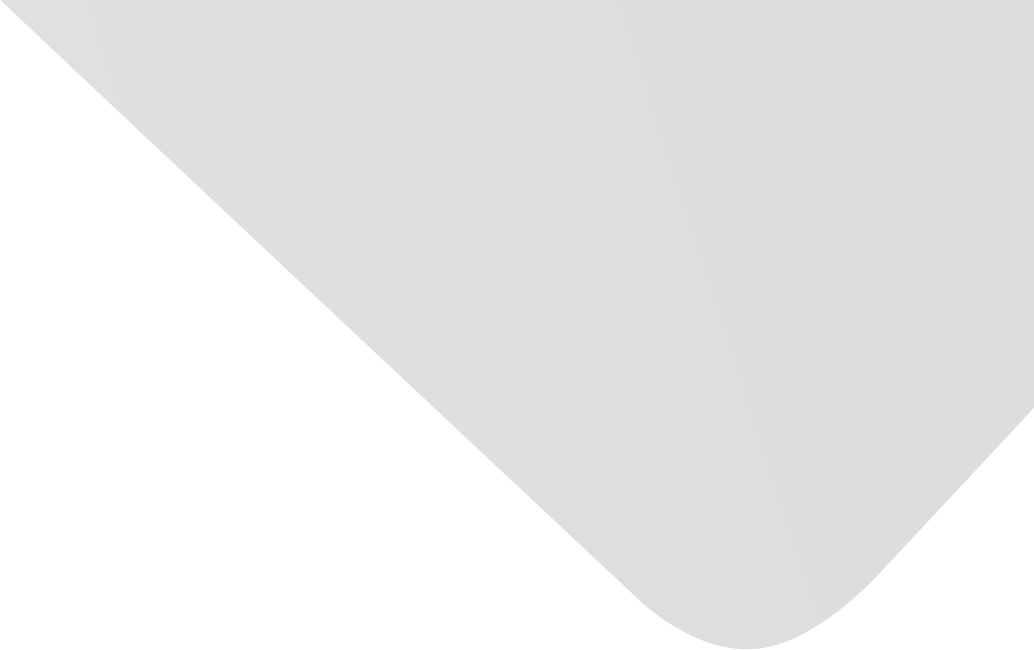
An Improved Refined Composite Multivariate Multiscale Fuzzy Entropy Method for MI-EEG Feature Extraction
Joint Authors
Li, Mingai
Yang, Jinfu
Wang, Ruotu
Duan, Lijuan
Source
Computational Intelligence and Neuroscience
Issue
Vol. 2019, Issue 2019 (31 Dec. 2019), pp.1-12, 12 p.
Publisher
Hindawi Publishing Corporation
Publication Date
2019-03-28
Country of Publication
Egypt
No. of Pages
12
Main Subjects
Abstract EN
Feature extraction of motor imagery electroencephalogram (MI-EEG) has shown good application prospects in the field of medical health.
Also, multivariate entropy-based feature extraction methods have been gradually applied to analyze complex multichannel biomedical signals, such as EEG and electromyography.
Compared with traditional multivariate entropies, refined composite multivariate multiscale fuzzy entropy (RCmvMFE) overcomes the defect of unstable entropy values caused by the scale factor increase and is beneficial towards obtaining richer feature information.
However, the coarse-grained process of RCmvMFE is mean filtered, which weakens Gaussian noise and is powerless against random impulse noise interference.
This yields poor quality feature information and low accuracy classification.
In this paper, RCmvMFE is improved (IRCmvMFE) by using composite filters in the coarse-grained procedure to enhance filter performance.
Median filters are employed to remove the impulse noise interference from multichannel MI-EEG signals, and these filtered MI-EEGs are further smoothed by the mean filters.
The multiscale IRCmvMFEs are calculated for all channels of composite filtered MI-EEGs, forming a feature vector, and a support vector machine is used for pattern classification.
Based on two public datasets with different motor imagery tasks, the recognition results of 10 × 10-fold cross-validation achieved 99.43% and 99.86%, respectively, and the statistical analysis of experimental results was completed, showing the effectiveness of IRCmvMFE, as well.
The proposed IRCmvMFE-based feature extraction method is superior compared to entropy-based and traditional methods.
American Psychological Association (APA)
Li, Mingai& Wang, Ruotu& Yang, Jinfu& Duan, Lijuan. 2019. An Improved Refined Composite Multivariate Multiscale Fuzzy Entropy Method for MI-EEG Feature Extraction. Computational Intelligence and Neuroscience،Vol. 2019, no. 2019, pp.1-12.
https://search.emarefa.net/detail/BIM-1129574
Modern Language Association (MLA)
Li, Mingai…[et al.]. An Improved Refined Composite Multivariate Multiscale Fuzzy Entropy Method for MI-EEG Feature Extraction. Computational Intelligence and Neuroscience No. 2019 (2019), pp.1-12.
https://search.emarefa.net/detail/BIM-1129574
American Medical Association (AMA)
Li, Mingai& Wang, Ruotu& Yang, Jinfu& Duan, Lijuan. An Improved Refined Composite Multivariate Multiscale Fuzzy Entropy Method for MI-EEG Feature Extraction. Computational Intelligence and Neuroscience. 2019. Vol. 2019, no. 2019, pp.1-12.
https://search.emarefa.net/detail/BIM-1129574
Data Type
Journal Articles
Language
English
Notes
Includes bibliographical references
Record ID
BIM-1129574