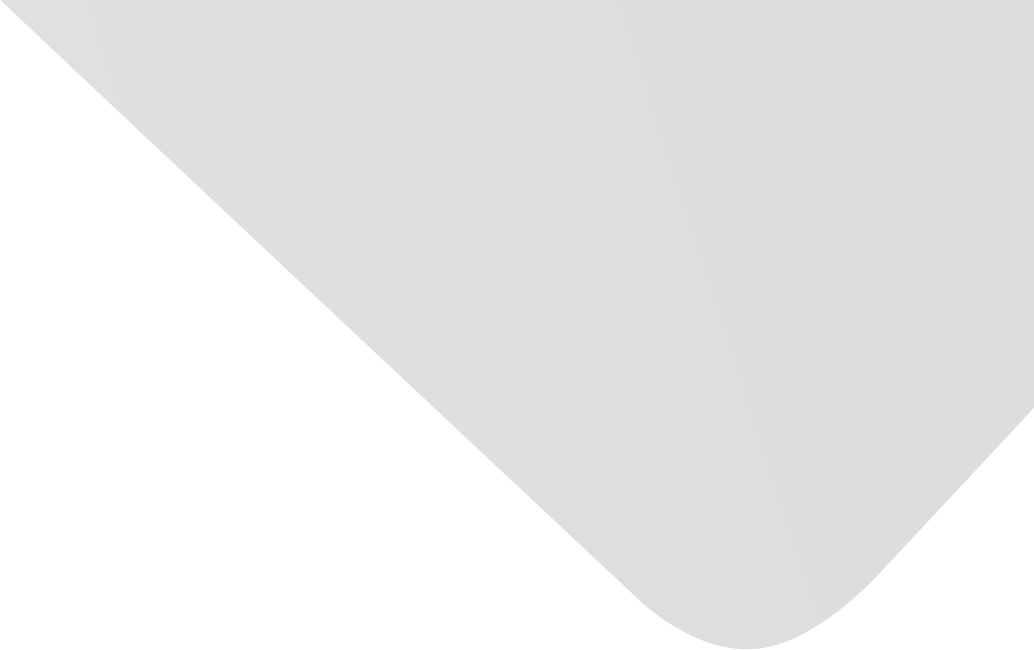
Conditional Random Fields for Image Labeling
Joint Authors
Liu, Tong
Huang, Xiutian
Ma, Jianshe
Source
Mathematical Problems in Engineering
Issue
Vol. 2016, Issue 2016 (31 Dec. 2016), pp.1-15, 15 p.
Publisher
Hindawi Publishing Corporation
Publication Date
2016-04-24
Country of Publication
Egypt
No. of Pages
15
Main Subjects
Abstract EN
With the rapid development and application of CRFs (Conditional Random Fields) in computer vision, many researchers have made some outstanding progress in this domain because CRFs solve the classical version of the label bias problem with respect to MEMMs (maximum entropy Markov models) and HMMs (hidden Markov models).
This paper reviews the research development and status of object recognition with CRFs and especially introduces two main discrete optimization methods for image labeling with CRFs: graph cut and mean field approximation.
This paper describes graph cut briefly while it introduces mean field approximation more detailedly which has a substantial speed of inference and is researched popularly in recent years.
American Psychological Association (APA)
Liu, Tong& Huang, Xiutian& Ma, Jianshe. 2016. Conditional Random Fields for Image Labeling. Mathematical Problems in Engineering،Vol. 2016, no. 2016, pp.1-15.
https://search.emarefa.net/detail/BIM-1112099
Modern Language Association (MLA)
Liu, Tong…[et al.]. Conditional Random Fields for Image Labeling. Mathematical Problems in Engineering No. 2016 (2016), pp.1-15.
https://search.emarefa.net/detail/BIM-1112099
American Medical Association (AMA)
Liu, Tong& Huang, Xiutian& Ma, Jianshe. Conditional Random Fields for Image Labeling. Mathematical Problems in Engineering. 2016. Vol. 2016, no. 2016, pp.1-15.
https://search.emarefa.net/detail/BIM-1112099
Data Type
Journal Articles
Language
English
Notes
Includes bibliographical references
Record ID
BIM-1112099