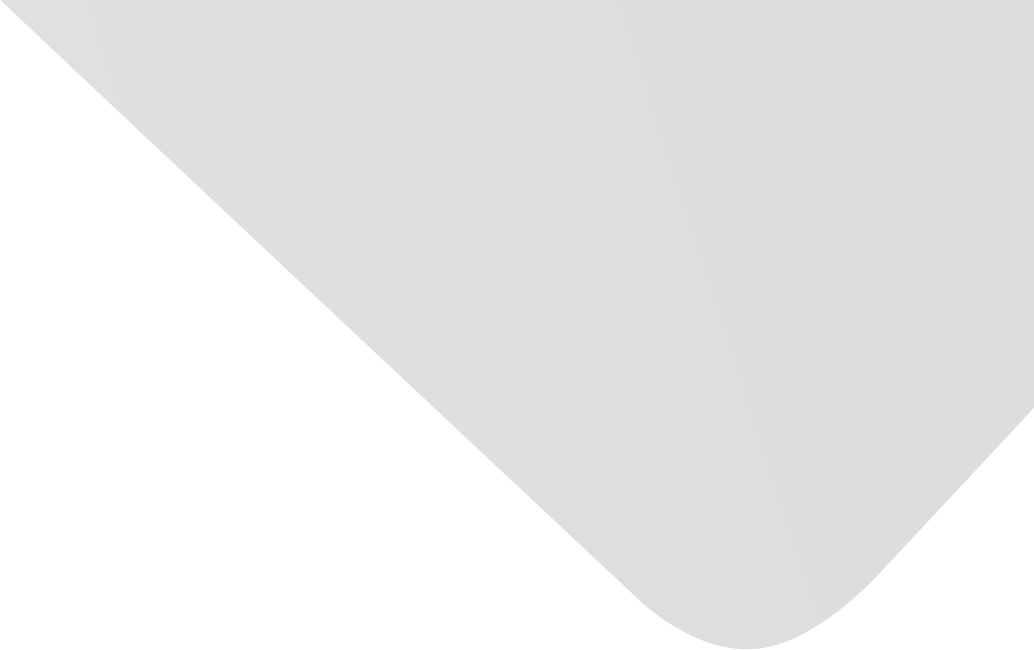
Deep Learning Approach for Estimating Workability of Self-Compacting Concrete from Mixing Image Sequences
Joint Authors
Source
Advances in Materials Science and Engineering
Issue
Vol. 2018, Issue 2018 (31 Dec. 2018), pp.1-16, 16 p.
Publisher
Hindawi Publishing Corporation
Publication Date
2018-11-25
Country of Publication
Egypt
No. of Pages
16
Abstract EN
We propose a deep learning approach to better utilize the spatial and temporal information obtained from image sequences of the self-compacting concrete- (SCC-) mixing process to recover SCC characteristics in terms of the predicted slump flow value (SF) and V-funnel flow time (VF).
The proposed model integrates features of the convolutional neural network and long short-term memory and is trained to extract features and compute an estimate.
The performance of the method is evaluated using the testing set.
The results indicate that the proposed method could potentially be used to automatically estimate SCC workability.
American Psychological Association (APA)
Ding, Zhongcong& An, Xuehui. 2018. Deep Learning Approach for Estimating Workability of Self-Compacting Concrete from Mixing Image Sequences. Advances in Materials Science and Engineering،Vol. 2018, no. 2018, pp.1-16.
https://search.emarefa.net/detail/BIM-1121377
Modern Language Association (MLA)
Ding, Zhongcong& An, Xuehui. Deep Learning Approach for Estimating Workability of Self-Compacting Concrete from Mixing Image Sequences. Advances in Materials Science and Engineering No. 2018 (2018), pp.1-16.
https://search.emarefa.net/detail/BIM-1121377
American Medical Association (AMA)
Ding, Zhongcong& An, Xuehui. Deep Learning Approach for Estimating Workability of Self-Compacting Concrete from Mixing Image Sequences. Advances in Materials Science and Engineering. 2018. Vol. 2018, no. 2018, pp.1-16.
https://search.emarefa.net/detail/BIM-1121377
Data Type
Journal Articles
Language
English
Notes
Includes bibliographical references
Record ID
BIM-1121377