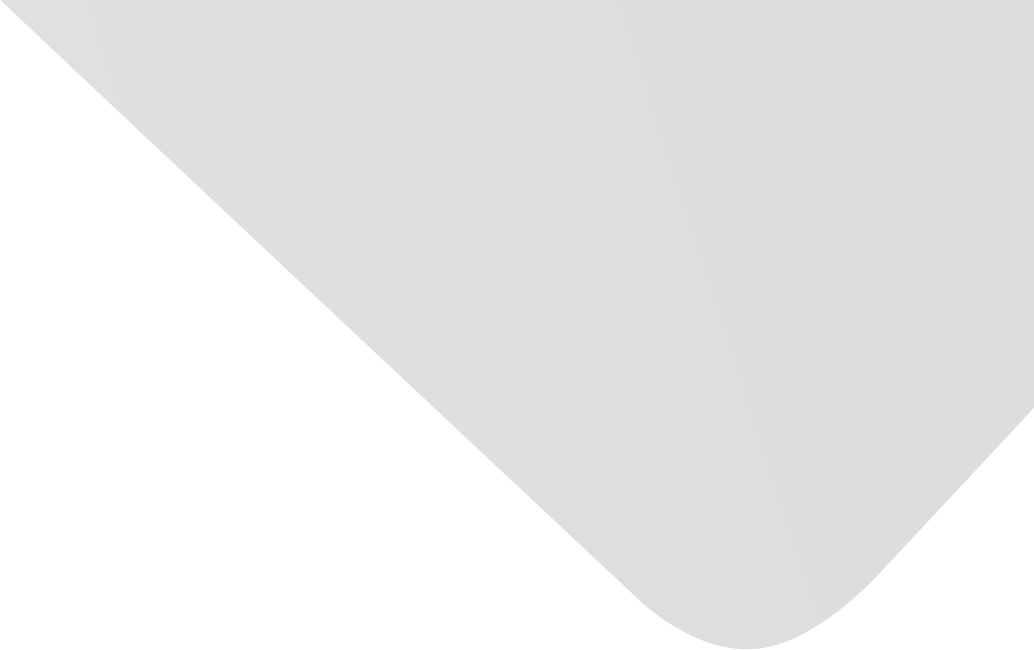
A High-Order CFS Algorithm for Clustering Big Data
Joint Authors
Bu, Fanyu
Li, Peng
Tang, Tong
Zhang, Ying
Chen, Zhikui
Source
Issue
Vol. 2016, Issue 2016 (31 Dec. 2016), pp.1-8, 8 p.
Publisher
Hindawi Publishing Corporation
Publication Date
2016-07-25
Country of Publication
Egypt
No. of Pages
8
Main Subjects
Telecommunications Engineering
Abstract EN
With the development of Internet of Everything such as Internet of Things, Internet of People, and Industrial Internet, big data is being generated.
Clustering is a widely used technique for big data analytics and mining.
However, most of current algorithms are not effective to cluster heterogeneous data which is prevalent in big data.
In this paper, we propose a high-order CFS algorithm (HOCFS) to cluster heterogeneous data by combining the CFS clustering algorithm and the dropout deep learning model, whose functionality rests on three pillars: (i) an adaptive dropout deep learning model to learn features from each type of data, (ii) a feature tensor model to capture the correlations of heterogeneous data, and (iii) a tensor distance-based high-order CFS algorithm to cluster heterogeneous data.
Furthermore, we verify our proposed algorithm on different datasets, by comparison with other two clustering schemes, that is, HOPCM and CFS.
Results confirm the effectiveness of the proposed algorithm in clustering heterogeneous data.
American Psychological Association (APA)
Bu, Fanyu& Chen, Zhikui& Li, Peng& Tang, Tong& Zhang, Ying. 2016. A High-Order CFS Algorithm for Clustering Big Data. Mobile Information Systems،Vol. 2016, no. 2016, pp.1-8.
https://search.emarefa.net/detail/BIM-1111456
Modern Language Association (MLA)
Bu, Fanyu…[et al.]. A High-Order CFS Algorithm for Clustering Big Data. Mobile Information Systems No. 2016 (2016), pp.1-8.
https://search.emarefa.net/detail/BIM-1111456
American Medical Association (AMA)
Bu, Fanyu& Chen, Zhikui& Li, Peng& Tang, Tong& Zhang, Ying. A High-Order CFS Algorithm for Clustering Big Data. Mobile Information Systems. 2016. Vol. 2016, no. 2016, pp.1-8.
https://search.emarefa.net/detail/BIM-1111456
Data Type
Journal Articles
Language
English
Notes
Includes bibliographical references
Record ID
BIM-1111456