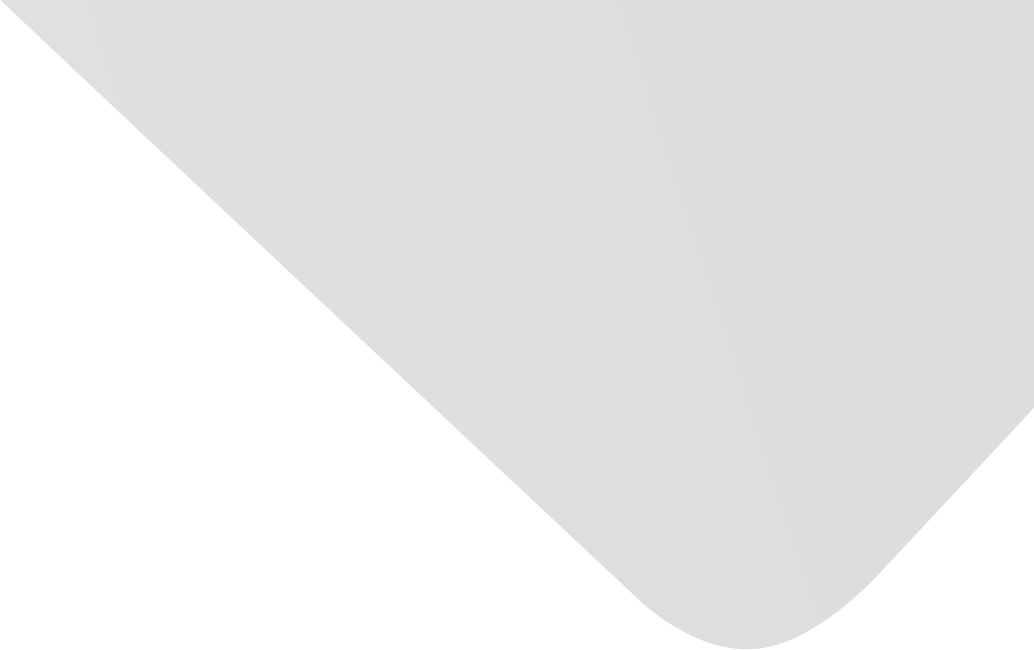
Human Action Recognition Using Improved Salient Dense Trajectories
Joint Authors
Li, Qingwu
Cheng, Haisu
Zhou, Yan
Huo, Guanying
Source
Computational Intelligence and Neuroscience
Issue
Vol. 2016, Issue 2016 (31 Dec. 2015), pp.1-11, 11 p.
Publisher
Hindawi Publishing Corporation
Publication Date
2016-05-17
Country of Publication
Egypt
No. of Pages
11
Main Subjects
Abstract EN
Human action recognition in videos is a topic of active research in computer vision.
Dense trajectory (DT) features were shown to be efficient for representing videos in state-of-the-art approaches.
In this paper, we present a more effective approach of video representation using improved salient dense trajectories: first, detecting the motion salient region and extracting the dense trajectories by tracking interest points in each spatial scale separately and then refining the dense trajectories via the analysis of the motion saliency.
Then, we compute several descriptors (i.e., trajectory displacement, HOG, HOF, and MBH) in the spatiotemporal volume aligned with the trajectories.
Finally, in order to represent the videos better, we optimize the framework of bag-of-words according to the motion salient intensity distribution and the idea of sparse coefficient reconstruction.
Our architecture is trained and evaluated on the four standard video actions datasets of KTH, UCF sports, HMDB51, and UCF50, and the experimental results show that our approach performs competitively comparing with the state-of-the-art results.
American Psychological Association (APA)
Li, Qingwu& Cheng, Haisu& Zhou, Yan& Huo, Guanying. 2016. Human Action Recognition Using Improved Salient Dense Trajectories. Computational Intelligence and Neuroscience،Vol. 2016, no. 2016, pp.1-11.
https://search.emarefa.net/detail/BIM-1099740
Modern Language Association (MLA)
Li, Qingwu…[et al.]. Human Action Recognition Using Improved Salient Dense Trajectories. Computational Intelligence and Neuroscience Vol. 2016, no. 2016 (2015), pp.1-11.
https://search.emarefa.net/detail/BIM-1099740
American Medical Association (AMA)
Li, Qingwu& Cheng, Haisu& Zhou, Yan& Huo, Guanying. Human Action Recognition Using Improved Salient Dense Trajectories. Computational Intelligence and Neuroscience. 2016. Vol. 2016, no. 2016, pp.1-11.
https://search.emarefa.net/detail/BIM-1099740
Data Type
Journal Articles
Language
English
Notes
Includes bibliographical references
Record ID
BIM-1099740