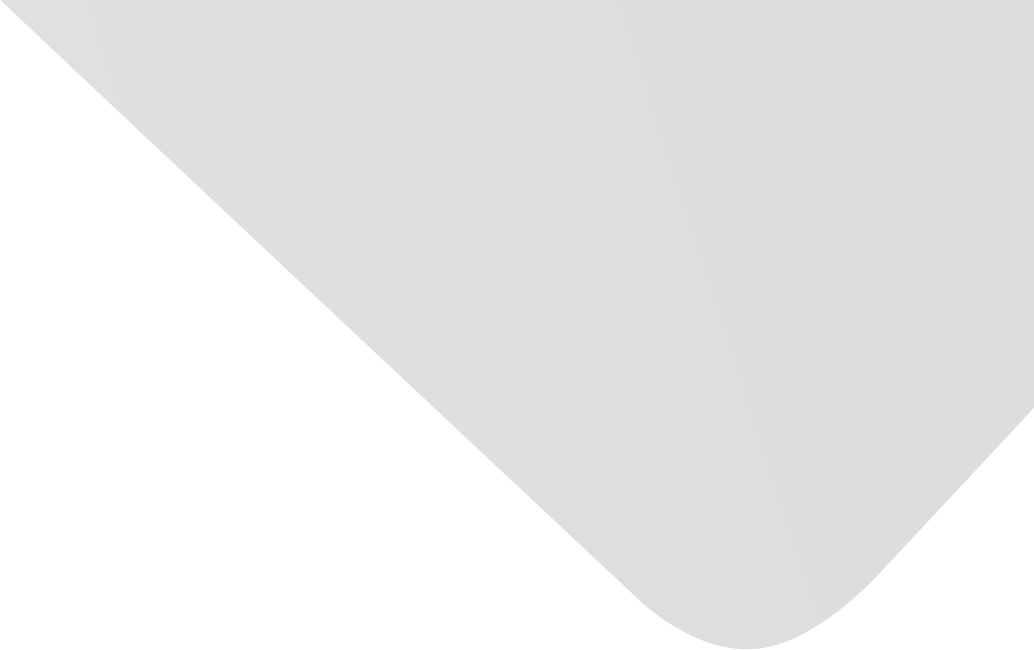
Large-Scale Coarse-to-Fine Object Retrieval Ontology and Deep Local Multitask Learning
Joint Authors
Ly, Ngoc Q.
Do, Tuong K.
Nguyen, Binh X.
Source
Computational Intelligence and Neuroscience
Issue
Vol. 2019, Issue 2019 (31 Dec. 2019), pp.1-40, 40 p.
Publisher
Hindawi Publishing Corporation
Publication Date
2019-07-18
Country of Publication
Egypt
No. of Pages
40
Main Subjects
Abstract EN
Object retrieval plays an increasingly important role in video surveillance, digital marketing, e-commerce, etc.
It is facing challenges such as large-scale datasets, imbalanced data, viewpoint, cluster background, and fine-grained details (attributes).
This paper has proposed a model to integrate object ontology, a local multitask deep neural network (local MDNN), and an imbalanced data solver to take advantages and overcome the shortcomings of deep learning network models to improve the performance of the large-scale object retrieval system from the coarse-grained level (categories) to the fine-grained level (attributes).
Our proposed coarse-to-fine object retrieval (CFOR) system can be robust and resistant to the challenges listed above.
To the best of our knowledge, the new main point of our CFOR system is the power of mutual support of object ontology, a local MDNN, and an imbalanced data solver in a unified system.
Object ontology supports the exploitation of the inner-group correlations to improve the system performance in category classification, attribute classification, and conducting training flow and retrieval flow to save computational costs in the training stage and retrieval stage on large-scale datasets, respectively.
A local MDNN supports linking object ontology to the raw data, and an imbalanced data solver based on Matthews’ correlation coefficient (MCC) addresses that the imbalance of data has contributed effectively to increasing the quality of object ontology realization without adjusting network architecture and data augmentation.
In order to evaluate the performance of the CFOR system, we experimented on the DeepFashion dataset.
This paper has shown that our local MDNN framework based on the pretrained NASNet architecture has achieved better performance (14.2% higher in recall rate) compared to single-task learning (STL) in the attribute learning task; it has also shown that our model with an imbalanced data solver has achieved better performance (5.14% higher in recall rate for fewer data attributes) compared to models that do not take this into account.
Moreover, MAP@30 hovers 0.815 in retrieval on an average of 35 imbalanced fashion attributes.
American Psychological Association (APA)
Ly, Ngoc Q.& Do, Tuong K.& Nguyen, Binh X.. 2019. Large-Scale Coarse-to-Fine Object Retrieval Ontology and Deep Local Multitask Learning. Computational Intelligence and Neuroscience،Vol. 2019, no. 2019, pp.1-40.
https://search.emarefa.net/detail/BIM-1129333
Modern Language Association (MLA)
Ly, Ngoc Q.…[et al.]. Large-Scale Coarse-to-Fine Object Retrieval Ontology and Deep Local Multitask Learning. Computational Intelligence and Neuroscience No. 2019 (2019), pp.1-40.
https://search.emarefa.net/detail/BIM-1129333
American Medical Association (AMA)
Ly, Ngoc Q.& Do, Tuong K.& Nguyen, Binh X.. Large-Scale Coarse-to-Fine Object Retrieval Ontology and Deep Local Multitask Learning. Computational Intelligence and Neuroscience. 2019. Vol. 2019, no. 2019, pp.1-40.
https://search.emarefa.net/detail/BIM-1129333
Data Type
Journal Articles
Language
English
Notes
Includes bibliographical references
Record ID
BIM-1129333