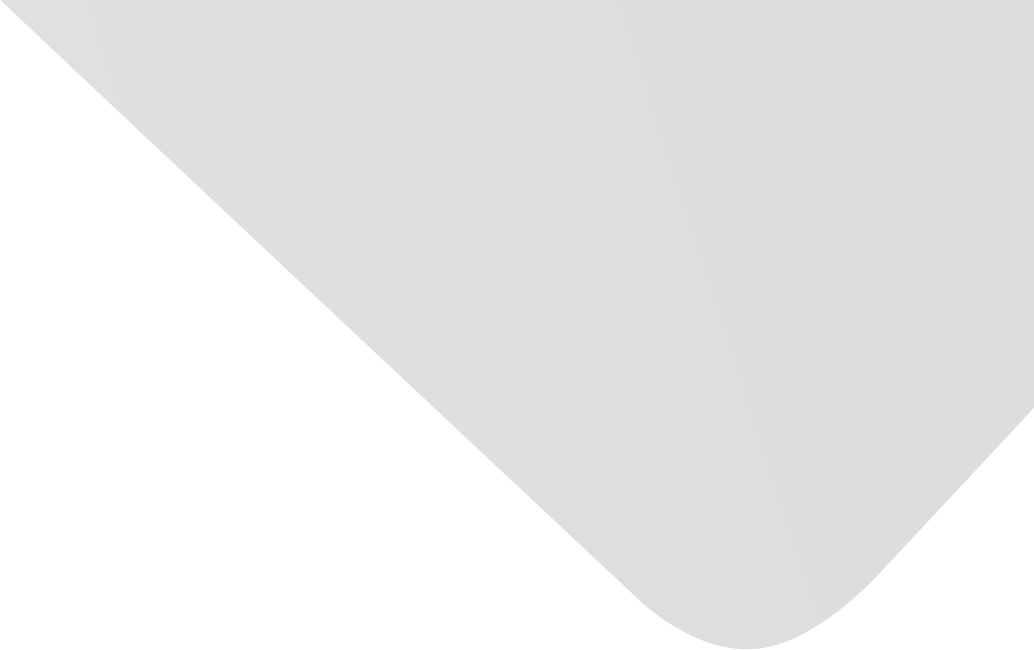
Prediction of Highway Tunnel Pavement Performance Based on Digital Twin and Multiple Time Series Stacking
Joint Authors
Yu, Gang
Zhang, Shuang
Hu, Min
Wang, Y. Ken
Source
Issue
Vol. 2020, Issue 2020 (31 Dec. 2020), pp.1-21, 21 p.
Publisher
Hindawi Publishing Corporation
Publication Date
2020-12-04
Country of Publication
Egypt
No. of Pages
21
Main Subjects
Abstract EN
The existing pavement performance prediction methods are limited to single-factor predictions, which often face the challenges of high cost, low efficiency, and poor accuracy.
It is difficult to simultaneously solve the temporal, spatial, and exogenous dependencies between pavement performance data and maintenance, the service life of highways, the environment, and other factors.
Digital twin technology based on the building information modeling (BIM) model, combined with machine learning, puts forward a new perspective and method for the accurate and timely prediction of pavement performance.
In this paper, we propose a highway tunnel pavement performance prediction approach based on a digital twin and multiple time series stacking (MTSS).
This paper (1) establishes an MTSS prediction model with heterogeneous stacking of eXtreme gradient boosting (XGBoost), the artificial neural network (ANN), random forest (RF), ridge regression, and support vector regression (SVR) component learners after exploratory data analysis (EDA); (2) proposes a method based on multiple time series feature extraction to accurately predict the pavement performance change trend, using the highway segment as the minimum computing unit and considering multiple factors; (3) uses grid search with the k-fold cross validation method to optimize hyperparameters to ensure the robustness, stability, and generalization ability of the prediction model; and (4) constructs a digital twin for pavement performance prediction to realize the real-time dynamic evolution of prediction.
The method proposed in this study is applied in the life cycle management of the Dalian highway-crossing tunnel in Shanghai, China.
A dataset covering 2010–2019 is collected for real-time prediction of the pavement performance.
The prediction accuracy evaluation shows that the mean absolute error (MAE) is 0.1314, the root mean squared error (RMSE) is 0.0386, the mean absolute percentage error (MAPE) is 5.10%, and the accuracy is 94.90%.
Its overall performance is better than a single model.
The results verify that the prediction method based on digital twin and MTSS is feasible and effective in the highway tunnel pavement performance prediction.
American Psychological Association (APA)
Yu, Gang& Zhang, Shuang& Hu, Min& Wang, Y. Ken. 2020. Prediction of Highway Tunnel Pavement Performance Based on Digital Twin and Multiple Time Series Stacking. Advances in Civil Engineering،Vol. 2020, no. 2020, pp.1-21.
https://search.emarefa.net/detail/BIM-1123337
Modern Language Association (MLA)
Yu, Gang…[et al.]. Prediction of Highway Tunnel Pavement Performance Based on Digital Twin and Multiple Time Series Stacking. Advances in Civil Engineering No. 2020 (2020), pp.1-21.
https://search.emarefa.net/detail/BIM-1123337
American Medical Association (AMA)
Yu, Gang& Zhang, Shuang& Hu, Min& Wang, Y. Ken. Prediction of Highway Tunnel Pavement Performance Based on Digital Twin and Multiple Time Series Stacking. Advances in Civil Engineering. 2020. Vol. 2020, no. 2020, pp.1-21.
https://search.emarefa.net/detail/BIM-1123337
Data Type
Journal Articles
Language
English
Notes
Includes bibliographical references
Record ID
BIM-1123337