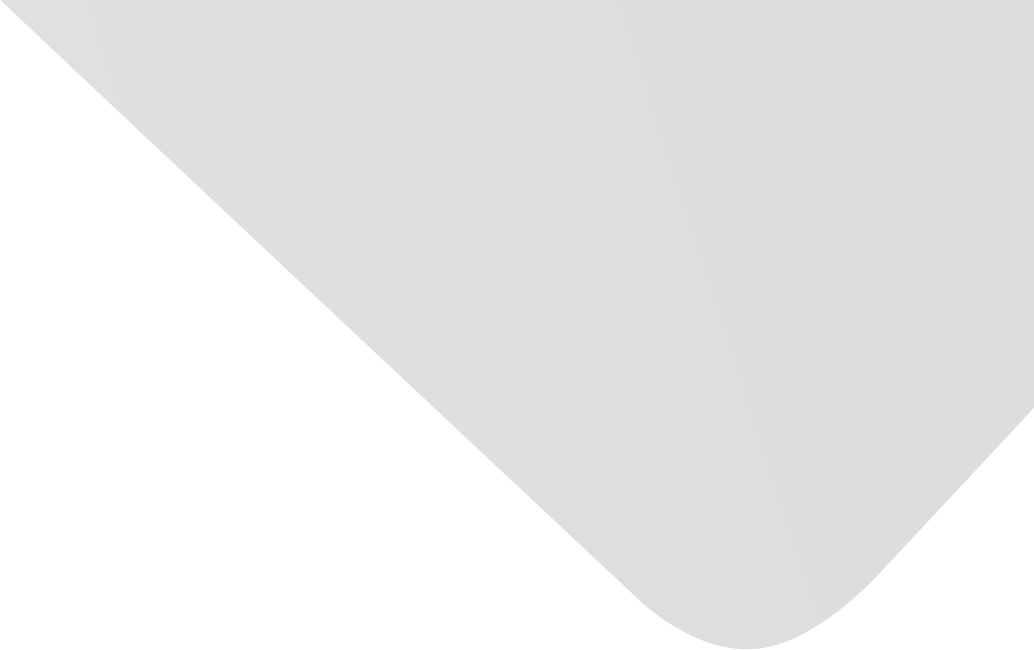
Research on Combinational Forecast Models for the Traffic Flow
Joint Authors
Yu, Zhiheng
Sun, Tieli
Sun, Hongguang
Yang, Fengqin
Source
Mathematical Problems in Engineering
Issue
Vol. 2015, Issue 2015 (31 Dec. 2015), pp.1-10, 10 p.
Publisher
Hindawi Publishing Corporation
Publication Date
2015-10-04
Country of Publication
Egypt
No. of Pages
10
Main Subjects
Abstract EN
In order to improve the prediction accuracy of the traffic flow, this paper proposes two combinational forecast models based on GM, ARIMA, and GRNN.
Firstly, the paper proposes the concept of associate-forecast and the weight distribution method based on reciprocal absolute percentage error and then uses GM(1,1), ARIMA, and GRNN to establish a combinational model of highway traffic flow according to the fixed weight coefficients.
Then the paper proposes the use of neural networks to determine variable weight coefficients and establishes Elman combinational forecast model based on GM(1,1), ARIMA, and GRNN, which achieves the integration of these three individuals.
Lastly, these two combinational models are applied to highway traffic flow on Chongzun of China and the experimental results verify their effectiveness compared with GM(1,1), ARIMA, and GRNN.
American Psychological Association (APA)
Yu, Zhiheng& Sun, Tieli& Sun, Hongguang& Yang, Fengqin. 2015. Research on Combinational Forecast Models for the Traffic Flow. Mathematical Problems in Engineering،Vol. 2015, no. 2015, pp.1-10.
https://search.emarefa.net/detail/BIM-1073205
Modern Language Association (MLA)
Yu, Zhiheng…[et al.]. Research on Combinational Forecast Models for the Traffic Flow. Mathematical Problems in Engineering No. 2015 (2015), pp.1-10.
https://search.emarefa.net/detail/BIM-1073205
American Medical Association (AMA)
Yu, Zhiheng& Sun, Tieli& Sun, Hongguang& Yang, Fengqin. Research on Combinational Forecast Models for the Traffic Flow. Mathematical Problems in Engineering. 2015. Vol. 2015, no. 2015, pp.1-10.
https://search.emarefa.net/detail/BIM-1073205
Data Type
Journal Articles
Language
English
Notes
Includes bibliographical references
Record ID
BIM-1073205