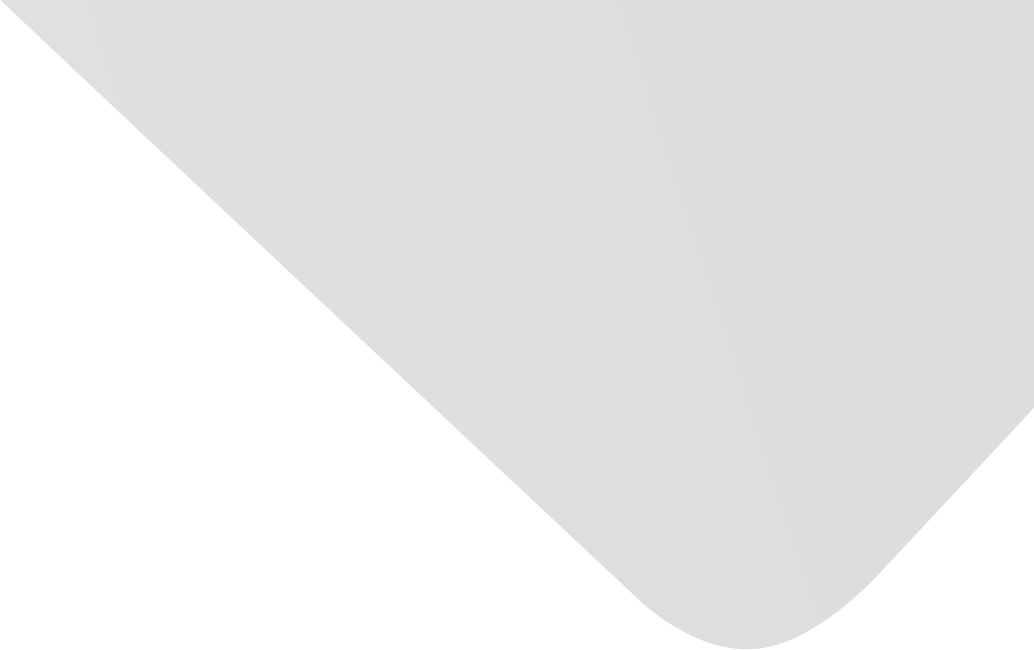
SVM Intrusion Detection Model Based on Compressed Sampling
Joint Authors
Chen, Shanxiong
Peng, Maoling
Xiong, Hailing
Yu, Xianping
Source
Journal of Electrical and Computer Engineering
Issue
Vol. 2016, Issue 2016 (31 Dec. 2016), pp.1-6, 6 p.
Publisher
Hindawi Publishing Corporation
Publication Date
2016-03-28
Country of Publication
Egypt
No. of Pages
6
Main Subjects
Information Technology and Computer Science
Abstract EN
Intrusion detection needs to deal with a large amount of data; particularly, the technology of network intrusion detection has to detect all of network data.
Massive data processing is the bottleneck of network software and hardware equipment in intrusion detection.
If we can reduce the data dimension in the stage of data sampling and directly obtain the feature information of network data, efficiency of detection can be improved greatly.
In the paper, we present a SVM intrusion detection model based on compressive sampling.
We use compressed sampling method in the compressed sensing theory to implement feature compression for network data flow so that we can gain refined sparse representation.
After that SVM is used to classify the compression results.
This method can realize detection of network anomaly behavior quickly without reducing the classification accuracy.
American Psychological Association (APA)
Chen, Shanxiong& Peng, Maoling& Xiong, Hailing& Yu, Xianping. 2016. SVM Intrusion Detection Model Based on Compressed Sampling. Journal of Electrical and Computer Engineering،Vol. 2016, no. 2016, pp.1-6.
https://search.emarefa.net/detail/BIM-1108426
Modern Language Association (MLA)
Chen, Shanxiong…[et al.]. SVM Intrusion Detection Model Based on Compressed Sampling. Journal of Electrical and Computer Engineering No. 2016 (2016), pp.1-6.
https://search.emarefa.net/detail/BIM-1108426
American Medical Association (AMA)
Chen, Shanxiong& Peng, Maoling& Xiong, Hailing& Yu, Xianping. SVM Intrusion Detection Model Based on Compressed Sampling. Journal of Electrical and Computer Engineering. 2016. Vol. 2016, no. 2016, pp.1-6.
https://search.emarefa.net/detail/BIM-1108426
Data Type
Journal Articles
Language
English
Notes
Includes bibliographical references
Record ID
BIM-1108426