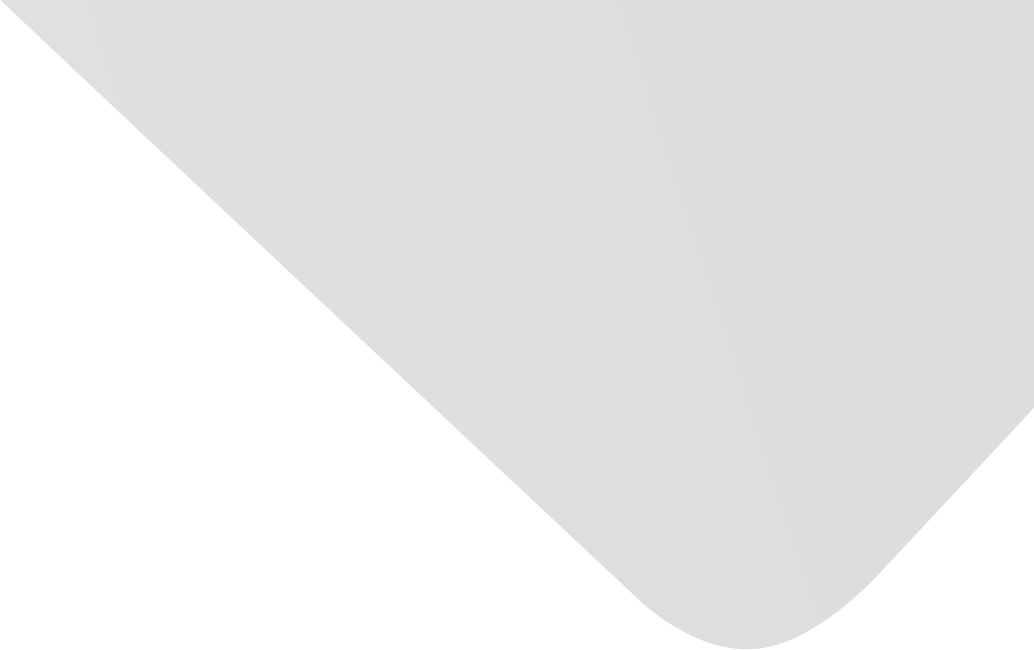
One-Class Classification with Extreme Learning Machine
Joint Authors
Qi, Honggang
Miao, Jun
Leng, Qian
Zhu, Wentao
Su, Guiping
Source
Mathematical Problems in Engineering
Issue
Vol. 2015, Issue 2015 (31 Dec. 2015), pp.1-11, 11 p.
Publisher
Hindawi Publishing Corporation
Publication Date
2015-05-26
Country of Publication
Egypt
No. of Pages
11
Main Subjects
Abstract EN
One-class classification problem has been investigated thoroughly for past decades.
Among one of the most effective neural network approaches for one-class classification, autoencoder has been successfully applied for many applications.
However, this classifier relies on traditional learning algorithms such as backpropagation to train the network, which is quite time-consuming.
To tackle the slow learning speed in autoencoder neural network, we propose a simple and efficient one-class classifier based on extreme learning machine (ELM).
The essence of ELM is that the hidden layer need not be tuned and the output weights can be analytically determined, which leads to much faster learning speed.
The experimental evaluation conducted on several real-world benchmarks shows that the ELM based one-class classifier can learn hundreds of times faster than autoencoder and it is competitive over a variety of one-class classification methods.
American Psychological Association (APA)
Leng, Qian& Qi, Honggang& Miao, Jun& Zhu, Wentao& Su, Guiping. 2015. One-Class Classification with Extreme Learning Machine. Mathematical Problems in Engineering،Vol. 2015, no. 2015, pp.1-11.
https://search.emarefa.net/detail/BIM-1073781
Modern Language Association (MLA)
Leng, Qian…[et al.]. One-Class Classification with Extreme Learning Machine. Mathematical Problems in Engineering No. 2015 (2015), pp.1-11.
https://search.emarefa.net/detail/BIM-1073781
American Medical Association (AMA)
Leng, Qian& Qi, Honggang& Miao, Jun& Zhu, Wentao& Su, Guiping. One-Class Classification with Extreme Learning Machine. Mathematical Problems in Engineering. 2015. Vol. 2015, no. 2015, pp.1-11.
https://search.emarefa.net/detail/BIM-1073781
Data Type
Journal Articles
Language
English
Notes
Includes bibliographical references
Record ID
BIM-1073781