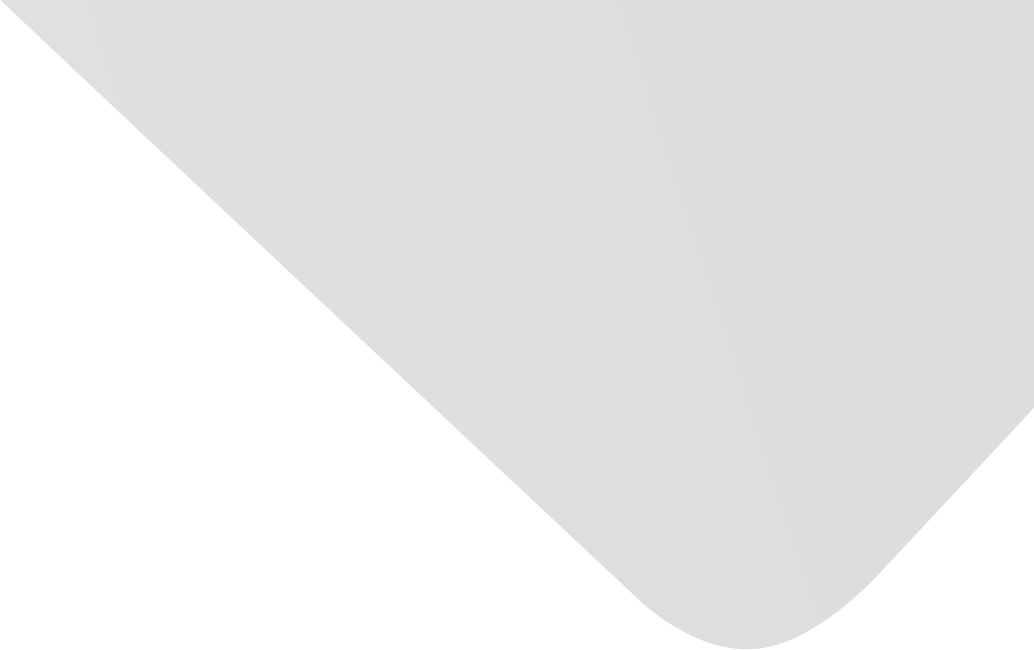
Preference Mining Using Neighborhood Rough Set Model on Two Universes
Author
Source
Computational Intelligence and Neuroscience
Issue
Vol. 2016, Issue 2016 (31 Dec. 2015), pp.1-13, 13 p.
Publisher
Hindawi Publishing Corporation
Publication Date
2016-12-04
Country of Publication
Egypt
No. of Pages
13
Main Subjects
Abstract EN
Preference mining plays an important role in e-commerce and video websites for enhancing user satisfaction and loyalty.
Some classical methods are not available for the cold-start problem when the user or the item is new.
In this paper, we propose a new model, called parametric neighborhood rough set on two universes (NRSTU), to describe the user and item data structures.
Furthermore, the neighborhood lower approximation operator is used for defining the preference rules.
Then, we provide the means for recommending items to users by using these rules.
Finally, we give an experimental example to show the details of NRSTU-based preference mining for cold-start problem.
The parameters of the model are also discussed.
The experimental results show that the proposed method presents an effective solution for preference mining.
In particular, NRSTU improves the recommendation accuracy by about 19% compared to the traditional method.
American Psychological Association (APA)
Zeng, Kai. 2016. Preference Mining Using Neighborhood Rough Set Model on Two Universes. Computational Intelligence and Neuroscience،Vol. 2016, no. 2016, pp.1-13.
https://search.emarefa.net/detail/BIM-1099743
Modern Language Association (MLA)
Zeng, Kai. Preference Mining Using Neighborhood Rough Set Model on Two Universes. Computational Intelligence and Neuroscience Vol. 2016, no. 2016 (2015), pp.1-13.
https://search.emarefa.net/detail/BIM-1099743
American Medical Association (AMA)
Zeng, Kai. Preference Mining Using Neighborhood Rough Set Model on Two Universes. Computational Intelligence and Neuroscience. 2016. Vol. 2016, no. 2016, pp.1-13.
https://search.emarefa.net/detail/BIM-1099743
Data Type
Journal Articles
Language
English
Notes
Includes bibliographical references
Record ID
BIM-1099743