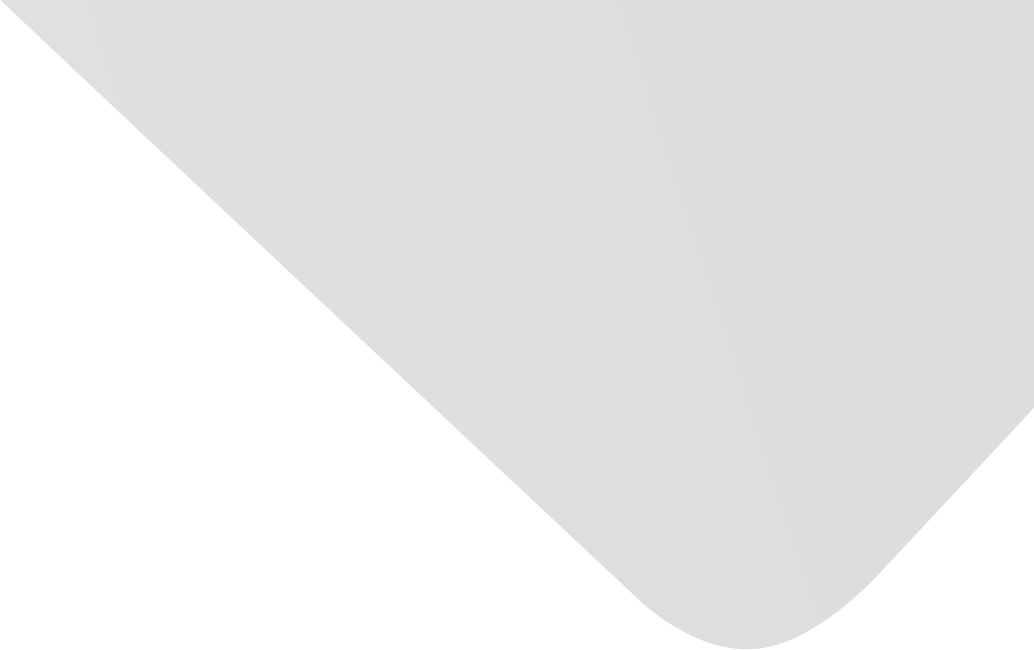
Adjusting Neural Network to a Particular Problem: Neural Network-Based Empirical Biological Model for Chlorophyll Concentration in the Upper Ocean
Joint Authors
Nadiga, Sudhir
Mehra, Avichal
Bayler, Eric
Krasnopolsky, Vladimir M.
Source
Applied Computational Intelligence and Soft Computing
Issue
Vol. 2018, Issue 2018 (31 Dec. 2018), pp.1-10, 10 p.
Publisher
Hindawi Publishing Corporation
Publication Date
2018-11-01
Country of Publication
Egypt
No. of Pages
10
Main Subjects
Information Technology and Computer Science
Abstract EN
The versatility of the neural network (NN) technique allows it to be successfully applied in many fields of science and to a great variety of problems.
For each problem or class of problems, a generic NN technique (e.g., multilayer perceptron (MLP)) usually requires some adjustments, which often are crucial for the development of a successful application.
In this paper, we introduce a NN application that demonstrates the importance of such adjustments; moreover, in this case, the adjustments applied to a generic NN technique may be successfully used in many other NN applications.
We introduce a NN technique, linking chlorophyll “a” (chl-a) variability—primarily driven by biological processes—with the physical processes of the upper ocean using a NN-based empirical biological model for chl-a.
In this study, satellite-derived surface parameter fields, sea-surface temperature (SST) and sea-surface height (SSH), as well as gridded salinity and temperature profiles from 0 to 75m depth are employed as signatures of upper-ocean dynamics.
Chlorophyll-a fields from NOAA’s operational Visible Imaging Infrared Radiometer Suite (VIIRS) are used, as well as Moderate Resolution Imaging Spectroradiometer (MODIS) and Sea-Viewing Wide Field-of-View Sensor (SeaWiFS) chl-a concentrations.
Different methods of optimizing the NN technique are investigated.
Results are assessed using the root-mean-square error (RMSE) metric and cross-correlations between observed ocean color (OC) fields and NN output.
To reduce the impact of noise in the data and to obtain a stable computation of the NN Jacobian, an ensemble of NN with different weights is constructed.
This study demonstrates that the NN technique provides an accurate, computationally cheap method to generate long (up to 10 years) time series of consistent chl-a concentration that are in good agreement with chl-a data observed by different satellite sensors during the relevant period.
The presented NN demonstrates a very good ability to generalize in terms of both space and time.
Consequently, the NN-based empirical biological model for chl-a can be used in oceanic models, coupled climate prediction systems, and data assimilation systems to dynamically consider biological processes in the upper ocean.
American Psychological Association (APA)
Krasnopolsky, Vladimir M.& Nadiga, Sudhir& Mehra, Avichal& Bayler, Eric. 2018. Adjusting Neural Network to a Particular Problem: Neural Network-Based Empirical Biological Model for Chlorophyll Concentration in the Upper Ocean. Applied Computational Intelligence and Soft Computing،Vol. 2018, no. 2018, pp.1-10.
https://search.emarefa.net/detail/BIM-1117055
Modern Language Association (MLA)
Krasnopolsky, Vladimir M.…[et al.]. Adjusting Neural Network to a Particular Problem: Neural Network-Based Empirical Biological Model for Chlorophyll Concentration in the Upper Ocean. Applied Computational Intelligence and Soft Computing No. 2018 (2018), pp.1-10.
https://search.emarefa.net/detail/BIM-1117055
American Medical Association (AMA)
Krasnopolsky, Vladimir M.& Nadiga, Sudhir& Mehra, Avichal& Bayler, Eric. Adjusting Neural Network to a Particular Problem: Neural Network-Based Empirical Biological Model for Chlorophyll Concentration in the Upper Ocean. Applied Computational Intelligence and Soft Computing. 2018. Vol. 2018, no. 2018, pp.1-10.
https://search.emarefa.net/detail/BIM-1117055
Data Type
Journal Articles
Language
English
Notes
Includes bibliographical references
Record ID
BIM-1117055