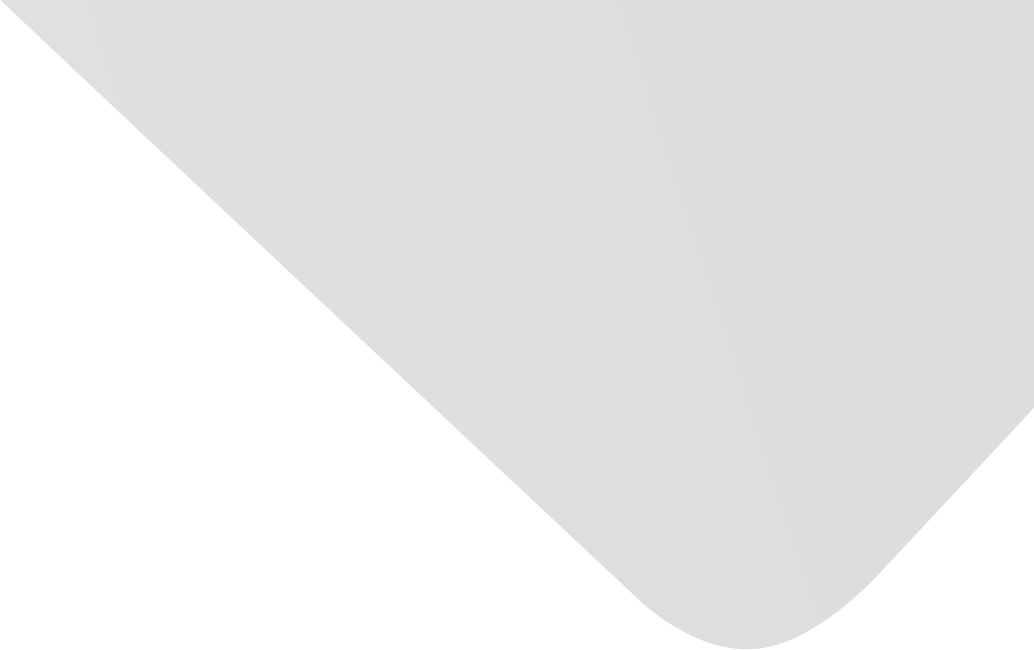
Bearing Fault Diagnosis Using a Novel Classifier Ensemble Based on Lifting Wavelet Packet Transforms and Sample Entropy
Joint Authors
Zhang, Long
Zhang, Lei
Hu, Junfeng
Xiong, Guoliang
Source
Issue
Vol. 2016, Issue 2016 (31 Dec. 2016), pp.1-13, 13 p.
Publisher
Hindawi Publishing Corporation
Publication Date
2016-02-29
Country of Publication
Egypt
No. of Pages
13
Main Subjects
Abstract EN
In order to improve the fault detection accuracy for rolling bearings, an automated fault diagnosis system is presented based on lifting wavelet packet transform (LWPT), sample entropy (SampEn), and classifier ensemble.
Bearing vibration signals are firstly decomposed into different frequency subbands through a three-level LWPT, resulting in a total of 8 frequency-band signals throughout the third layers of the LWPT decomposition tree.
The SampEns of all the 8 components are then calculated as feature vectors.
Such a feature extraction paradigm is expected to depict complexity, irregularity, and nonstationarity of bearing vibrations.
Moreover, a novel classifier ensemble is proposed to alleviate the effect of initial parameters on the performance of member classifiers and to improve classification effectiveness.
Experiments were conducted on electric motor bearings considering various set of fault categories and fault severity levels.
Experimental results demonstrate the proposed diagnosis system can effectively improve bearing fault recognition accuracy and stability in comparison with diagnosis methods based on a single classifier.
American Psychological Association (APA)
Zhang, Lei& Zhang, Long& Hu, Junfeng& Xiong, Guoliang. 2016. Bearing Fault Diagnosis Using a Novel Classifier Ensemble Based on Lifting Wavelet Packet Transforms and Sample Entropy. Shock and Vibration،Vol. 2016, no. 2016, pp.1-13.
https://search.emarefa.net/detail/BIM-1119205
Modern Language Association (MLA)
Zhang, Lei…[et al.]. Bearing Fault Diagnosis Using a Novel Classifier Ensemble Based on Lifting Wavelet Packet Transforms and Sample Entropy. Shock and Vibration No. 2016 (2016), pp.1-13.
https://search.emarefa.net/detail/BIM-1119205
American Medical Association (AMA)
Zhang, Lei& Zhang, Long& Hu, Junfeng& Xiong, Guoliang. Bearing Fault Diagnosis Using a Novel Classifier Ensemble Based on Lifting Wavelet Packet Transforms and Sample Entropy. Shock and Vibration. 2016. Vol. 2016, no. 2016, pp.1-13.
https://search.emarefa.net/detail/BIM-1119205
Data Type
Journal Articles
Language
English
Notes
Includes bibliographical references
Record ID
BIM-1119205