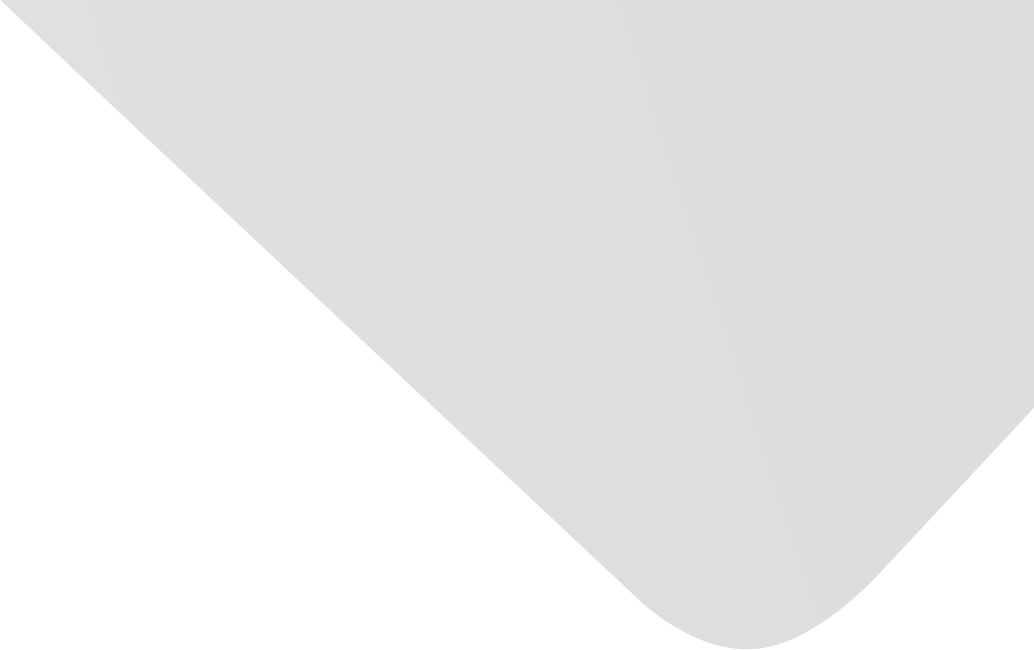
Noninvasive Evaluation of the Pathologic Grade of Hepatocellular Carcinoma Using MCF-3DCNN: A Pilot Study
Joint Authors
Yang, Da-wei
Yang, Zheng-han
Wang, Zhenchang
Jia, Xi-bin
Xiao, Yu-jie
Wang, Xiao-pei
Source
Issue
Vol. 2019, Issue 2019 (31 Dec. 2019), pp.1-12, 12 p.
Publisher
Hindawi Publishing Corporation
Publication Date
2019-04-28
Country of Publication
Egypt
No. of Pages
12
Main Subjects
Abstract EN
Purpose.
To evaluate the diagnostic performance of deep learning with a multichannel fusion three-dimensional convolutional neural network (MCF-3DCNN) in the differentiation of the pathologic grades of hepatocellular carcinoma (HCC) based on dynamic contrast-enhanced magnetic resonance images (DCE-MR images).
Methods and Materials.
Fifty-one histologically proven HCCs from 42 consecutive patients from January 2015 to September 2017 were included in this retrospective study.
Pathologic examinations revealed nine well-differentiated (WD), 35 moderately differentiated (MD), and seven poorly differentiated (PD) HCCs.
DCE-MR images with five phases were collected using a 3.0 Tesla MR scanner.
The 4D-tensor representation was employed to organize the collected data in one temporal and three spatial dimensions by referring to the phases and 3D scanning slices of the DCE-MR images.
A deep learning diagnosis model with MCF-3DCNN was proposed, and the structure of MCF-3DCNN was determined to approximate clinical diagnosis experience by taking into account the significance of the spatial and temporal information from DCE-MR images.
Then, MCF-3DCNN was trained based on well-labeled samples of HCC lesions from real patient cases by experienced radiologists.
The accuracy when differentiating the pathologic grades of HCC was calculated, and the performance of MCF-3DCNN in lesion diagnosis was assessed.
Additionally, the areas under the receiver operating characteristic curves (AUC) for distinguishing WD, MD, and PD HCCs were calculated.
Results.
MCF-3DCNN achieved an average accuracy of 0.7396±0.0104 with regard to totally differentiating the pathologic grade of HCC.
MCF-3DCNN also achieved the highest diagnostic performance for discriminating WD HCCs from others, with an average AUC, accuracy, sensitivity, and specificity of 0.96, 91.00%, 96.88%, and 89.62%, respectively.
Conclusions.
This study indicates that MCF-3DCNN can be a promising technology for evaluating the pathologic grade of HCC based on DCE-MR images.
American Psychological Association (APA)
Yang, Da-wei& Jia, Xi-bin& Xiao, Yu-jie& Wang, Xiao-pei& Wang, Zhenchang& Yang, Zheng-han. 2019. Noninvasive Evaluation of the Pathologic Grade of Hepatocellular Carcinoma Using MCF-3DCNN: A Pilot Study. BioMed Research International،Vol. 2019, no. 2019, pp.1-12.
https://search.emarefa.net/detail/BIM-1128835
Modern Language Association (MLA)
Yang, Da-wei…[et al.]. Noninvasive Evaluation of the Pathologic Grade of Hepatocellular Carcinoma Using MCF-3DCNN: A Pilot Study. BioMed Research International No. 2019 (2019), pp.1-12.
https://search.emarefa.net/detail/BIM-1128835
American Medical Association (AMA)
Yang, Da-wei& Jia, Xi-bin& Xiao, Yu-jie& Wang, Xiao-pei& Wang, Zhenchang& Yang, Zheng-han. Noninvasive Evaluation of the Pathologic Grade of Hepatocellular Carcinoma Using MCF-3DCNN: A Pilot Study. BioMed Research International. 2019. Vol. 2019, no. 2019, pp.1-12.
https://search.emarefa.net/detail/BIM-1128835
Data Type
Journal Articles
Language
English
Notes
Includes bibliographical references
Record ID
BIM-1128835