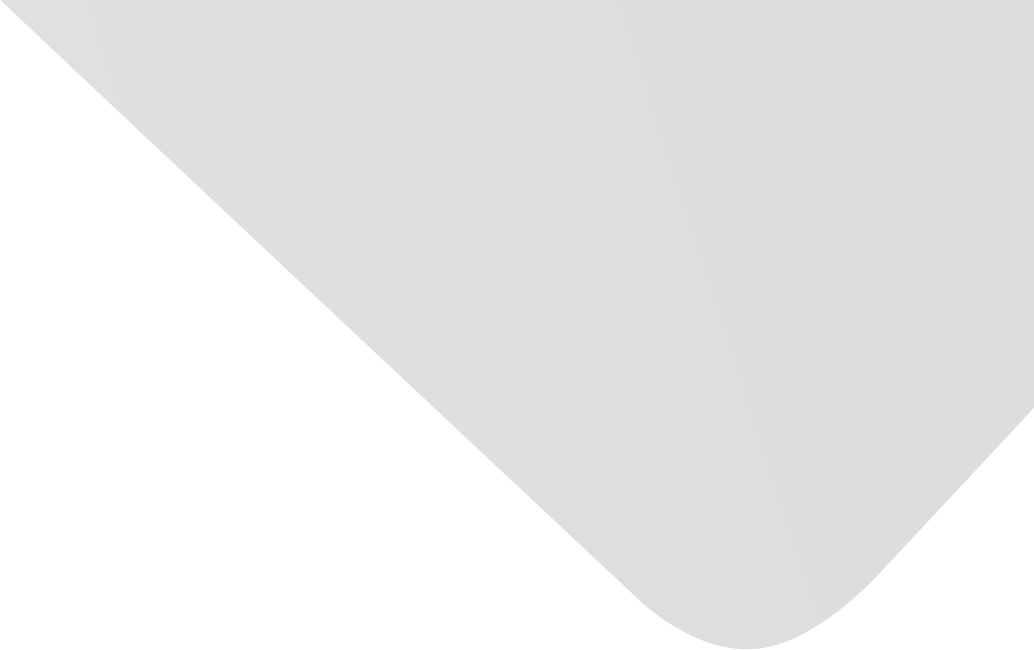
Comparison of Machine Learning Techniques for the Prediction of Compressive Strength of Concrete
Joint Authors
Chopra, Palika
Sharma, Rajendra Kumar
Kumar, Maneek
Chopra, Tanuj
Source
Issue
Vol. 2018, Issue 2018 (31 Dec. 2018), pp.1-9, 9 p.
Publisher
Hindawi Publishing Corporation
Publication Date
2018-04-12
Country of Publication
Egypt
No. of Pages
9
Main Subjects
Abstract EN
A comparative analysis for the prediction of compressive strength of concrete at the ages of 28, 56, and 91 days has been carried out using machine learning techniques via “R” software environment.
R is digging out a strong foothold in the statistical realm and is becoming an indispensable tool for researchers.
The dataset has been generated under controlled laboratory conditions.
Using R miner, the most widely used data mining techniques decision tree (DT) model, random forest (RF) model, and neural network (NN) model have been used and compared with the help of coefficient of determination (R2) and root-mean-square error (RMSE), and it is inferred that the NN model predicts with high accuracy for compressive strength of concrete.
American Psychological Association (APA)
Chopra, Palika& Sharma, Rajendra Kumar& Kumar, Maneek& Chopra, Tanuj. 2018. Comparison of Machine Learning Techniques for the Prediction of Compressive Strength of Concrete. Advances in Civil Engineering،Vol. 2018, no. 2018, pp.1-9.
https://search.emarefa.net/detail/BIM-1116230
Modern Language Association (MLA)
Chopra, Palika…[et al.]. Comparison of Machine Learning Techniques for the Prediction of Compressive Strength of Concrete. Advances in Civil Engineering No. 2018 (2018), pp.1-9.
https://search.emarefa.net/detail/BIM-1116230
American Medical Association (AMA)
Chopra, Palika& Sharma, Rajendra Kumar& Kumar, Maneek& Chopra, Tanuj. Comparison of Machine Learning Techniques for the Prediction of Compressive Strength of Concrete. Advances in Civil Engineering. 2018. Vol. 2018, no. 2018, pp.1-9.
https://search.emarefa.net/detail/BIM-1116230
Data Type
Journal Articles
Language
English
Notes
Includes bibliographical references
Record ID
BIM-1116230