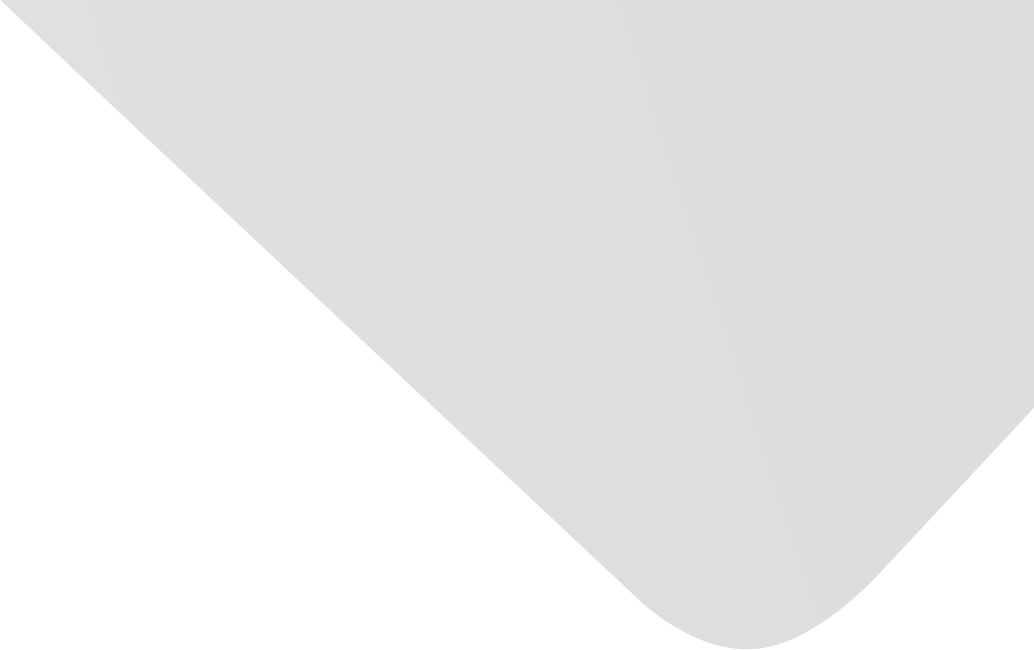
Learning Domain-Independent Deep Representations by Mutual Information Minimization
Joint Authors
Wang, Jing-Yan
Wang, Ke
Liu, Jiayong
Source
Computational Intelligence and Neuroscience
Issue
Vol. 2019, Issue 2019 (31 Dec. 2019), pp.1-14, 14 p.
Publisher
Hindawi Publishing Corporation
Publication Date
2019-06-16
Country of Publication
Egypt
No. of Pages
14
Main Subjects
Abstract EN
Domain transfer learning aims to learn common data representations from a source domain and a target domain so that the source domain data can help the classification of the target domain.
Conventional transfer representation learning imposes the distributions of source and target domain representations to be similar, which heavily relies on the characterization of the distributions of domains and the distribution matching criteria.
In this paper, we proposed a novel framework for domain transfer representation learning.
Our motive is to make the learned representations of data points independent from the domains which they belong to.
In other words, from an optimal cross-domain representation of a data point, it is difficult to tell which domain it is from.
In this way, the learned representations can be generalized to different domains.
To measure the dependency between the representations and the corresponding domain which the data points belong to, we propose to use the mutual information between the representations and the domain-belonging indicators.
By minimizing such mutual information, we learn the representations which are independent from domains.
We build a classwise deep convolutional network model as a representation model and maximize the margin of each data point of the corresponding class, which is defined over the intraclass and interclass neighborhood.
To learn the parameters of the model, we construct a unified minimization problem where the margins are maximized while the representation-domain mutual information is minimized.
In this way, we learn representations which are not only discriminate but also independent from domains.
An iterative algorithm based on the Adam optimization method is proposed to solve the minimization to learn the classwise deep model parameters and the cross-domain representations simultaneously.
Extensive experiments over benchmark datasets show its effectiveness and advantage over existing domain transfer learning methods.
American Psychological Association (APA)
Wang, Ke& Liu, Jiayong& Wang, Jing-Yan. 2019. Learning Domain-Independent Deep Representations by Mutual Information Minimization. Computational Intelligence and Neuroscience،Vol. 2019, no. 2019, pp.1-14.
https://search.emarefa.net/detail/BIM-1129691
Modern Language Association (MLA)
Wang, Ke…[et al.]. Learning Domain-Independent Deep Representations by Mutual Information Minimization. Computational Intelligence and Neuroscience No. 2019 (2019), pp.1-14.
https://search.emarefa.net/detail/BIM-1129691
American Medical Association (AMA)
Wang, Ke& Liu, Jiayong& Wang, Jing-Yan. Learning Domain-Independent Deep Representations by Mutual Information Minimization. Computational Intelligence and Neuroscience. 2019. Vol. 2019, no. 2019, pp.1-14.
https://search.emarefa.net/detail/BIM-1129691
Data Type
Journal Articles
Language
English
Notes
Includes bibliographical references
Record ID
BIM-1129691