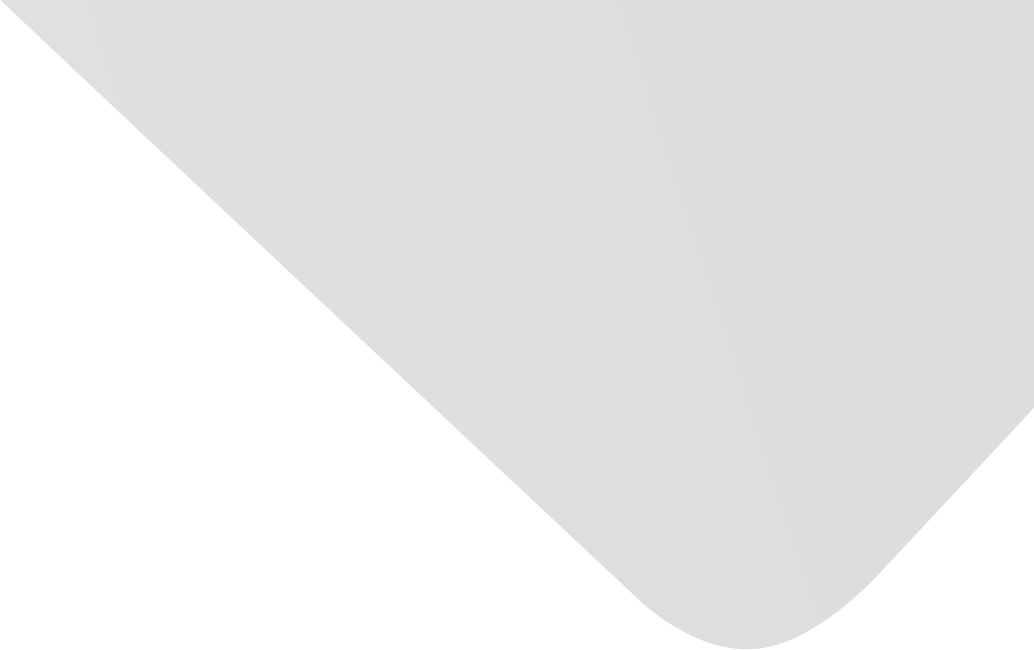
Kernel Recursive Least-Squares Temporal Difference Algorithms with Sparsification and Regularization
Joint Authors
Zhang, Chunyuan
Niu, Xinzheng
Zhu, Qingxin
Source
Computational Intelligence and Neuroscience
Issue
Vol. 2016, Issue 2016 (31 Dec. 2015), pp.1-11, 11 p.
Publisher
Hindawi Publishing Corporation
Publication Date
2016-06-29
Country of Publication
Egypt
No. of Pages
11
Main Subjects
Abstract EN
By combining with sparse kernel methods, least-squares temporal difference (LSTD) algorithms can construct the feature dictionary automatically and obtain a better generalization ability.
However, the previous kernel-based LSTD algorithms do not consider regularization and their sparsification processes are batch or offline, which hinder their widespread applications in online learning problems.
In this paper, we combine the following five techniques and propose two novel kernel recursive LSTD algorithms: (i) online sparsification, which can cope with unknown state regions and be used for online learning, (ii) L2 and L1 regularization, which can avoid overfitting and eliminate the influence of noise, (iii) recursive least squares, which can eliminate matrix-inversion operations and reduce computational complexity, (iv) a sliding-window approach, which can avoid caching all history samples and reduce the computational cost, and (v) the fixed-point subiteration and online pruning, which can make L1 regularization easy to implement.
Finally, simulation results on two 50-state chain problems demonstrate the effectiveness of our algorithms.
American Psychological Association (APA)
Zhang, Chunyuan& Zhu, Qingxin& Niu, Xinzheng. 2016. Kernel Recursive Least-Squares Temporal Difference Algorithms with Sparsification and Regularization. Computational Intelligence and Neuroscience،Vol. 2016, no. 2016, pp.1-11.
https://search.emarefa.net/detail/BIM-1099597
Modern Language Association (MLA)
Zhang, Chunyuan…[et al.]. Kernel Recursive Least-Squares Temporal Difference Algorithms with Sparsification and Regularization. Computational Intelligence and Neuroscience Vol. 2016, no. 2016 (2015), pp.1-11.
https://search.emarefa.net/detail/BIM-1099597
American Medical Association (AMA)
Zhang, Chunyuan& Zhu, Qingxin& Niu, Xinzheng. Kernel Recursive Least-Squares Temporal Difference Algorithms with Sparsification and Regularization. Computational Intelligence and Neuroscience. 2016. Vol. 2016, no. 2016, pp.1-11.
https://search.emarefa.net/detail/BIM-1099597
Data Type
Journal Articles
Language
English
Notes
Includes bibliographical references
Record ID
BIM-1099597