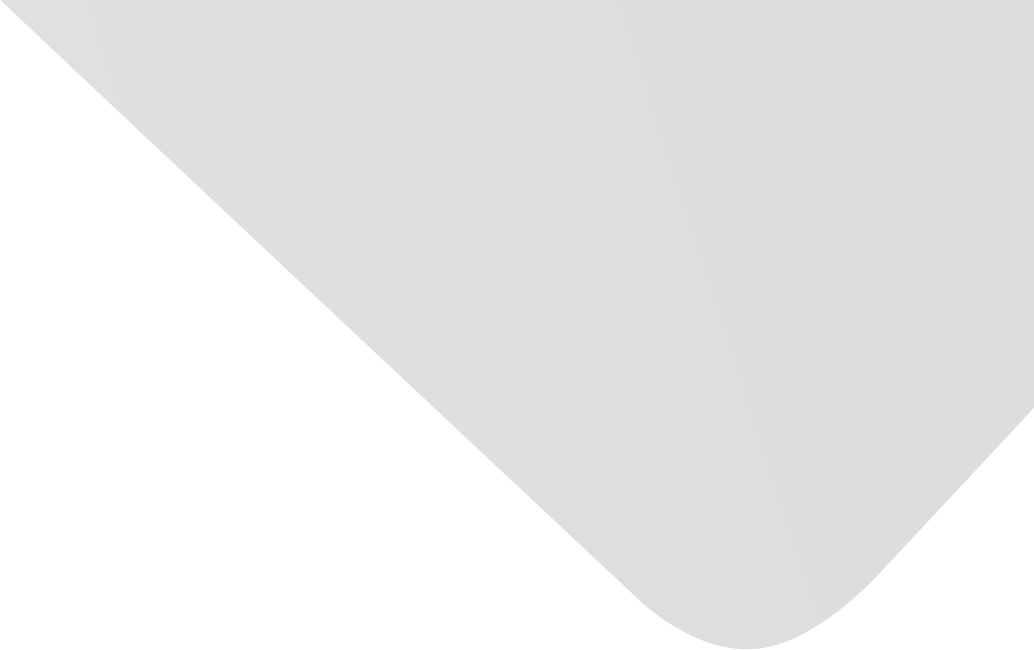
A Feature Selection Approach Based on Interclass and Intraclass Relative Contributions of Terms
Joint Authors
Zhao, Minghua
Zhou, Hongfang
Guo, Jie
Wang, Yinghui
Source
Computational Intelligence and Neuroscience
Issue
Vol. 2016, Issue 2016 (31 Dec. 2015), pp.1-8, 8 p.
Publisher
Hindawi Publishing Corporation
Publication Date
2016-08-08
Country of Publication
Egypt
No. of Pages
8
Main Subjects
Abstract EN
Feature selection plays a critical role in text categorization.
During feature selecting, high-frequency terms and the interclass and intraclass relative contributions of terms all have significant effects on classification results.
So we put forward a feature selection approach, IIRCT, based on interclass and intraclass relative contributions of terms in the paper.
In our proposed algorithm, three critical factors, which are term frequency and the interclass relative contribution and the intraclass relative contribution of terms, are all considered synthetically.
Finally, experiments are made with the help of kNN classifier.
And the corresponding results on 20 NewsGroup and SougouCS corpora show that IIRCT algorithm achieves better performance than DF, t-Test, and CMFS algorithms.
American Psychological Association (APA)
Zhou, Hongfang& Guo, Jie& Wang, Yinghui& Zhao, Minghua. 2016. A Feature Selection Approach Based on Interclass and Intraclass Relative Contributions of Terms. Computational Intelligence and Neuroscience،Vol. 2016, no. 2016, pp.1-8.
https://search.emarefa.net/detail/BIM-1099587
Modern Language Association (MLA)
Zhou, Hongfang…[et al.]. A Feature Selection Approach Based on Interclass and Intraclass Relative Contributions of Terms. Computational Intelligence and Neuroscience Vol. 2016, no. 2016 (2015), pp.1-8.
https://search.emarefa.net/detail/BIM-1099587
American Medical Association (AMA)
Zhou, Hongfang& Guo, Jie& Wang, Yinghui& Zhao, Minghua. A Feature Selection Approach Based on Interclass and Intraclass Relative Contributions of Terms. Computational Intelligence and Neuroscience. 2016. Vol. 2016, no. 2016, pp.1-8.
https://search.emarefa.net/detail/BIM-1099587
Data Type
Journal Articles
Language
English
Notes
Includes bibliographical references
Record ID
BIM-1099587