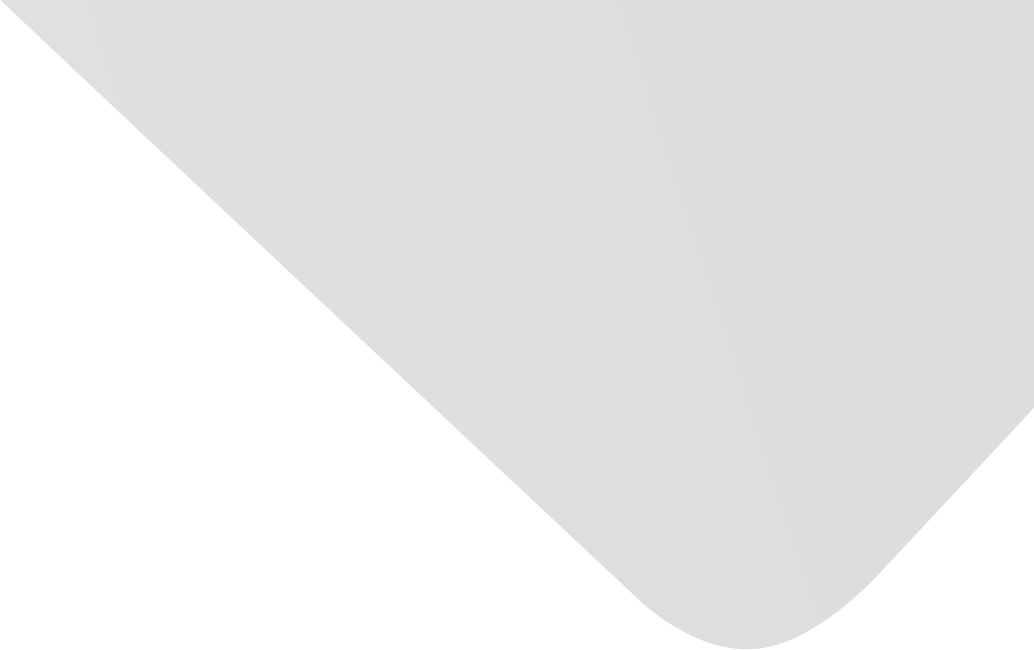
Emergency Department Capacity Planning: A Recurrent Neural Network and Simulation Approach
Joint Authors
Source
Computational and Mathematical Methods in Medicine
Issue
Vol. 2019, Issue 2019 (31 Dec. 2019), pp.1-13, 13 p.
Publisher
Hindawi Publishing Corporation
Publication Date
2019-11-15
Country of Publication
Egypt
No. of Pages
13
Main Subjects
Abstract EN
Emergency departments (EDs) play a vital role in the whole healthcare system as they are the first point of care in hospitals for urgent and critically ill patients.
Therefore, effective management of hospital’s ED is crucial in improving the quality of the healthcare service.
The effectiveness depends on how efficiently the hospital resources are used, particularly under budget constraints.
Simulation modeling is one of the best methods to optimize resources and needs inputs such as patients’ arrival time, patient’s length of stay (LOS), and the route of patients in the ED.
This study develops a simulation model to determine the optimum number of beds in an ED by minimizing the patients’ LOS.
The hospital data are analyzed, and patients’ LOS and the route of patients in the ED are determined.
To determine patients’ arrival times, the features associated with patients’ arrivals at ED are identified.
Mean arrival rate is used as a feature in addition to climatic and temporal variables.
The exhaustive feature-selection method has been used to determine the best subset of the features, and the mean arrival rate is determined as one of the most significant features.
This study is executed using the one-year ED arrival data together with five-year (43.824 study hours) ED arrival data to improve the accuracy of predictions.
Furthermore, ten different machine learning (ML) algorithms are used utilizing the same best subset of these features.
After a tenfold cross-validation experiment, based on mean absolute percentage error (MAPE), the stateful long short-term memory (LSTM) model performed better than other models with an accuracy of 47%, followed by the decision tree and random forest methods.
Using the simulation method, the LOS has been minimized by 7% and the number of beds at the ED has been optimized.
American Psychological Association (APA)
Nas, Serkan& Koyuncu, Melik. 2019. Emergency Department Capacity Planning: A Recurrent Neural Network and Simulation Approach. Computational and Mathematical Methods in Medicine،Vol. 2019, no. 2019, pp.1-13.
https://search.emarefa.net/detail/BIM-1130572
Modern Language Association (MLA)
Nas, Serkan& Koyuncu, Melik. Emergency Department Capacity Planning: A Recurrent Neural Network and Simulation Approach. Computational and Mathematical Methods in Medicine No. 2019 (2019), pp.1-13.
https://search.emarefa.net/detail/BIM-1130572
American Medical Association (AMA)
Nas, Serkan& Koyuncu, Melik. Emergency Department Capacity Planning: A Recurrent Neural Network and Simulation Approach. Computational and Mathematical Methods in Medicine. 2019. Vol. 2019, no. 2019, pp.1-13.
https://search.emarefa.net/detail/BIM-1130572
Data Type
Journal Articles
Language
English
Notes
Includes bibliographical references
Record ID
BIM-1130572