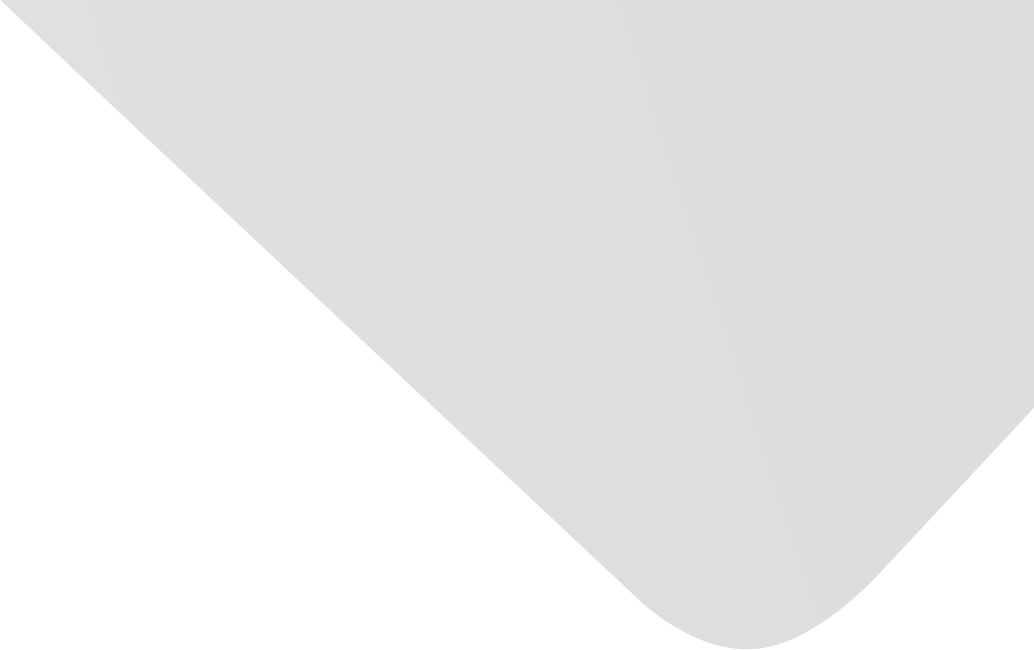
Efficient Actor-Critic Algorithm with Hierarchical Model Learning and Planning
Joint Authors
Liu, Quan
Zhong, Shan
Fu, QiMing
Source
Computational Intelligence and Neuroscience
Issue
Vol. 2016, Issue 2016 (31 Dec. 2015), pp.1-15, 15 p.
Publisher
Hindawi Publishing Corporation
Publication Date
2016-10-03
Country of Publication
Egypt
No. of Pages
15
Main Subjects
Abstract EN
To improve the convergence rate and the sample efficiency, two efficient learning methods AC-HMLP and RAC-HMLP (AC-HMLP with l 2 -regularization) are proposed by combining actor-critic algorithm with hierarchical model learning and planning.
The hierarchical models consisting of the local and the global models, which are learned at the same time during learning of the value function and the policy, are approximated by local linear regression (LLR) and linear function approximation (LFA), respectively.
Both the local model and the global model are applied to generate samples for planning; the former is used only if the state-prediction error does not surpass the threshold at each time step, while the latter is utilized at the end of each episode.
The purpose of taking both models is to improve the sample efficiency and accelerate the convergence rate of the whole algorithm through fully utilizing the local and global information.
Experimentally, AC-HMLP and RAC-HMLP are compared with three representative algorithms on two Reinforcement Learning (RL) benchmark problems.
The results demonstrate that they perform best in terms of convergence rate and sample efficiency.
American Psychological Association (APA)
Zhong, Shan& Liu, Quan& Fu, QiMing. 2016. Efficient Actor-Critic Algorithm with Hierarchical Model Learning and Planning. Computational Intelligence and Neuroscience،Vol. 2016, no. 2016, pp.1-15.
https://search.emarefa.net/detail/BIM-1099688
Modern Language Association (MLA)
Zhong, Shan…[et al.]. Efficient Actor-Critic Algorithm with Hierarchical Model Learning and Planning. Computational Intelligence and Neuroscience Vol. 2016, no. 2016 (2015), pp.1-15.
https://search.emarefa.net/detail/BIM-1099688
American Medical Association (AMA)
Zhong, Shan& Liu, Quan& Fu, QiMing. Efficient Actor-Critic Algorithm with Hierarchical Model Learning and Planning. Computational Intelligence and Neuroscience. 2016. Vol. 2016, no. 2016, pp.1-15.
https://search.emarefa.net/detail/BIM-1099688
Data Type
Journal Articles
Language
English
Notes
Includes bibliographical references
Record ID
BIM-1099688