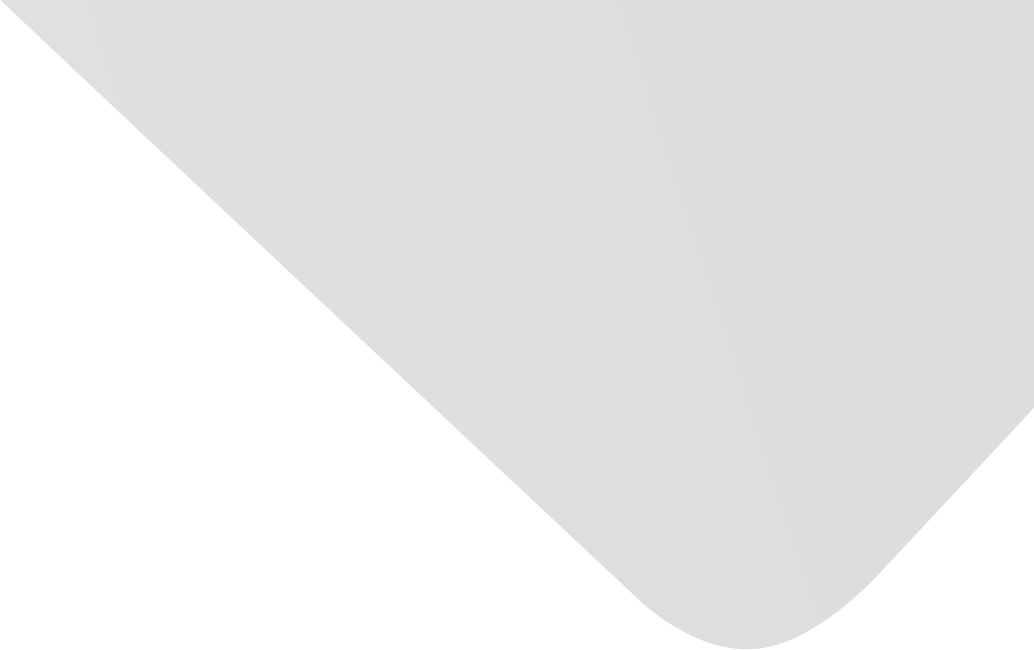
Robust Structure Preserving Nonnegative Matrix Factorization for Dimensionality Reduction
Joint Authors
Han, Zhi
Tang, Yandong
Li, Bingfeng
Source
Mathematical Problems in Engineering
Issue
Vol. 2016, Issue 2016 (31 Dec. 2016), pp.1-14, 14 p.
Publisher
Hindawi Publishing Corporation
Publication Date
2016-06-29
Country of Publication
Egypt
No. of Pages
14
Main Subjects
Abstract EN
As a linear dimensionality reduction method, nonnegative matrix factorization (NMF) has been widely used in many fields, such as machine learning and data mining.
However, there are still two major drawbacks for NMF: (a) NMF can only perform semantic factorization in Euclidean space, and it fails to discover the intrinsic geometrical structure of high-dimensional data distribution.
(b) NMF suffers from noisy data, which are commonly encountered in real-world applications.
To address these issues, in this paper, we present a new robust structure preserving nonnegative matrix factorization (RSPNMF) framework.
In RSPNMF, a local affinity graph and a distant repulsion graph are constructed to encode the geometrical information, and noisy data influence is alleviated by characterizing the data reconstruction term of NMF with l 2,1 -norm instead of l 2 -norm.
With incorporation of the local and distant structure preservation regularization term into the robust NMF framework, our algorithm can discover a low-dimensional embedding subspace with the nature of structure preservation.
RSPNMF is formulated as an optimization problem and solved by an effective iterative multiplicative update algorithm.
Experimental results on some facial image datasets clustering show significant performance improvement of RSPNMF in comparison with the state-of-the-art algorithms.
American Psychological Association (APA)
Li, Bingfeng& Tang, Yandong& Han, Zhi. 2016. Robust Structure Preserving Nonnegative Matrix Factorization for Dimensionality Reduction. Mathematical Problems in Engineering،Vol. 2016, no. 2016, pp.1-14.
https://search.emarefa.net/detail/BIM-1112583
Modern Language Association (MLA)
Li, Bingfeng…[et al.]. Robust Structure Preserving Nonnegative Matrix Factorization for Dimensionality Reduction. Mathematical Problems in Engineering No. 2016 (2016), pp.1-14.
https://search.emarefa.net/detail/BIM-1112583
American Medical Association (AMA)
Li, Bingfeng& Tang, Yandong& Han, Zhi. Robust Structure Preserving Nonnegative Matrix Factorization for Dimensionality Reduction. Mathematical Problems in Engineering. 2016. Vol. 2016, no. 2016, pp.1-14.
https://search.emarefa.net/detail/BIM-1112583
Data Type
Journal Articles
Language
English
Notes
Includes bibliographical references
Record ID
BIM-1112583