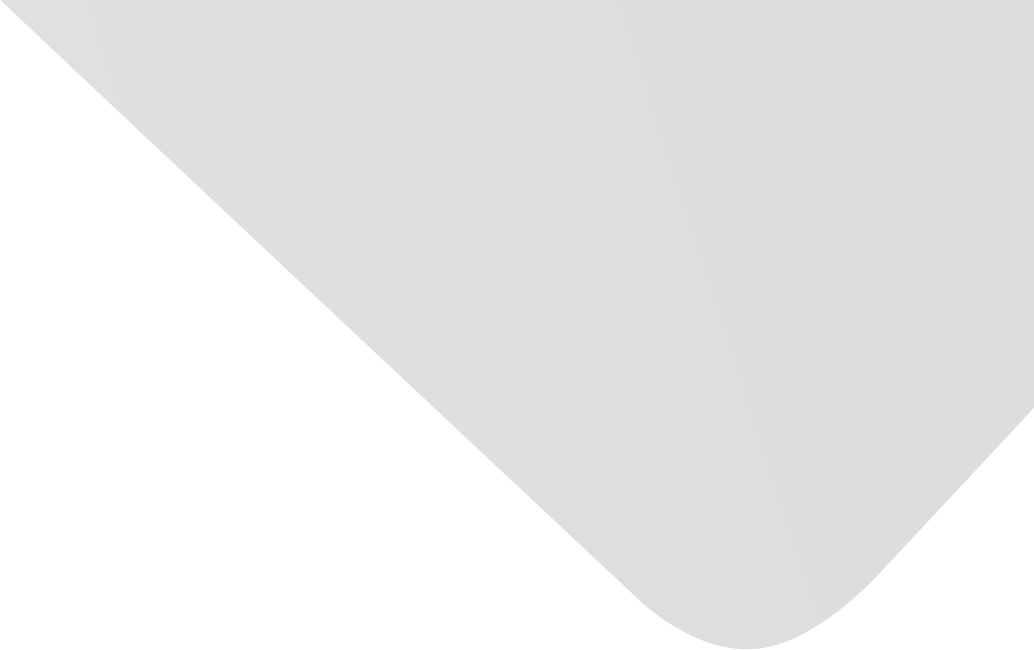
Facing High EEG Signals Variability during Classification Using Fractal Dimension and Different Cutoff Frequencies
Joint Authors
Vázquez, Roberto A.
Salazar-Varas, R.
Source
Computational Intelligence and Neuroscience
Issue
Vol. 2019, Issue 2019 (31 Dec. 2019), pp.1-12, 12 p.
Publisher
Hindawi Publishing Corporation
Publication Date
2019-05-20
Country of Publication
Egypt
No. of Pages
12
Main Subjects
Abstract EN
In the development of a brain-computer interface (BCI), some issues should be regarded in order to improve its reliability and performance.
Perhaps, one of the most challenging issues is related to the high variability of the brain signals, which directly impacts the accuracy of the classification.
In this sense, novel feature extraction techniques should be explored in order to select those able to face this variability.
Furthermore, to improve the performance of the selected feature extraction technique, the parameters of the filter applied in the preprocessing stage need to be properly selected.
Then, this work presents an analysis of the robustness of the fractal dimension as feature extraction technique under high variability of the EEG signals, particularly when the training data are recorded one day and the testing data are obtained on a different day.
The results are compared with those obtained by an autoregressive model, which is a technique commonly used in BCI applications.
Also, the effect of properly selecting the cutoff frequencies of the filter in the preprocessing stage is evaluated.
This research is supported by several experiments carried out using a public data set from the BCI international competition, specifically data set 2a from BCIIC IV, related to motor tasks.
By a statistical test, it is demonstrated that the performance achieved using the fractal dimension is significantly better than that reached by the AR model.
Also, it is demonstrated that the selection of the appropriate cutoff frequencies improves significantly the performance in the classification.
The increase rate is approximately of 17%.
American Psychological Association (APA)
Salazar-Varas, R.& Vázquez, Roberto A.. 2019. Facing High EEG Signals Variability during Classification Using Fractal Dimension and Different Cutoff Frequencies. Computational Intelligence and Neuroscience،Vol. 2019, no. 2019, pp.1-12.
https://search.emarefa.net/detail/BIM-1129661
Modern Language Association (MLA)
Salazar-Varas, R.& Vázquez, Roberto A.. Facing High EEG Signals Variability during Classification Using Fractal Dimension and Different Cutoff Frequencies. Computational Intelligence and Neuroscience No. 2019 (2019), pp.1-12.
https://search.emarefa.net/detail/BIM-1129661
American Medical Association (AMA)
Salazar-Varas, R.& Vázquez, Roberto A.. Facing High EEG Signals Variability during Classification Using Fractal Dimension and Different Cutoff Frequencies. Computational Intelligence and Neuroscience. 2019. Vol. 2019, no. 2019, pp.1-12.
https://search.emarefa.net/detail/BIM-1129661
Data Type
Journal Articles
Language
English
Notes
Includes bibliographical references
Record ID
BIM-1129661