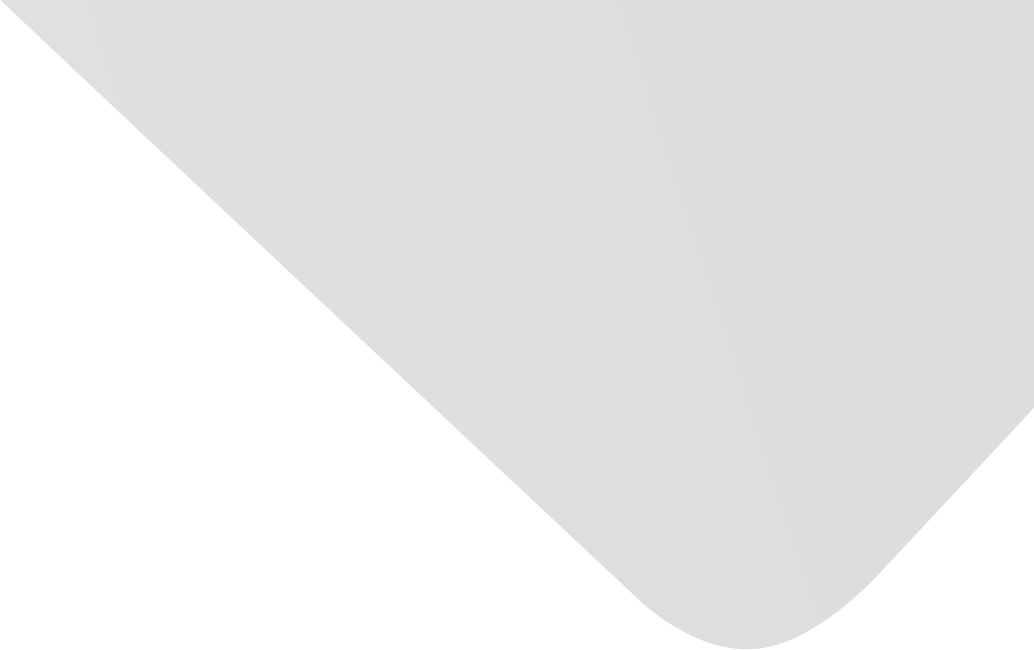
Head Pose Estimation with Improved Random Regression Forests
Joint Authors
Sang, Gaoli
Chen, Hu
Zhao, Qijun
Source
Mathematical Problems in Engineering
Issue
Vol. 2015, Issue 2015 (31 Dec. 2015), pp.1-11, 11 p.
Publisher
Hindawi Publishing Corporation
Publication Date
2015-10-12
Country of Publication
Egypt
No. of Pages
11
Main Subjects
Abstract EN
Perception of head pose is useful for many face-related tasks such as face recognition, gaze estimation, and emotion analysis.
In this paper, we propose a novel random forest based method for estimating head pose angles from single face images.
In order to improve the effectiveness of the constructed head pose predictor, we introduce feature weighting and tree screening into the random forest training process.
In this way, the features with more discriminative power are more likely to be chosen for constructing trees, and each of the trees in the obtained random forest usually has high pose estimation accuracy, while the diversity or generalization ability of the forest is not deteriorated.
The proposed method has been evaluated on four public databases as well as a surveillance dataset collected by ourselves.
The results show that the proposed method can achieve state-of-the-art pose estimation accuracy.
Moreover, we investigate the impact of pose angle sampling intervals and heterogeneous face images on the effectiveness of trained head pose predictors.
American Psychological Association (APA)
Sang, Gaoli& Chen, Hu& Zhao, Qijun. 2015. Head Pose Estimation with Improved Random Regression Forests. Mathematical Problems in Engineering،Vol. 2015, no. 2015, pp.1-11.
https://search.emarefa.net/detail/BIM-1074516
Modern Language Association (MLA)
Sang, Gaoli…[et al.]. Head Pose Estimation with Improved Random Regression Forests. Mathematical Problems in Engineering No. 2015 (2015), pp.1-11.
https://search.emarefa.net/detail/BIM-1074516
American Medical Association (AMA)
Sang, Gaoli& Chen, Hu& Zhao, Qijun. Head Pose Estimation with Improved Random Regression Forests. Mathematical Problems in Engineering. 2015. Vol. 2015, no. 2015, pp.1-11.
https://search.emarefa.net/detail/BIM-1074516
Data Type
Journal Articles
Language
English
Notes
Includes bibliographical references
Record ID
BIM-1074516