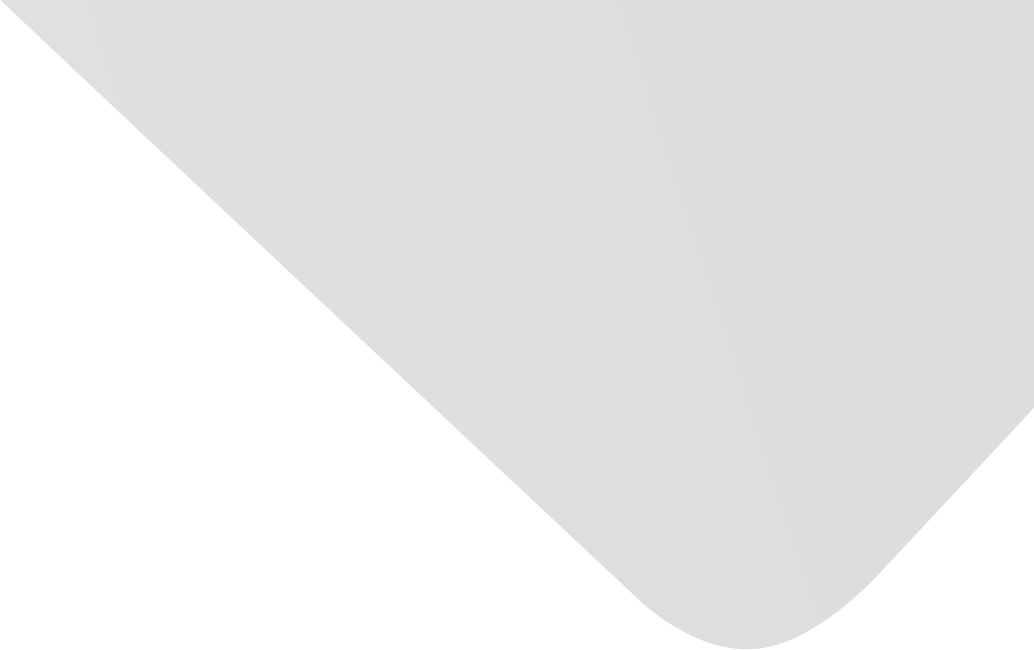
Deep Learning for Hyperspectral Data Classification through Exponential Momentum Deep Convolution Neural Networks
Joint Authors
Source
Issue
Vol. 2016, Issue 2016 (31 Dec. 2016), pp.1-8, 8 p.
Publisher
Hindawi Publishing Corporation
Publication Date
2016-10-25
Country of Publication
Egypt
No. of Pages
8
Main Subjects
Abstract EN
Classification is a hot topic in hyperspectral remote sensing community.
In the last decades, numerous efforts have been concentrated on the classification problem.
Most of the existing studies and research efforts are following the conventional pattern recognition paradigm, which is based on complex handcrafted features.
However, it is rarely known which features are important for the problem.
In this paper, a new classification skeleton based on deep machine learning is proposed for hyperspectral data.
The proposed classification framework, which is composed of exponential momentum deep convolution neural network and support vector machine (SVM), can hierarchically construct high-level spectral-spatial features in an automated way.
Experimental results and quantitative validation on widely used datasets showcase the potential of the developed approach for accurate hyperspectral data classification.
American Psychological Association (APA)
Yue, Qi& Ma, Caiwen. 2016. Deep Learning for Hyperspectral Data Classification through Exponential Momentum Deep Convolution Neural Networks. Journal of Sensors،Vol. 2016, no. 2016, pp.1-8.
https://search.emarefa.net/detail/BIM-1110409
Modern Language Association (MLA)
Yue, Qi& Ma, Caiwen. Deep Learning for Hyperspectral Data Classification through Exponential Momentum Deep Convolution Neural Networks. Journal of Sensors No. 2016 (2016), pp.1-8.
https://search.emarefa.net/detail/BIM-1110409
American Medical Association (AMA)
Yue, Qi& Ma, Caiwen. Deep Learning for Hyperspectral Data Classification through Exponential Momentum Deep Convolution Neural Networks. Journal of Sensors. 2016. Vol. 2016, no. 2016, pp.1-8.
https://search.emarefa.net/detail/BIM-1110409
Data Type
Journal Articles
Language
English
Notes
Includes bibliographical references
Record ID
BIM-1110409