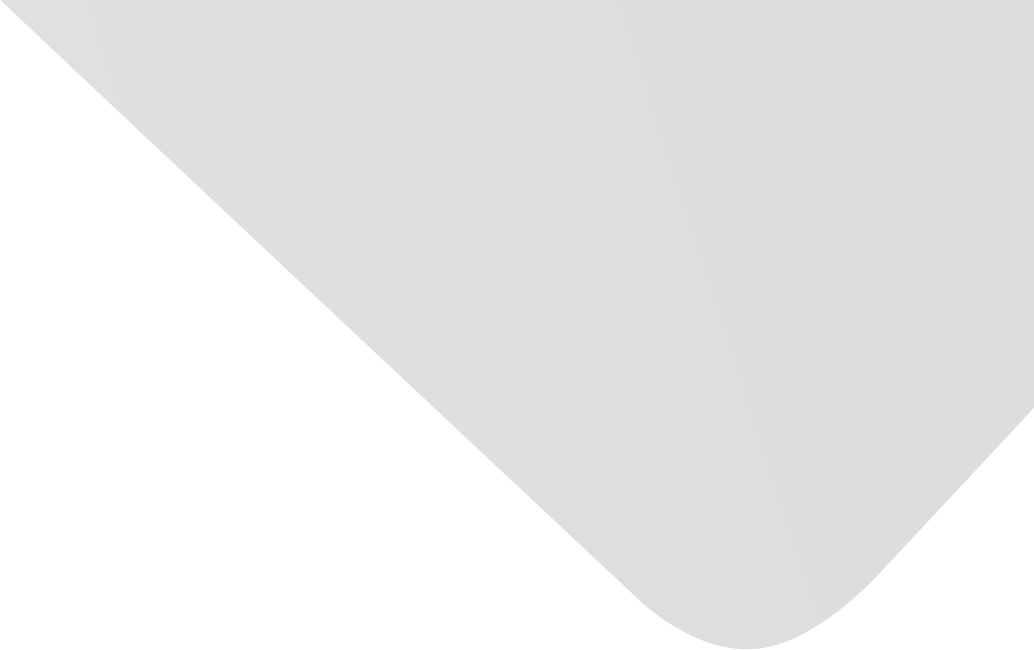
Visual Tracking Based on an Improved Online Multiple Instance Learning Algorithm
Joint Authors
Source
Computational Intelligence and Neuroscience
Issue
Vol. 2016, Issue 2016 (31 Dec. 2015), pp.1-9, 9 p.
Publisher
Hindawi Publishing Corporation
Publication Date
2015-12-30
Country of Publication
Egypt
No. of Pages
9
Main Subjects
Abstract EN
An improved online multiple instance learning (IMIL) for a visual tracking algorithm is proposed.
In the IMIL algorithm, the importance of each instance contributing to a bag probability is with respect to their probabilities.
A selection strategy based on an inner product is presented to choose weak classifier from a classifier pool, which avoids computing instance probabilities and bag probability M times.
Furthermore, a feedback strategy is presented to update weak classifiers.
In the feedback update strategy, different weights are assigned to the tracking result and template according to the maximum classifier score.
Finally, the presented algorithm is compared with other state-of-the-art algorithms.
The experimental results demonstrate that the proposed tracking algorithm runs in real-time and is robust to occlusion and appearance changes.
American Psychological Association (APA)
Wang, Li Jia& Zhang, Hua. 2015. Visual Tracking Based on an Improved Online Multiple Instance Learning Algorithm. Computational Intelligence and Neuroscience،Vol. 2016, no. 2016, pp.1-9.
https://search.emarefa.net/detail/BIM-1099657
Modern Language Association (MLA)
Wang, Li Jia& Zhang, Hua. Visual Tracking Based on an Improved Online Multiple Instance Learning Algorithm. Computational Intelligence and Neuroscience Vol. 2016, no. 2016 (2015), pp.1-9.
https://search.emarefa.net/detail/BIM-1099657
American Medical Association (AMA)
Wang, Li Jia& Zhang, Hua. Visual Tracking Based on an Improved Online Multiple Instance Learning Algorithm. Computational Intelligence and Neuroscience. 2015. Vol. 2016, no. 2016, pp.1-9.
https://search.emarefa.net/detail/BIM-1099657
Data Type
Journal Articles
Language
English
Notes
Includes bibliographical references
Record ID
BIM-1099657