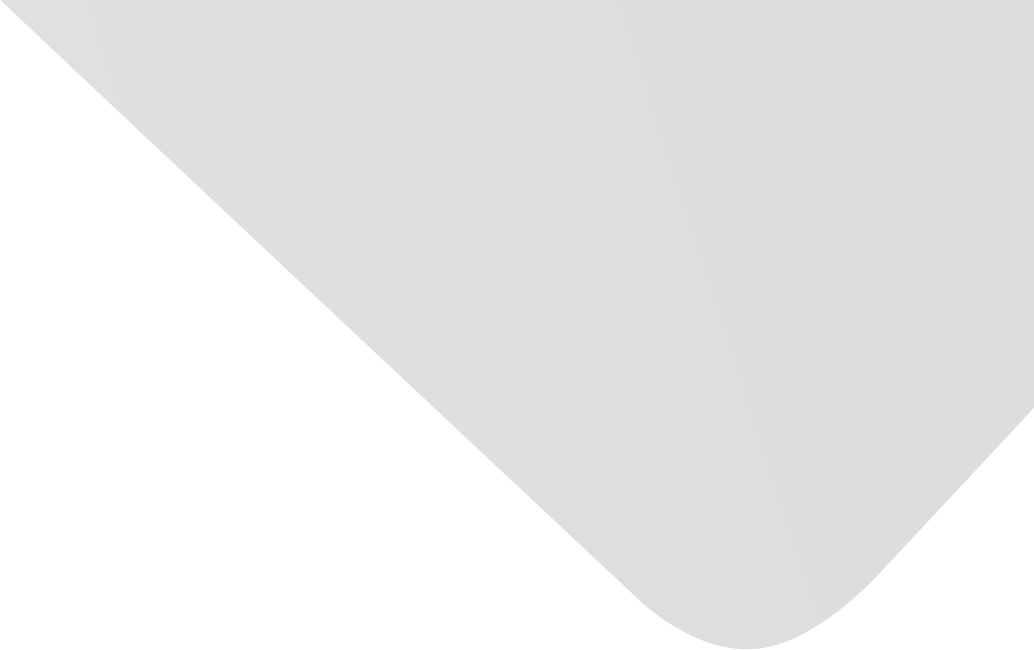
Generalization Bounds for Coregularized Multiple Kernel Learning
Joint Authors
Source
Computational Intelligence and Neuroscience
Issue
Vol. 2018, Issue 2018 (31 Dec. 2018), pp.1-13, 13 p.
Publisher
Hindawi Publishing Corporation
Publication Date
2018-11-01
Country of Publication
Egypt
No. of Pages
13
Main Subjects
Abstract EN
Multiple kernel learning (MKL) as an approach to automated kernel selection plays an important role in machine learning.
Some learning theories have been built to analyze the generalization of multiple kernel learning.
However, less work has been studied on multiple kernel learning in the framework of semisupervised learning.
In this paper, we analyze the generalization of multiple kernel learning in the framework of semisupervised multiview learning.
We apply Rademacher chaos complexity to control the performance of the candidate class of coregularized multiple kernels and obtain the generalization error bound of coregularized multiple kernel learning.
Furthermore, we show that the existing results about multiple kennel learning and coregularized kernel learning can be regarded as the special cases of our main results in this paper.
American Psychological Association (APA)
Wu, Xinxing& Hu, Guosheng. 2018. Generalization Bounds for Coregularized Multiple Kernel Learning. Computational Intelligence and Neuroscience،Vol. 2018, no. 2018, pp.1-13.
https://search.emarefa.net/detail/BIM-1130609
Modern Language Association (MLA)
Wu, Xinxing& Hu, Guosheng. Generalization Bounds for Coregularized Multiple Kernel Learning. Computational Intelligence and Neuroscience No. 2018 (2018), pp.1-13.
https://search.emarefa.net/detail/BIM-1130609
American Medical Association (AMA)
Wu, Xinxing& Hu, Guosheng. Generalization Bounds for Coregularized Multiple Kernel Learning. Computational Intelligence and Neuroscience. 2018. Vol. 2018, no. 2018, pp.1-13.
https://search.emarefa.net/detail/BIM-1130609
Data Type
Journal Articles
Language
English
Notes
Includes bibliographical references
Record ID
BIM-1130609