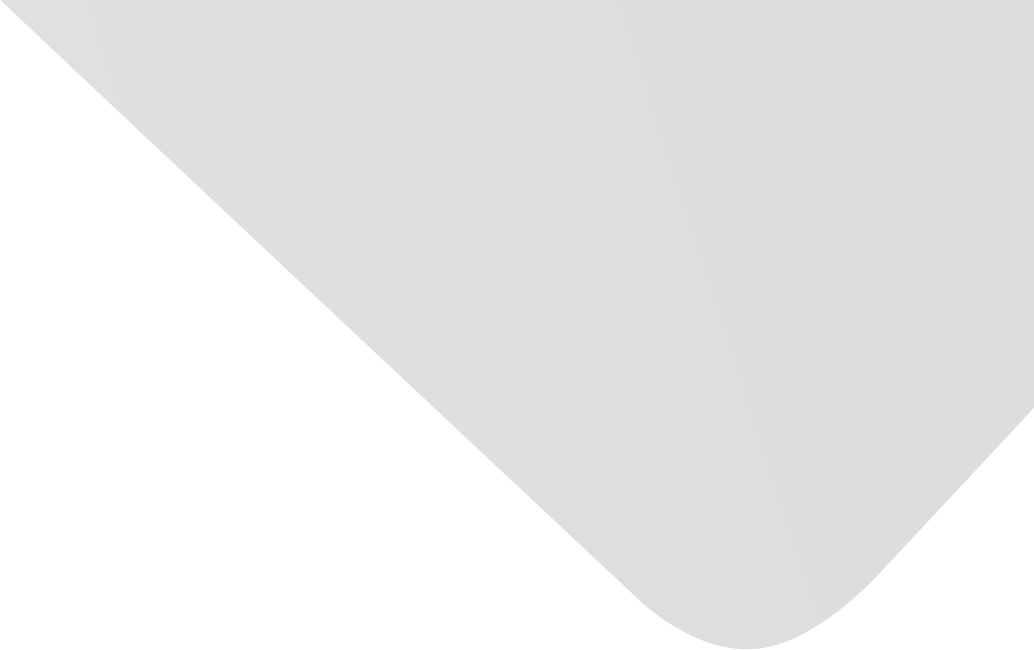
Protein Remote Homology Detection Based on an Ensemble Learning Approach
Joint Authors
Chen, Junjie
Liu, Bingquan
Huang, Dong
Source
Issue
Vol. 2016, Issue 2016 (31 Dec. 2016), pp.1-11, 11 p.
Publisher
Hindawi Publishing Corporation
Publication Date
2016-05-08
Country of Publication
Egypt
No. of Pages
11
Main Subjects
Abstract EN
Protein remote homology detection is one of the central problems in bioinformatics.
Although some computational methods have been proposed, the problem is still far from being solved.
In this paper, an ensemble classifier for protein remote homology detection, called SVM-Ensemble, was proposed with a weighted voting strategy.
SVM-Ensemble combined three basic classifiers based on different feature spaces, including Kmer, ACC, and SC-PseAAC.
These features consider the characteristics of proteins from various perspectives, incorporating both the sequence composition and the sequence-order information along the protein sequences.
Experimental results on a widely used benchmark dataset showed that the proposed SVM-Ensemble can obviously improve the predictive performance for the protein remote homology detection.
Moreover, it achieved the best performance and outperformed other state-of-the-art methods.
American Psychological Association (APA)
Chen, Junjie& Liu, Bingquan& Huang, Dong. 2016. Protein Remote Homology Detection Based on an Ensemble Learning Approach. BioMed Research International،Vol. 2016, no. 2016, pp.1-11.
https://search.emarefa.net/detail/BIM-1098224
Modern Language Association (MLA)
Chen, Junjie…[et al.]. Protein Remote Homology Detection Based on an Ensemble Learning Approach. BioMed Research International No. 2016 (2016), pp.1-11.
https://search.emarefa.net/detail/BIM-1098224
American Medical Association (AMA)
Chen, Junjie& Liu, Bingquan& Huang, Dong. Protein Remote Homology Detection Based on an Ensemble Learning Approach. BioMed Research International. 2016. Vol. 2016, no. 2016, pp.1-11.
https://search.emarefa.net/detail/BIM-1098224
Data Type
Journal Articles
Language
English
Notes
Includes bibliographical references
Record ID
BIM-1098224