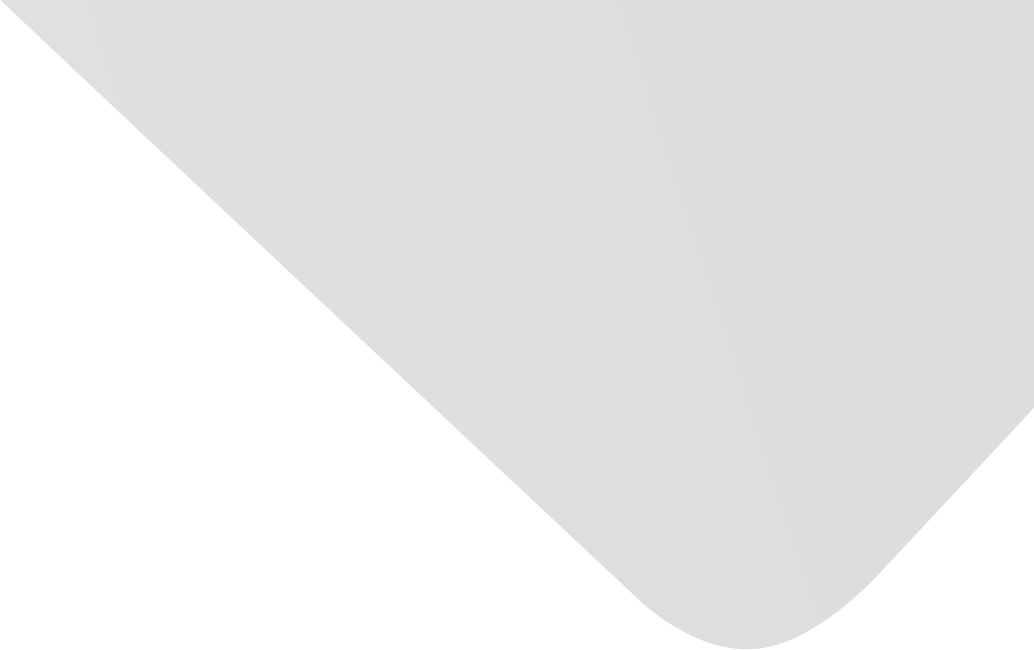
Performance Assessment of Multiple Classifiers Based on Ensemble Feature Selection Scheme for Sentiment Analysis
Joint Authors
Source
Applied Computational Intelligence and Soft Computing
Issue
Vol. 2018, Issue 2018 (31 Dec. 2018), pp.1-12, 12 p.
Publisher
Hindawi Publishing Corporation
Publication Date
2018-10-01
Country of Publication
Egypt
No. of Pages
12
Main Subjects
Information Technology and Computer Science
Abstract EN
Sentiment classification or sentiment analysis has been acknowledged as an open research domain.
In recent years, an enormous research work is being performed in these fields by applying various numbers of methodologies.
Feature generation and selection are consequent for text mining as the high-dimensional feature set can affect the performance of sentiment analysis.
This paper investigates the inability or incompetency of the widely used feature selection methods (IG, Chi-square, and Gini Index) with unigram and bigram feature set on four machine learning classification algorithms (MNB, SVM, KNN, and ME).
The proposed methods are evaluated on the basis of three standard datasets, namely, IMDb movie review and electronics and kitchen product review dataset.
Initially, unigram and bigram features are extracted by applying n-gram method.
In addition, we generate a composite features vector CompUniBi (unigram + bigram), which is sent to the feature selection methods Information Gain (IG), Gini Index (GI), and Chi-square (CHI) to get an optimal feature subset by assigning a score to each of the features.
These methods offer a ranking to the features depending on their score; thus a prominent feature vector (CompIG, CompGI, and CompCHI) can be generated easily for classification.
Finally, the machine learning classifiers SVM, MNB, KNN, and ME used prominent feature vector for classifying the review document into either positive or negative.
The performance of the algorithm is measured by evaluation methods such as precision, recall, and F-measure.
Experimental results show that the composite feature vector achieved a better performance than unigram feature, which is encouraging as well as comparable to the related research.
The best results were obtained from the combination of Information Gain with SVM in terms of highest accuracy.
American Psychological Association (APA)
Ghosh, Monalisa& Sanyal, Goutam. 2018. Performance Assessment of Multiple Classifiers Based on Ensemble Feature Selection Scheme for Sentiment Analysis. Applied Computational Intelligence and Soft Computing،Vol. 2018, no. 2018, pp.1-12.
https://search.emarefa.net/detail/BIM-1117066
Modern Language Association (MLA)
Ghosh, Monalisa& Sanyal, Goutam. Performance Assessment of Multiple Classifiers Based on Ensemble Feature Selection Scheme for Sentiment Analysis. Applied Computational Intelligence and Soft Computing No. 2018 (2018), pp.1-12.
https://search.emarefa.net/detail/BIM-1117066
American Medical Association (AMA)
Ghosh, Monalisa& Sanyal, Goutam. Performance Assessment of Multiple Classifiers Based on Ensemble Feature Selection Scheme for Sentiment Analysis. Applied Computational Intelligence and Soft Computing. 2018. Vol. 2018, no. 2018, pp.1-12.
https://search.emarefa.net/detail/BIM-1117066
Data Type
Journal Articles
Language
English
Notes
Includes bibliographical references
Record ID
BIM-1117066