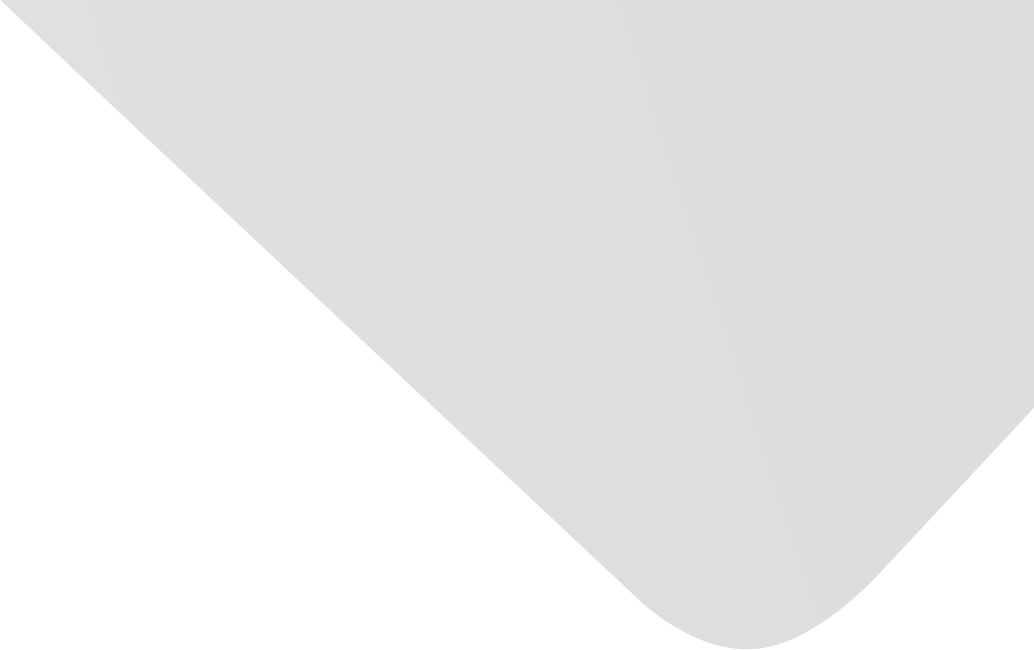
Compressed Sensing MRI Reconstruction from Highly Undersampled k-Space Data Using Nonsubsampled Shearlet Transform Sparsity Prior
Joint Authors
Yuan, Min
Yang, Bingxin
Ma, Yide
Zhang, Jiuwen
Zhang, Runpu
Zhang, Caiyuan
Source
Mathematical Problems in Engineering
Issue
Vol. 2015, Issue 2015 (31 Dec. 2015), pp.1-18, 18 p.
Publisher
Hindawi Publishing Corporation
Publication Date
2015-03-25
Country of Publication
Egypt
No. of Pages
18
Main Subjects
Abstract EN
Compressed sensing has shown great potential in speeding up MR imaging by undersampling k-space data.
Generally sparsity is used as a priori knowledge to improve the quality of reconstructed image.
Compressed sensing MR image (CS-MRI) reconstruction methods have employed widely used sparsifying transforms such as wavelet or total variation, which are not preeminent in dealing with MR images containing distributed discontinuities and cannot provide a sufficient sparse representation and the decomposition at any direction.
In this paper, we propose a novel CS-MRI reconstruction method from highly undersampled k-space data using nonsubsampled shearlet transform (NSST) sparsity prior.
In particular, we have implemented a flexible decomposition with an arbitrary even number of directional subbands at each level using NSST for MR images.
The highly directional sensitivity of NSST and its optimal approximation properties lead to improvement in CS-MRI reconstruction applications.
The experimental results demonstrate that the proposed method results in the high quality reconstruction, which is highly effective at preserving the intrinsic anisotropic features of MRI meanwhile suppressing the artifacts and added noise.
The objective evaluation indices outperform all compared CS-MRI methods.
In summary, NSST with even number directional decomposition is very competitive in CS-MRI applications as sparsity prior in terms of performance and computational efficiency.
American Psychological Association (APA)
Yuan, Min& Yang, Bingxin& Ma, Yide& Zhang, Jiuwen& Zhang, Runpu& Zhang, Caiyuan. 2015. Compressed Sensing MRI Reconstruction from Highly Undersampled k-Space Data Using Nonsubsampled Shearlet Transform Sparsity Prior. Mathematical Problems in Engineering،Vol. 2015, no. 2015, pp.1-18.
https://search.emarefa.net/detail/BIM-1074291
Modern Language Association (MLA)
Yuan, Min…[et al.]. Compressed Sensing MRI Reconstruction from Highly Undersampled k-Space Data Using Nonsubsampled Shearlet Transform Sparsity Prior. Mathematical Problems in Engineering No. 2015 (2015), pp.1-18.
https://search.emarefa.net/detail/BIM-1074291
American Medical Association (AMA)
Yuan, Min& Yang, Bingxin& Ma, Yide& Zhang, Jiuwen& Zhang, Runpu& Zhang, Caiyuan. Compressed Sensing MRI Reconstruction from Highly Undersampled k-Space Data Using Nonsubsampled Shearlet Transform Sparsity Prior. Mathematical Problems in Engineering. 2015. Vol. 2015, no. 2015, pp.1-18.
https://search.emarefa.net/detail/BIM-1074291
Data Type
Journal Articles
Language
English
Notes
Includes bibliographical references
Record ID
BIM-1074291