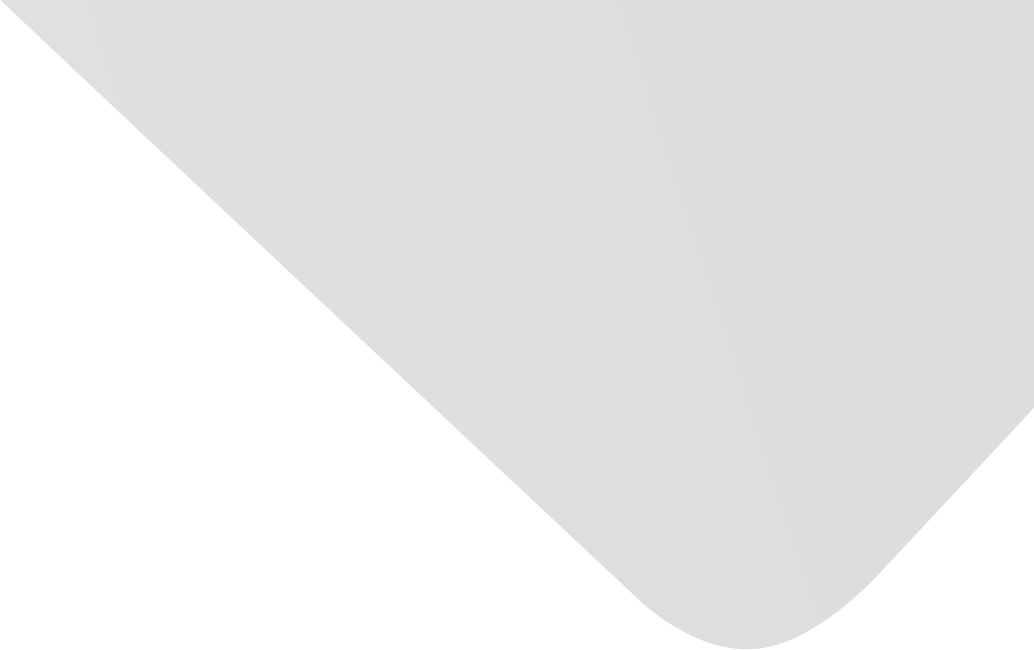
Analysis of Generalization Ability for Different AdaBoost Variants Based on Classification and Regression Trees
Joint Authors
Wu, Shuqiong
Nagahashi, Hiroshi
Source
Journal of Electrical and Computer Engineering
Issue
Vol. 2015, Issue 2015 (31 Dec. 2015), pp.1-17, 17 p.
Publisher
Hindawi Publishing Corporation
Publication Date
2015-02-10
Country of Publication
Egypt
No. of Pages
17
Main Subjects
Information Technology and Computer Science
Abstract EN
As a machine learning method, AdaBoost is widely applied to data classification and object detection because of its robustness and efficiency.
AdaBoost constructs a global and optimal combination of weak classifiers based on a sample reweighting.
It is known that this kind of combination improves the classification performance tremendously.
As the popularity of AdaBoost increases, many variants have been proposed to improve the performance of AdaBoost.
Then, a lot of comparison and review studies for AdaBoost variants have also been published.
Some researchers compared different AdaBoost variants by experiments in their own fields, and others reviewed various AdaBoost variants by basically introducing these algorithms.
However, there is a lack of mathematical analysis of the generalization abilities for different AdaBoost variants.
In this paper, we analyze the generalization abilities of six AdaBoost variants in terms of classification margins.
The six compared variants are Real AdaBoost, Gentle AdaBoost, Modest AdaBoost, Parameterized AdaBoost, Margin-pruning Boost, and Penalized AdaBoost.
Finally, we use experiments to verify our analyses.
American Psychological Association (APA)
Wu, Shuqiong& Nagahashi, Hiroshi. 2015. Analysis of Generalization Ability for Different AdaBoost Variants Based on Classification and Regression Trees. Journal of Electrical and Computer Engineering،Vol. 2015, no. 2015, pp.1-17.
https://search.emarefa.net/detail/BIM-1068149
Modern Language Association (MLA)
Wu, Shuqiong& Nagahashi, Hiroshi. Analysis of Generalization Ability for Different AdaBoost Variants Based on Classification and Regression Trees. Journal of Electrical and Computer Engineering No. 2015 (2015), pp.1-17.
https://search.emarefa.net/detail/BIM-1068149
American Medical Association (AMA)
Wu, Shuqiong& Nagahashi, Hiroshi. Analysis of Generalization Ability for Different AdaBoost Variants Based on Classification and Regression Trees. Journal of Electrical and Computer Engineering. 2015. Vol. 2015, no. 2015, pp.1-17.
https://search.emarefa.net/detail/BIM-1068149
Data Type
Journal Articles
Language
English
Notes
Includes bibliographical references
Record ID
BIM-1068149