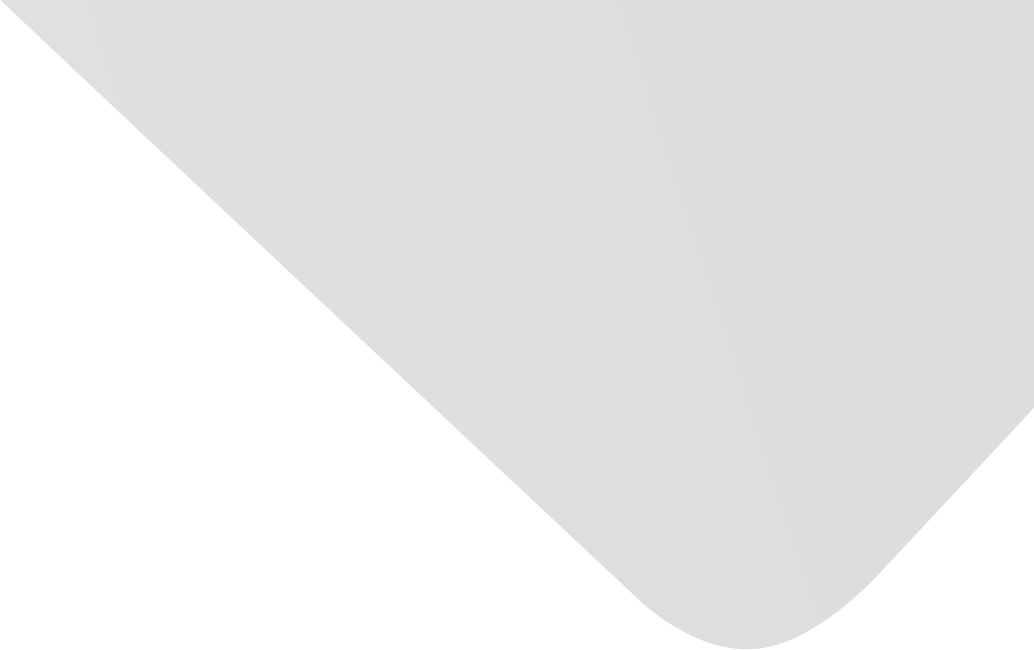
Ensemble Deep Learning for Biomedical Time Series Classification
Joint Authors
Source
Computational Intelligence and Neuroscience
Issue
Vol. 2016, Issue 2016 (31 Dec. 2015), pp.1-13, 13 p.
Publisher
Hindawi Publishing Corporation
Publication Date
2016-09-20
Country of Publication
Egypt
No. of Pages
13
Main Subjects
Abstract EN
Ensemble learning has been proved to improve the generalization ability effectively in both theory and practice.
In this paper, we briefly outline the current status of research on it first.
Then, a new deep neural network-based ensemble method that integrates filtering views, local views, distorted views, explicit training, implicit training, subview prediction, and Simple Average is proposed for biomedical time series classification.
Finally, we validate its effectiveness on the Chinese Cardiovascular Disease Database containing a large number of electrocardiogram recordings.
The experimental results show that the proposed method has certain advantages compared to some well-known ensemble methods, such as Bagging and AdaBoost.
American Psychological Association (APA)
Jin, Lin-peng& Dong, Jun. 2016. Ensemble Deep Learning for Biomedical Time Series Classification. Computational Intelligence and Neuroscience،Vol. 2016, no. 2016, pp.1-13.
https://search.emarefa.net/detail/BIM-1099725
Modern Language Association (MLA)
Jin, Lin-peng& Dong, Jun. Ensemble Deep Learning for Biomedical Time Series Classification. Computational Intelligence and Neuroscience Vol. 2016, no. 2016 (2015), pp.1-13.
https://search.emarefa.net/detail/BIM-1099725
American Medical Association (AMA)
Jin, Lin-peng& Dong, Jun. Ensemble Deep Learning for Biomedical Time Series Classification. Computational Intelligence and Neuroscience. 2016. Vol. 2016, no. 2016, pp.1-13.
https://search.emarefa.net/detail/BIM-1099725
Data Type
Journal Articles
Language
English
Notes
Includes bibliographical references
Record ID
BIM-1099725