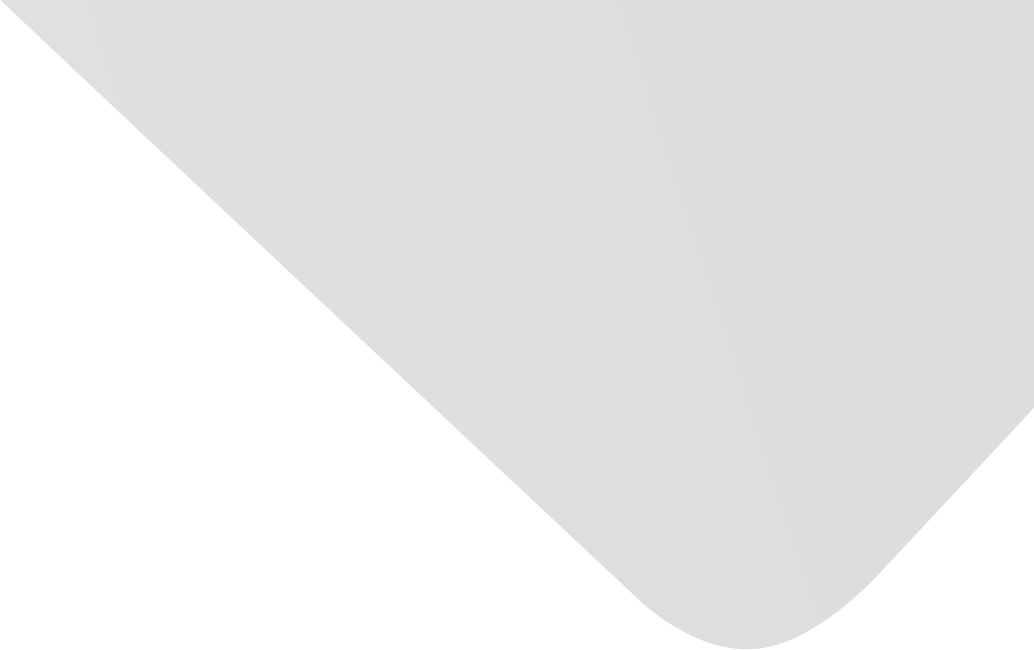
Classification of Continuous Sky Brightness Data Using Random Forest
Joint Authors
Priyatikanto, Rhorom
Mayangsari, Lidia
Prihandoko, Rudi A.
Admiranto, Agustinus G.
Source
Issue
Vol. 2020, Issue 2020 (31 Dec. 2020), pp.1-11, 11 p.
Publisher
Hindawi Publishing Corporation
Publication Date
2020-04-01
Country of Publication
Egypt
No. of Pages
11
Main Subjects
Abstract EN
Sky brightness measuring and monitoring are required to mitigate the negative effect of light pollution as a byproduct of modern civilization.
Good handling of a pile of sky brightness data includes evaluation and classification of the data according to its quality and characteristics such that further analysis and inference can be conducted properly.
This study aims to develop a classification model based on Random Forest algorithm and to evaluate its performance.
Using sky brightness data from 1250 nights with minute temporal resolution acquired at eight different stations in Indonesia, datasets consisting of 15 features were created to train and test the model.
Those features were extracted from the observation time, the global statistics of nightly sky brightness, or the light curve characteristics.
Among those features, 10 are considered to be the most important for the classification task.
The model was trained to classify the data into six classes (1: peculiar data, 2: overcast, 3: cloudy, 4: clear, 5: moonlit-cloudy, and 6: moonlit-clear) and then tested to achieve high accuracy (92%) and scores (F-score = 84% and G-mean = 84%).
Some misclassifications exist, but the classification results are considerably good as indicated by posterior distributions of the sky brightness as a function of classes.
Data classified as class-4 have sharp distribution with typical full width at half maximum of 1.5 mag/arcsec2, while distributions of class-2 and -3 are left skewed with the latter having lighter tail.
Due to the moonlight, distributions of class-5 and -6 data are more smeared or have larger spread.
These results demonstrate that the established classification model is reasonably good and consistent.
American Psychological Association (APA)
Priyatikanto, Rhorom& Mayangsari, Lidia& Prihandoko, Rudi A.& Admiranto, Agustinus G.. 2020. Classification of Continuous Sky Brightness Data Using Random Forest. Advances in Astronomy،Vol. 2020, no. 2020, pp.1-11.
https://search.emarefa.net/detail/BIM-1119779
Modern Language Association (MLA)
Priyatikanto, Rhorom…[et al.]. Classification of Continuous Sky Brightness Data Using Random Forest. Advances in Astronomy No. 2020 (2020), pp.1-11.
https://search.emarefa.net/detail/BIM-1119779
American Medical Association (AMA)
Priyatikanto, Rhorom& Mayangsari, Lidia& Prihandoko, Rudi A.& Admiranto, Agustinus G.. Classification of Continuous Sky Brightness Data Using Random Forest. Advances in Astronomy. 2020. Vol. 2020, no. 2020, pp.1-11.
https://search.emarefa.net/detail/BIM-1119779
Data Type
Journal Articles
Language
English
Notes
Includes bibliographical references
Record ID
BIM-1119779