of Random Forest Survival Models to Increase Generalizability of Decision Trees: A Case Study in Acute Myocardial Infarction. Computational and Mathematical Methods in Medicine،Vol. 2015, no. 2015, pp.1-6.
https://search.emarefa.net/detail/BIM-1057942
Modern Language Association (MLA)
Yosefian, Iman…[et al.]. Application of Random Forest Survival Models to Increase Generalizability of Decision Trees: A Case Study in Acute Myocardial Infarction. Computational and Mathematical Methods in Medicine No. 2015 (2015), pp.1-6.
https://search.emarefa.net/detail/BIM-1057942
American Medical Association (AMA)
Yosefian, Iman& Mosa Farkhani, Ehsan& Baneshi, Mohammad Reza. Application of Random Forest Survival Models to Increase Generalizability of Decision Trees: A Case Study in Acute Myocardial Infarction. Computational and Mathematical Methods in Medicine. 2015. Vol. 2015, no. 2015, pp.1-6.
https://search.emarefa.net/detail/BIM-1057942
Data Type
Journal Articles
Language
English
Notes
Includes bibliographical references
Record ID
BIM-1057942