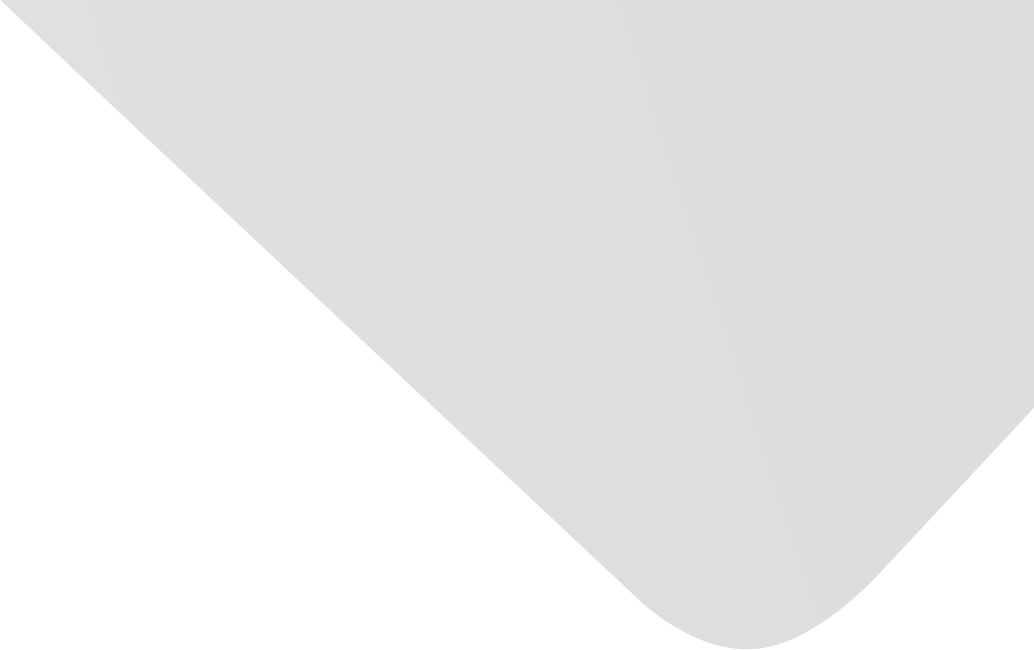
Prediction of Compressive Strength of Concrete Using Artificial Neural Network and Genetic Programming
Joint Authors
Chopra, Palika
Sharma, Rajendra Kumar
Kumar, Maneek
Source
Advances in Materials Science and Engineering
Issue
Vol. 2016, Issue 2016 (31 Dec. 2016), pp.1-10, 10 p.
Publisher
Hindawi Publishing Corporation
Publication Date
2016-01-10
Country of Publication
Egypt
No. of Pages
10
Abstract EN
An effort has been made to develop concrete compressive strength prediction models with the help of two emerging data mining techniques, namely, Artificial Neural Networks (ANNs) and Genetic Programming (GP).
The data for analysis and model development was collected at 28-, 56-, and 91-day curing periods through experiments conducted in the laboratory under standard controlled conditions.
The developed models have also been tested on in situ concrete data taken from literature.
A comparison of the prediction results obtained using both the models is presented and it can be inferred that the ANN model with the training function Levenberg-Marquardt (LM) for the prediction of concrete compressive strength is the best prediction tool.
American Psychological Association (APA)
Chopra, Palika& Sharma, Rajendra Kumar& Kumar, Maneek. 2016. Prediction of Compressive Strength of Concrete Using Artificial Neural Network and Genetic Programming. Advances in Materials Science and Engineering،Vol. 2016, no. 2016, pp.1-10.
https://search.emarefa.net/detail/BIM-1096402
Modern Language Association (MLA)
Chopra, Palika…[et al.]. Prediction of Compressive Strength of Concrete Using Artificial Neural Network and Genetic Programming. Advances in Materials Science and Engineering No. 2016 (2016), pp.1-10.
https://search.emarefa.net/detail/BIM-1096402
American Medical Association (AMA)
Chopra, Palika& Sharma, Rajendra Kumar& Kumar, Maneek. Prediction of Compressive Strength of Concrete Using Artificial Neural Network and Genetic Programming. Advances in Materials Science and Engineering. 2016. Vol. 2016, no. 2016, pp.1-10.
https://search.emarefa.net/detail/BIM-1096402
Data Type
Journal Articles
Language
English
Notes
Includes bibliographical references
Record ID
BIM-1096402