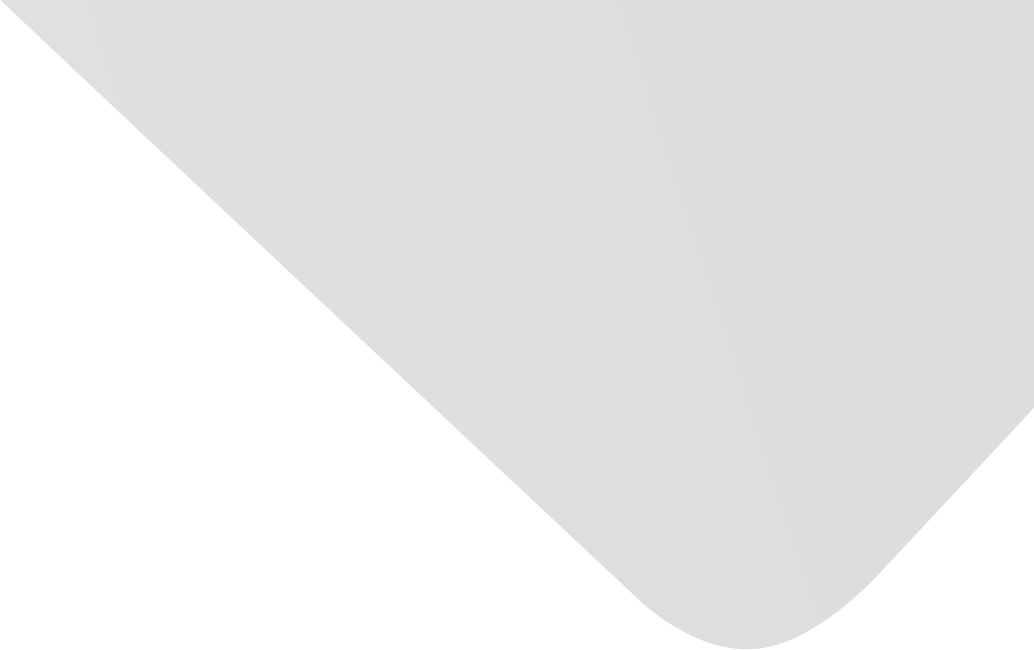
Obtaining Cross Modal Similarity Metric with Deep Neural Architecture
Joint Authors
Li, Ruifan
Feng, Fangxiang
Wang, Xiaojie
Lu, Peng
Li, Bohan
Source
Mathematical Problems in Engineering
Issue
Vol. 2015, Issue 2015 (31 Dec. 2015), pp.1-9, 9 p.
Publisher
Hindawi Publishing Corporation
Publication Date
2015-04-23
Country of Publication
Egypt
No. of Pages
9
Main Subjects
Abstract EN
Analyzing complex system with multimodal data, such as image and text, has recently received tremendous attention.
Modeling the relationship between different modalities is the key to address this problem.
Motivated by recent successful applications of deep neural learning in unimodal data, in this paper, we propose a computational deep neural architecture, bimodal deep architecture (BDA) for measuring the similarity between different modalities.
Our proposed BDA architecture has three closely related consecutive components.
For image and text modalities, the first component can be constructed using some popular feature extraction methods in their individual modalities.
The second component has two types of stacked restricted Boltzmann machines (RBMs).
Specifically, for image modality a binary-binary RBM is stacked over a Gaussian-binary RBM; for text modality a binary-binary RBM is stacked over a replicated softmax RBM.
In the third component, we come up with a variant autoencoder with a predefined loss function for discriminatively learning the regularity between different modalities.
We show experimentally the effectiveness of our approach to the task of classifying image tags on public available datasets.
American Psychological Association (APA)
Li, Ruifan& Feng, Fangxiang& Wang, Xiaojie& Lu, Peng& Li, Bohan. 2015. Obtaining Cross Modal Similarity Metric with Deep Neural Architecture. Mathematical Problems in Engineering،Vol. 2015, no. 2015, pp.1-9.
https://search.emarefa.net/detail/BIM-1073443
Modern Language Association (MLA)
Li, Ruifan…[et al.]. Obtaining Cross Modal Similarity Metric with Deep Neural Architecture. Mathematical Problems in Engineering No. 2015 (2015), pp.1-9.
https://search.emarefa.net/detail/BIM-1073443
American Medical Association (AMA)
Li, Ruifan& Feng, Fangxiang& Wang, Xiaojie& Lu, Peng& Li, Bohan. Obtaining Cross Modal Similarity Metric with Deep Neural Architecture. Mathematical Problems in Engineering. 2015. Vol. 2015, no. 2015, pp.1-9.
https://search.emarefa.net/detail/BIM-1073443
Data Type
Journal Articles
Language
English
Notes
Includes bibliographical references
Record ID
BIM-1073443