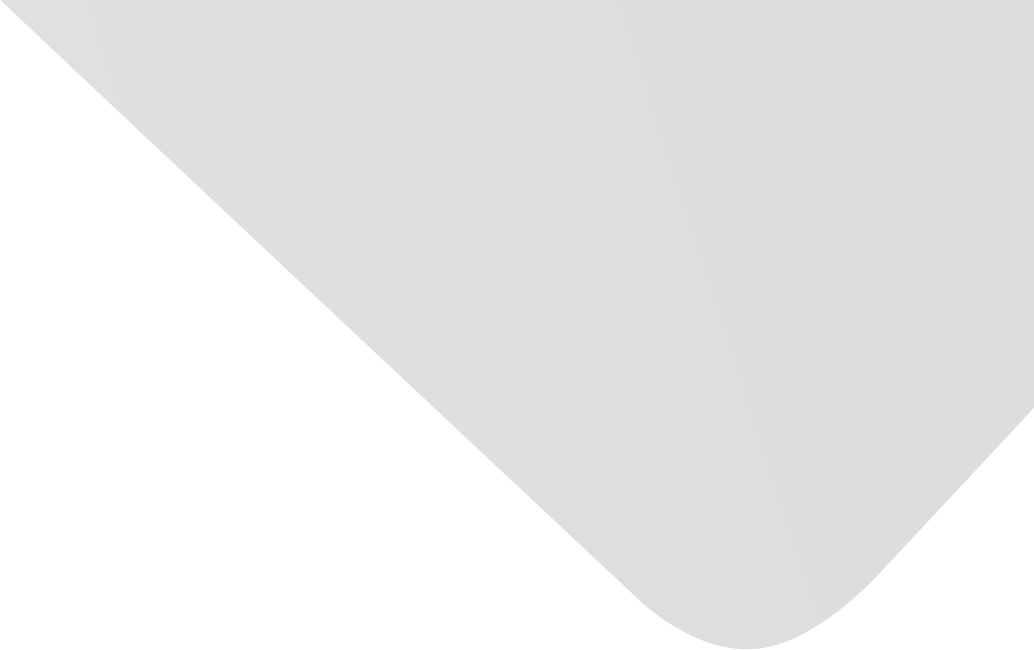
Predicting Soil Organic Carbon Content Using Hyperspectral Remote Sensing in a Degraded Mountain Landscape in Lesotho
Joint Authors
Bangelesa, Freddy
Adam, Elhadi
Knight, Jasper
Dhau, Inos
Ramudzuli, Marubini
Mokotjomela, Thabiso M.
Source
Applied and Environmental Soil Science
Issue
Vol. 2020, Issue 2020 (31 Dec. 2020), pp.1-11, 11 p.
Publisher
Hindawi Publishing Corporation
Publication Date
2020-04-13
Country of Publication
Egypt
No. of Pages
11
Main Subjects
Earth Science , Water and Environment
Abstract EN
Soil organic carbon constitutes an important indicator of soil fertility.
The purpose of this study was to predict soil organic carbon content in the mountainous terrain of eastern Lesotho, southern Africa, which is an area of high endemic biodiversity as well as an area extensively used for small-scale agriculture.
An integrated field and laboratory approach was undertaken, through measurements of reflectance spectra of soil using an Analytical Spectral Device (ASD) FieldSpec® 4 optical sensor.
Soil spectra were collected on the land surface under field conditions and then on soil in the laboratory, in order to assess the accuracy of field spectroscopy-based models.
The predictive performance of two different statistical models (random forest and partial least square regression) was compared.
Results show that random forest regression can most accurately predict the soil organic carbon contents on an independent dataset using the field spectroscopy data.
In contrast, the partial least square regression model overfits the calibration dataset.
Important wavelengths to predict soil organic contents were localised around the visible range (400–700 nm).
This study shows that soil organic carbon can be most accurately estimated using derivative field spectroscopy measurements and random forest regression.
American Psychological Association (APA)
Bangelesa, Freddy& Adam, Elhadi& Knight, Jasper& Dhau, Inos& Ramudzuli, Marubini& Mokotjomela, Thabiso M.. 2020. Predicting Soil Organic Carbon Content Using Hyperspectral Remote Sensing in a Degraded Mountain Landscape in Lesotho. Applied and Environmental Soil Science،Vol. 2020, no. 2020, pp.1-11.
https://search.emarefa.net/detail/BIM-1126265
Modern Language Association (MLA)
Bangelesa, Freddy…[et al.]. Predicting Soil Organic Carbon Content Using Hyperspectral Remote Sensing in a Degraded Mountain Landscape in Lesotho. Applied and Environmental Soil Science No. 2020 (2020), pp.1-11.
https://search.emarefa.net/detail/BIM-1126265
American Medical Association (AMA)
Bangelesa, Freddy& Adam, Elhadi& Knight, Jasper& Dhau, Inos& Ramudzuli, Marubini& Mokotjomela, Thabiso M.. Predicting Soil Organic Carbon Content Using Hyperspectral Remote Sensing in a Degraded Mountain Landscape in Lesotho. Applied and Environmental Soil Science. 2020. Vol. 2020, no. 2020, pp.1-11.
https://search.emarefa.net/detail/BIM-1126265
Data Type
Journal Articles
Language
English
Notes
Includes bibliographical references
Record ID
BIM-1126265