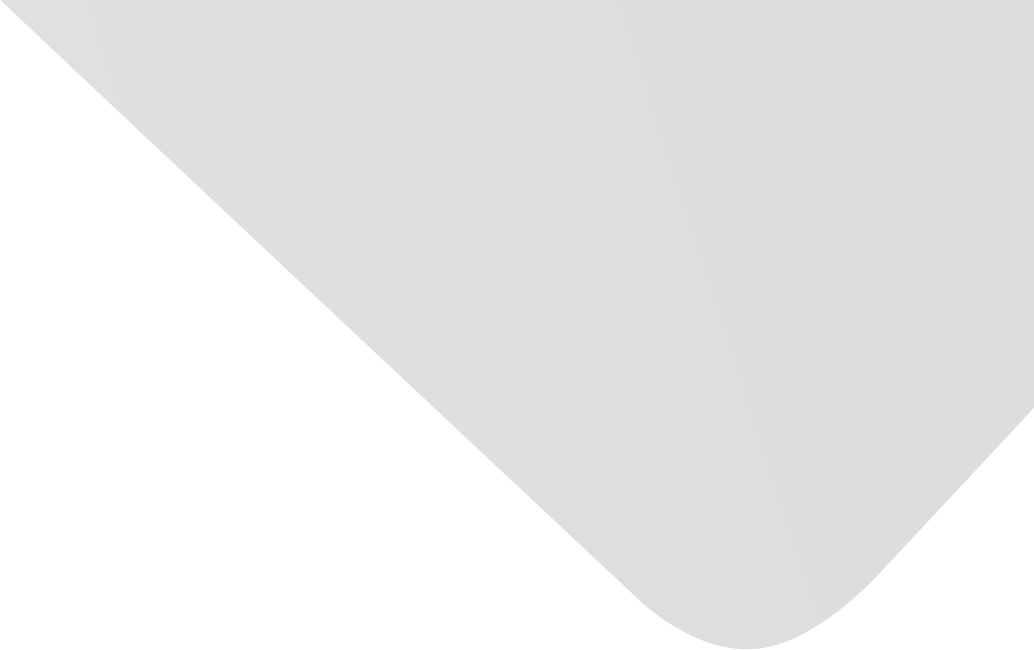
Predicting Bank Operational Efficiency Using Machine Learning Algorithm: Comparative Study of Decision Tree, Random Forest, and Neural Networks
Joint Authors
Appiahene, Peter
Missah, Yaw Marfo
Najim, Ussiph
Source
Issue
Vol. 2020, Issue 2020 (31 Dec. 2020), pp.1-12, 12 p.
Publisher
Hindawi Publishing Corporation
Publication Date
2020-07-23
Country of Publication
Egypt
No. of Pages
12
Main Subjects
Abstract EN
The financial crisis that hit Ghana from 2015 to 2018 has raised various issues with respect to the efficiency of banks and the safety of depositors’ in the banking industry.
As part of measures to improve the banking sector and also restore customers’ confidence, efficiency and performance analysis in the banking industry has become a hot issue.
This is because stakeholders have to detect the underlying causes of inefficiencies within the banking industry.
Nonparametric methods such as Data Envelopment Analysis (DEA) have been suggested in the literature as a good measure of banks’ efficiency and performance.
Machine learning algorithms have also been viewed as a good tool to estimate various nonparametric and nonlinear problems.
This paper presents a combined DEA with three machine learning approaches in evaluating bank efficiency and performance using 444 Ghanaian bank branches, Decision Making Units (DMUs).
The results were compared with the corresponding efficiency ratings obtained from the DEA.
Finally, the prediction accuracies of the three machine learning algorithm models were compared.
The results suggested that the decision tree (DT) and its C5.0 algorithm provided the best predictive model.
It had 100% accuracy in predicting the 134 holdout sample dataset (30% banks) and a P value of 0.00.
The DT was followed closely by random forest algorithm with a predictive accuracy of 98.5% and a P value of 0.00 and finally the neural network (86.6% accuracy) with a P value 0.66.
The study concluded that banks in Ghana can use the result of this study to predict their respective efficiencies.
All experiments were performed within a simulation environment and conducted in R studio using R codes.
American Psychological Association (APA)
Appiahene, Peter& Missah, Yaw Marfo& Najim, Ussiph. 2020. Predicting Bank Operational Efficiency Using Machine Learning Algorithm: Comparative Study of Decision Tree, Random Forest, and Neural Networks. Advances in Fuzzy Systems،Vol. 2020, no. 2020, pp.1-12.
https://search.emarefa.net/detail/BIM-1126388
Modern Language Association (MLA)
Appiahene, Peter…[et al.]. Predicting Bank Operational Efficiency Using Machine Learning Algorithm: Comparative Study of Decision Tree, Random Forest, and Neural Networks. Advances in Fuzzy Systems No. 2020 (2020), pp.1-12.
https://search.emarefa.net/detail/BIM-1126388
American Medical Association (AMA)
Appiahene, Peter& Missah, Yaw Marfo& Najim, Ussiph. Predicting Bank Operational Efficiency Using Machine Learning Algorithm: Comparative Study of Decision Tree, Random Forest, and Neural Networks. Advances in Fuzzy Systems. 2020. Vol. 2020, no. 2020, pp.1-12.
https://search.emarefa.net/detail/BIM-1126388
Data Type
Journal Articles
Language
English
Notes
Includes bibliographical references
Record ID
BIM-1126388