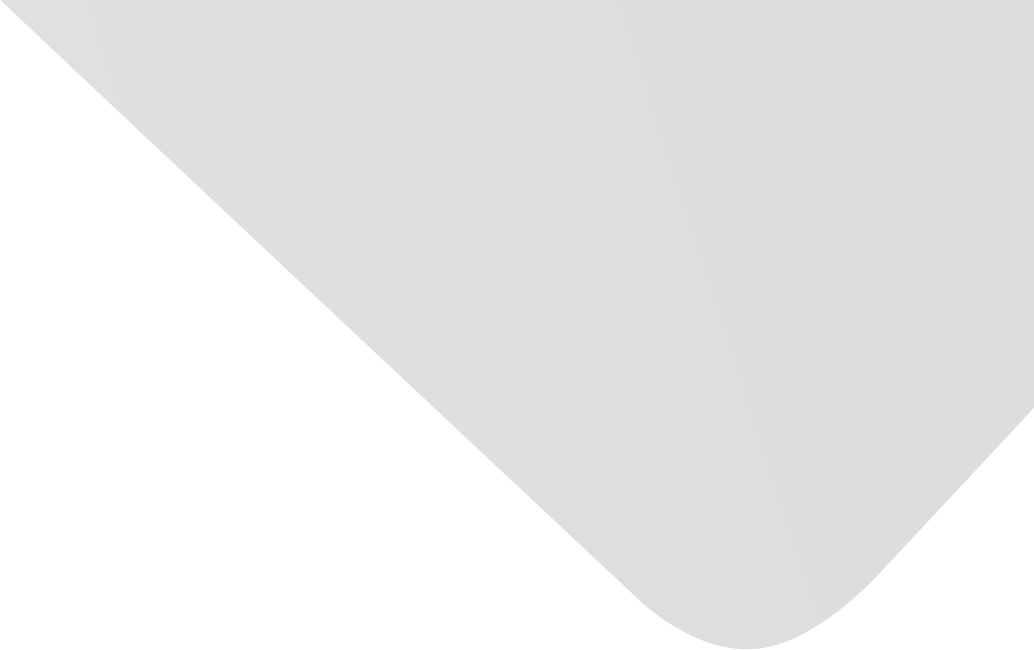
Correlation Assisted Strong Uncorrelating Transform Complex Common Spatial Patterns for Spatially Distant Channel Data
Joint Authors
Kim, Youngjoo
Ryu, Jiwoo
Park, Cheolsoo
Lee, Heejun
Lee, Seung Min
Source
Computational Intelligence and Neuroscience
Issue
Vol. 2018, Issue 2018 (31 Dec. 2018), pp.1-9, 9 p.
Publisher
Hindawi Publishing Corporation
Publication Date
2018-05-15
Country of Publication
Egypt
No. of Pages
9
Main Subjects
Abstract EN
The Strong Uncorrelating Transform Complex Common Spatial Patterns (SUTCCSP) algorithm, designed for multichannel data analysis, has a limitation on keeping the correlation information among channels during the simultaneous diagonalization process of the covariance and pseudocovariance matrices.
This paper focuses on the importance of preserving the correlation information among multichannel data and proposes the correlation assisted SUTCCSP (CASUT) algorithm to address this issue.
The performance of the proposed algorithm was demonstrated by classifying the motor imagery electroencephalogram (EEG) dataset.
The features were first extracted using CSP algorithms including the proposed method, and then the random forest classifier was utilized for the classification.
Experiments using CASUT yielded an average classification accuracy of 78.10 (%), which significantly outperformed those of original CSP, Complex Common Spatial Patterns (CCSP), and SUTCCSP with p-values less than 0.01, tested by the Wilcoxon signed rank test.
American Psychological Association (APA)
Kim, Youngjoo& Ryu, Jiwoo& Lee, Heejun& Lee, Seung Min& Park, Cheolsoo. 2018. Correlation Assisted Strong Uncorrelating Transform Complex Common Spatial Patterns for Spatially Distant Channel Data. Computational Intelligence and Neuroscience،Vol. 2018, no. 2018, pp.1-9.
https://search.emarefa.net/detail/BIM-1130717
Modern Language Association (MLA)
Kim, Youngjoo…[et al.]. Correlation Assisted Strong Uncorrelating Transform Complex Common Spatial Patterns for Spatially Distant Channel Data. Computational Intelligence and Neuroscience No. 2018 (2018), pp.1-9.
https://search.emarefa.net/detail/BIM-1130717
American Medical Association (AMA)
Kim, Youngjoo& Ryu, Jiwoo& Lee, Heejun& Lee, Seung Min& Park, Cheolsoo. Correlation Assisted Strong Uncorrelating Transform Complex Common Spatial Patterns for Spatially Distant Channel Data. Computational Intelligence and Neuroscience. 2018. Vol. 2018, no. 2018, pp.1-9.
https://search.emarefa.net/detail/BIM-1130717
Data Type
Journal Articles
Language
English
Notes
Includes bibliographical references
Record ID
BIM-1130717