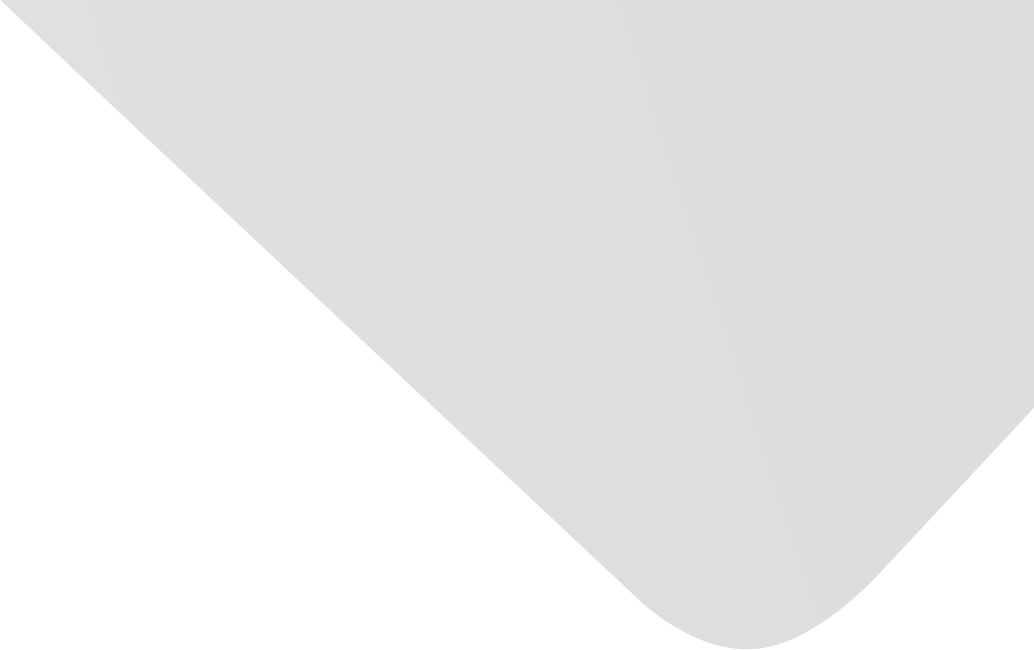
An Efficient Feature Selection Strategy Based on Multiple Support Vector Machine Technology with Gene Expression Data
Joint Authors
Zhang, Ying
Deng, Qingchun
Liang, Wenbin
Zou, Xianchun
Source
Issue
Vol. 2018, Issue 2018 (31 Dec. 2018), pp.1-11, 11 p.
Publisher
Hindawi Publishing Corporation
Publication Date
2018-08-30
Country of Publication
Egypt
No. of Pages
11
Main Subjects
Abstract EN
The application of gene expression data to the diagnosis and classification of cancer has become a hot issue in the field of cancer classification.
Gene expression data usually contains a large number of tumor-free data and has the characteristics of high dimensions.
In order to select determinant genes related to breast cancer from the initial gene expression data, we propose a new feature selection method, namely, support vector machine based on recursive feature elimination and parameter optimization (SVM-RFE-PO).
The grid search (GS) algorithm, the particle swarm optimization (PSO) algorithm, and the genetic algorithm (GA) are applied to search the optimal parameters in the feature selection process.
Herein, the new feature selection method contains three kinds of algorithms: support vector machine based on recursive feature elimination and grid search (SVM-RFE-GS), support vector machine based on recursive feature elimination and particle swarm optimization (SVM-RFE-PSO), and support vector machine based on recursive feature elimination and genetic algorithm (SVM-RFE-GA).
Then the selected optimal feature subsets are used to train the SVM classifier for cancer classification.
We also use random forest feature selection (RFFS), random forest feature selection and grid search (RFFS-GS), and minimal redundancy maximal relevance (mRMR) algorithm as feature selection methods to compare the effects of the SVM-RFE-PO algorithm.
The results showed that the feature subset obtained by feature selection using SVM-RFE-PSO algorithm results has a better prediction performance of Area Under Curve (AUC) in the testing data set.
This algorithm not only is time-saving, but also is capable of extracting more representative and useful genes.
American Psychological Association (APA)
Zhang, Ying& Deng, Qingchun& Liang, Wenbin& Zou, Xianchun. 2018. An Efficient Feature Selection Strategy Based on Multiple Support Vector Machine Technology with Gene Expression Data. BioMed Research International،Vol. 2018, no. 2018, pp.1-11.
https://search.emarefa.net/detail/BIM-1128596
Modern Language Association (MLA)
Zhang, Ying…[et al.]. An Efficient Feature Selection Strategy Based on Multiple Support Vector Machine Technology with Gene Expression Data. BioMed Research International No. 2018 (2018), pp.1-11.
https://search.emarefa.net/detail/BIM-1128596
American Medical Association (AMA)
Zhang, Ying& Deng, Qingchun& Liang, Wenbin& Zou, Xianchun. An Efficient Feature Selection Strategy Based on Multiple Support Vector Machine Technology with Gene Expression Data. BioMed Research International. 2018. Vol. 2018, no. 2018, pp.1-11.
https://search.emarefa.net/detail/BIM-1128596
Data Type
Journal Articles
Language
English
Notes
Includes bibliographical references
Record ID
BIM-1128596