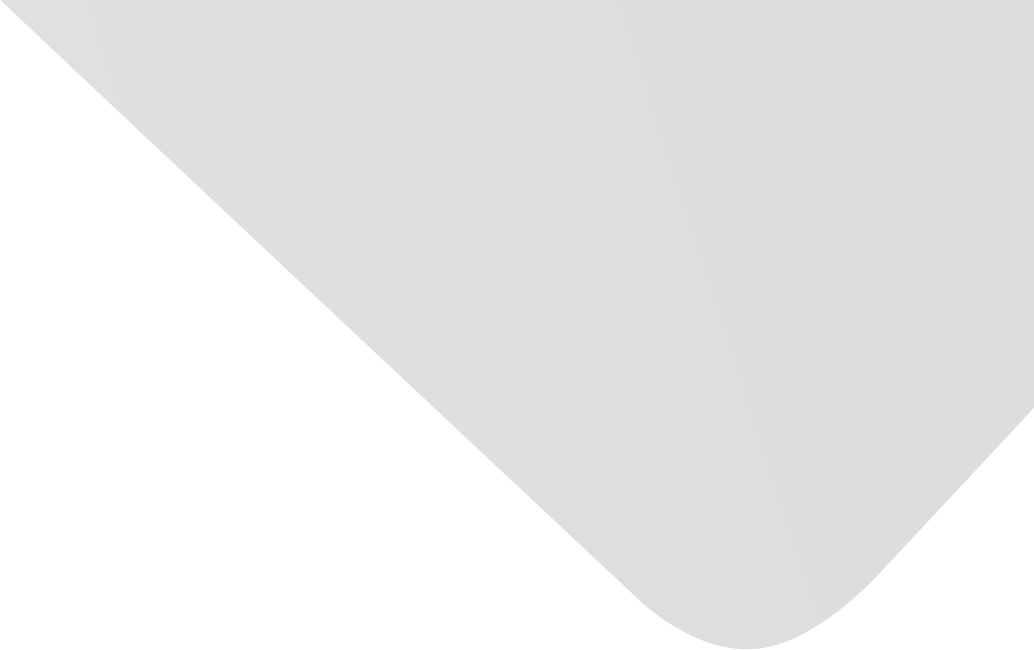
Aero Engine Component Fault Diagnosis Using Multi-Hidden-Layer Extreme Learning Machine with Optimized Structure
Joint Authors
Yang, Xinyi
Zhang, Xiaofeng
Pang, Shan
Source
International Journal of Aerospace Engineering
Issue
Vol. 2016, Issue 2016 (31 Dec. 2016), pp.1-11, 11 p.
Publisher
Hindawi Publishing Corporation
Publication Date
2016-08-23
Country of Publication
Egypt
No. of Pages
11
Abstract EN
A new aero gas turbine engine gas path component fault diagnosis method based on multi-hidden-layer extreme learning machine with optimized structure (OM-ELM) was proposed.
OM-ELM employs quantum-behaved particle swarm optimization to automatically obtain the optimal network structure according to both the root mean square error on training data set and the norm of output weights.
The proposed method is applied to handwritten recognition data set and a gas turbine engine diagnostic application and is compared with basic ELM, multi-hidden-layer ELM, and two state-of-the-art deep learning algorithms: deep belief network and the stacked denoising autoencoder.
Results show that, with optimized network structure, OM-ELM obtains better test accuracy in both applications and is more robust to sensor noise.
Meanwhile it controls the model complexity and needs far less hidden nodes than multi-hidden-layer ELM, thus saving computer memory and making it more efficient to implement.
All these advantages make our method an effective and reliable tool for engine component fault diagnosis tool.
American Psychological Association (APA)
Pang, Shan& Yang, Xinyi& Zhang, Xiaofeng. 2016. Aero Engine Component Fault Diagnosis Using Multi-Hidden-Layer Extreme Learning Machine with Optimized Structure. International Journal of Aerospace Engineering،Vol. 2016, no. 2016, pp.1-11.
https://search.emarefa.net/detail/BIM-1104971
Modern Language Association (MLA)
Pang, Shan…[et al.]. Aero Engine Component Fault Diagnosis Using Multi-Hidden-Layer Extreme Learning Machine with Optimized Structure. International Journal of Aerospace Engineering No. 2016 (2016), pp.1-11.
https://search.emarefa.net/detail/BIM-1104971
American Medical Association (AMA)
Pang, Shan& Yang, Xinyi& Zhang, Xiaofeng. Aero Engine Component Fault Diagnosis Using Multi-Hidden-Layer Extreme Learning Machine with Optimized Structure. International Journal of Aerospace Engineering. 2016. Vol. 2016, no. 2016, pp.1-11.
https://search.emarefa.net/detail/BIM-1104971
Data Type
Journal Articles
Language
English
Notes
Includes bibliographical references
Record ID
BIM-1104971