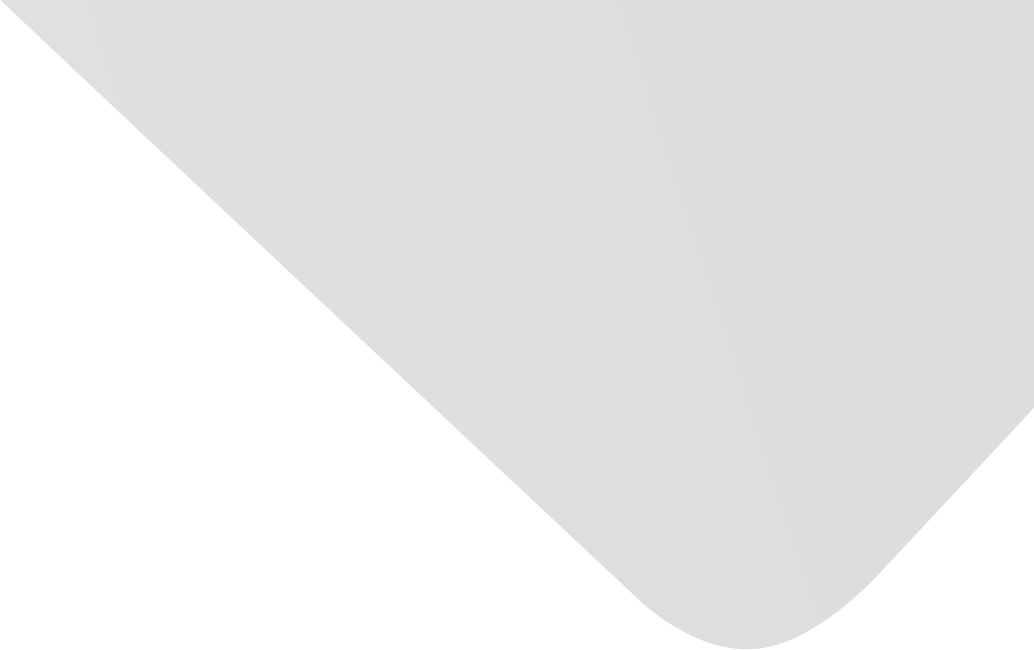
Adaptive Loss Inference Using Unicast End-to-End Measurements
Joint Authors
Source
Mathematical Problems in Engineering
Issue
Vol. 2016, Issue 2016 (31 Dec. 2016), pp.1-11, 11 p.
Publisher
Hindawi Publishing Corporation
Publication Date
2016-12-20
Country of Publication
Egypt
No. of Pages
11
Main Subjects
Abstract EN
We address the problem of inferring link loss rates from unicast end-to-end measurements on the basis of network tomography.
Because measurement probes will incur additional traffic overheads, most tomography-based approaches perform the inference by collecting the measurements only on selected paths to reduce the overhead.
However, all previous approaches select paths offline, which will inevitably miss many potential identifiable links, whose loss rates should be unbiasedly determined.
Furthermore, if element failures exist, an appreciable number of the selected paths may become unavailable.
In this paper, we creatively propose an adaptive loss inference approach in which the paths are selected sequentially depending on the previous measurement results.
In each round, we compute the loss rates of links that can be unbiasedly determined based on the current measurement results and remove them from the system.
Meanwhile, we locate the most possible failures based on the current measurement outcomes to avoid selecting unavailable paths in subsequent rounds.
In this way, all identifiable and potential identifiable links can be determined unbiasedly using only 20% of all available end-to-end measurements.
Compared with a previous classical approach through extensive simulations, the results strongly confirm the promising performance of our proposed approach.
American Psychological Association (APA)
Qiao, Yan& Jiao, Jun& Ma, Huimin. 2016. Adaptive Loss Inference Using Unicast End-to-End Measurements. Mathematical Problems in Engineering،Vol. 2016, no. 2016, pp.1-11.
https://search.emarefa.net/detail/BIM-1111813
Modern Language Association (MLA)
Qiao, Yan…[et al.]. Adaptive Loss Inference Using Unicast End-to-End Measurements. Mathematical Problems in Engineering No. 2016 (2016), pp.1-11.
https://search.emarefa.net/detail/BIM-1111813
American Medical Association (AMA)
Qiao, Yan& Jiao, Jun& Ma, Huimin. Adaptive Loss Inference Using Unicast End-to-End Measurements. Mathematical Problems in Engineering. 2016. Vol. 2016, no. 2016, pp.1-11.
https://search.emarefa.net/detail/BIM-1111813
Data Type
Journal Articles
Language
English
Notes
Includes bibliographical references
Record ID
BIM-1111813